利用PCA建立点云局部坐标系的c++ 代码实现
时间: 2023-10-04 15:10:36 浏览: 244
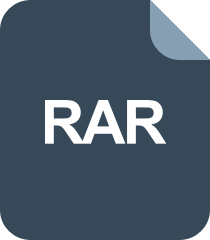
pca_normal_normal_点云_pca_点云主成分分析_法向量计算_

以下是基于 PCL(Point Cloud Library)库的 C++ 代码示例,使用 PCA 方法建立点云的局部坐标系:
```cpp
#include <iostream>
#include <pcl/point_types.h>
#include <pcl/features/normal_3d.h>
#include <pcl/filters/extract_indices.h>
#include <pcl/filters/voxel_grid.h>
#include <pcl/segmentation/sac_segmentation.h>
#include <pcl/common/transforms.h>
#include <pcl/visualization/pcl_visualizer.h>
int main(int argc, char** argv)
{
// Load point cloud data
pcl::PointCloud<pcl::PointXYZ>::Ptr cloud(new pcl::PointCloud<pcl::PointXYZ>);
pcl::io::loadPCDFile<pcl::PointXYZ>("input_cloud.pcd", *cloud);
// Apply voxel grid filtering to downsample the point cloud
pcl::VoxelGrid<pcl::PointXYZ> voxel_grid;
voxel_grid.setInputCloud(cloud);
voxel_grid.setLeafSize(0.01f, 0.01f, 0.01f);
pcl::PointCloud<pcl::PointXYZ>::Ptr cloud_downsampled(new pcl::PointCloud<pcl::PointXYZ>);
voxel_grid.filter(*cloud_downsampled);
// Estimate surface normals of the downsampled point cloud
pcl::NormalEstimation<pcl::PointXYZ, pcl::Normal> normal_estimation;
normal_estimation.setInputCloud(cloud_downsampled);
pcl::search::KdTree<pcl::PointXYZ>::Ptr kd_tree(new pcl::search::KdTree<pcl::PointXYZ>);
normal_estimation.setSearchMethod(kd_tree);
pcl::PointCloud<pcl::Normal>::Ptr cloud_normals(new pcl::PointCloud<pcl::Normal>);
normal_estimation.setRadiusSearch(0.03f);
normal_estimation.compute(*cloud_normals);
// Extract the dominant plane from the point cloud
pcl::SACSegmentationFromNormals<pcl::PointXYZ, pcl::Normal> sac_segmentation;
sac_segmentation.setOptimizeCoefficients(true);
sac_segmentation.setModelType(pcl::SACMODEL_NORMAL_PLANE);
sac_segmentation.setMethodType(pcl::SAC_RANSAC);
sac_segmentation.setMaxIterations(1000);
sac_segmentation.setDistanceThreshold(0.01);
sac_segmentation.setInputCloud(cloud_downsampled);
sac_segmentation.setInputNormals(cloud_normals);
pcl::ModelCoefficients::Ptr coefficients(new pcl::ModelCoefficients);
pcl::PointIndices::Ptr inliers(new pcl::PointIndices);
sac_segmentation.segment(*inliers, *coefficients);
// Extract the points of the dominant plane
pcl::ExtractIndices<pcl::PointXYZ> extract_indices;
extract_indices.setInputCloud(cloud_downsampled);
extract_indices.setIndices(inliers);
extract_indices.setNegative(false);
pcl::PointCloud<pcl::PointXYZ>::Ptr plane_points(new pcl::PointCloud<pcl::PointXYZ>);
extract_indices.filter(*plane_points);
// Compute the centroid of the plane points
Eigen::Vector4f centroid;
pcl::compute3DCentroid(*plane_points, centroid);
// Compute the covariance matrix of the plane points
Eigen::Matrix3f covariance_matrix;
pcl::computeCovarianceMatrixNormalized(*plane_points, centroid, covariance_matrix);
// Compute the eigenvectors and eigenvalues of the covariance matrix
Eigen::SelfAdjointEigenSolver<Eigen::Matrix3f> eigen_solver(covariance_matrix, Eigen::ComputeEigenvectors);
Eigen::Matrix3f eigenvectors = eigen_solver.eigenvectors();
Eigen::Vector3f eigenvalues = eigen_solver.eigenvalues();
// Compute the rotation matrix to align the eigenvectors with the x, y, and z axes
Eigen::Matrix4f transform_matrix = Eigen::Matrix4f::Identity();
Eigen::Matrix3f rotation_matrix = eigenvectors.transpose();
transform_matrix.block<3, 3>(0, 0) = rotation_matrix;
// Transform the point cloud to align with the x, y, and z axes
pcl::PointCloud<pcl::PointXYZ>::Ptr transformed_cloud(new pcl::PointCloud<pcl::PointXYZ>);
pcl::transformPointCloud(*cloud_downsampled, *transformed_cloud, transform_matrix);
// Visualize the original and transformed point clouds
pcl::visualization::PCLVisualizer viewer("Point Cloud Viewer");
viewer.setBackgroundColor(0.0, 0.0, 0.0);
pcl::visualization::PointCloudColorHandlerCustom<pcl::PointXYZ> original_color_handler(cloud_downsampled, 255, 255, 255);
pcl::visualization::PointCloudColorHandlerCustom<pcl::PointXYZ> transformed_color_handler(transformed_cloud, 255, 0, 0);
viewer.addPointCloud(cloud_downsampled, original_color_handler, "original_cloud");
viewer.addPointCloud(transformed_cloud, transformed_color_handler, "transformed_cloud");
viewer.setPointCloudRenderingProperties(pcl::visualization::PCL_VISUALIZER_POINT_SIZE, 1, "original_cloud");
viewer.setPointCloudRenderingProperties(pcl::visualization::PCL_VISUALIZER_POINT_SIZE, 1, "transformed_cloud");
viewer.addCoordinateSystem(0.1);
viewer.initCameraParameters();
while (!viewer.wasStopped())
{
viewer.spinOnce();
}
return 0;
}
```
这个代码示例中使用了 PCL 库中的一些模块,包括:
- `pcl::io::loadPCDFile()`:用于加载 PCD 文件中的点云数据。
- `pcl::VoxelGrid`:用于对点云进行下采样。
- `pcl::NormalEstimation`:用于估计点云的法向量。
- `pcl::SACSegmentationFromNormals`:用于从点云中提取主平面。
- `pcl::ExtractIndices`:用于从点云中提取指定索引的点。
- `pcl::compute3DCentroid`:用于计算点云的质心。
- `pcl::computeCovarianceMatrixNormalized`:用于计算点云的协方差矩阵。
- `Eigen::SelfAdjointEigenSolver`:用于计算协方差矩阵的特征向量和特征值。
- `pcl::transformPointCloud`:用于将点云进行旋转和平移操作。
- `pcl::visualization::PCLVisualizer`:用于可视化点云和坐标系。
具体实现过程可以参考代码中的注释。需要注意的是,这个代码示例只是一个简单的实现,实际应用需要根据具体情况进行修改和优化。
阅读全文
相关推荐
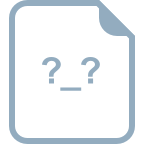
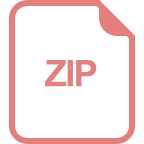















