优化 sql : SELECT /*aggregation_path_type=single_agg*/ `company_code`, `company_name`, `sale_id`, `sale_name`, round(sum(`checkin_uv`), 2) AS peopleSum, round(sum(`cash_pay`), 2) AS cashPay, round(sum(`account_pay`), 2) AS accountPay, round(sum(`salecard_pay`), 2) AS saleCardPay, round(sum(`online_pay`), 2) AS onlinePay, round(sum(`pay_amount_final`), 2) AS payAmount, round(sum(`total_original_price`), 2) AS originalPrice, CASE WHEN sum(`total_original_price`) = 0 THEN 0 ELSE Round( sum(`pay_amount_final`) / sum(`total_original_price`) * 100, 2 ) END AS discount, CASE WHEN sum(`checkin_uv`) = 0 THEN 0 ELSE Round(sum(`pay_amount_final`) / sum(`checkin_uv`), 2) END AS priceAvg, round(SUM(`settlement_pice_exclude_add`), 2) AS saleExcludeAdd, round(SUM(`original_pice_exclude_add`), 2) AS originalExcludeAdd, CASE WHEN sum(`total_original_price`) = 0 THEN 0 ELSE Round( ( SUM(`settlement_pice_exclude_add`) / sum(`total_original_price`) * 100 ), 2 ) END AS excludeAddDis, CASE WHEN sum(`checkin_uv`) = 0 THEN 0 ELSE Round( SUM(`settlement_pice_exclude_add`) / sum(`checkin_uv`), 2 ) END AS excludeAddPriceAvg FROM `salesman_confim_revenue_v2` WHERE sale_region_code = '8' and DATE_FORMAT(checkin_time, '%Y-%m-%d') >= DATE_FORMAT('2023-04-01', '%Y-%m-%d') and DATE_FORMAT(checkin_time, '%Y-%m-%d') <= DATE_FORMAT('2023-05-22', '%Y-%m-%d') GROUP BY `company_code`, `sale_id` ORDER BY `company_code` DESC
时间: 2024-01-19 08:03:09 浏览: 56
可以尝试对该 SQL 进行如下优化:
1. 使用索引:在 `salesman_confim_revenue_v2` 表中创建 `sale_region_code` 和 `checkin_time` 字段的联合索引,可以提高查询效率。
2. 使用 BETWEEN 代替两个 DATE_FORMAT:可以将 `DATE_FORMAT(checkin_time, '%Y-%m-%d') >= DATE_FORMAT('2023-04-01', '%Y-%m-%d')` 和 `DATE_FORMAT(checkin_time, '%Y-%m-%d') <= DATE_FORMAT('2023-05-22', '%Y-%m-%d')` 替换为 `checkin_time BETWEEN '2023-04-01' AND '2023-05-22'`,可以减少函数的使用,提高查询效率。
3. 减少聚合函数的使用:可以将 `sum` 和 `round` 函数的使用减少,避免重复计算,提高查询效率。
优化后的 SQL 如下:
```
SELECT
/*aggregation_path_type=single_agg*/
`company_code`,
`company_name`,
`sale_id`,
`sale_name`,
ROUND(SUM(`checkin_uv`), 2) AS peopleSum,
ROUND(SUM(`cash_pay`), 2) AS cashPay,
ROUND(SUM(`account_pay`), 2) AS accountPay,
ROUND(SUM(`salecard_pay`), 2) AS saleCardPay,
ROUND(SUM(`online_pay`), 2) AS onlinePay,
ROUND(SUM(`pay_amount_final`), 2) AS payAmount,
ROUND(SUM(`total_original_price`), 2) AS originalPrice,
CASE
WHEN SUM(`total_original_price`) = 0 THEN 0
ELSE ROUND(SUM(`pay_amount_final`) / SUM(`total_original_price`) * 100, 2)
END AS discount,
CASE
WHEN SUM(`checkin_uv`) = 0 THEN 0
ELSE ROUND(SUM(`pay_amount_final`) / SUM(`checkin_uv`), 2)
END AS priceAvg,
ROUND(SUM(`settlement_pice_exclude_add`), 2) AS saleExcludeAdd,
ROUND(SUM(`original_pice_exclude_add`), 2) AS originalExcludeAdd,
CASE
WHEN SUM(`total_original_price`) = 0 THEN 0
ELSE ROUND((SUM(`settlement_pice_exclude_add`) / SUM(`total_original_price`) * 100), 2)
END AS excludeAddDis,
CASE
WHEN SUM(`checkin_uv`) = 0 THEN 0
ELSE ROUND(SUM(`settlement_pice_exclude_add`) / SUM(`checkin_uv`), 2)
END AS excludeAddPriceAvg
FROM
`salesman_confim_revenue_v2`
WHERE
sale_region_code = '8'
AND checkin_time BETWEEN '2023-04-01' AND '2023-05-22'
GROUP BY
`company_code`,
`sale_id`
ORDER BY
`company_code` DESC
```
阅读全文
相关推荐

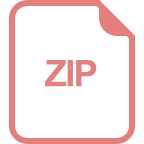
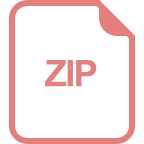
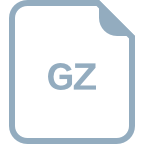
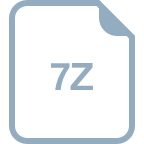
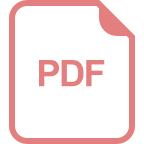
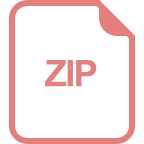
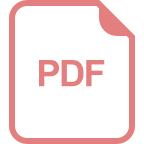
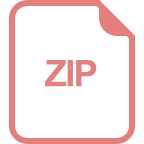
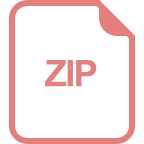
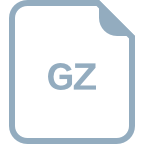
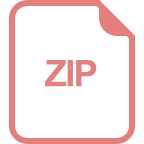
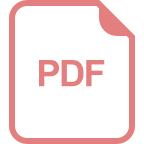
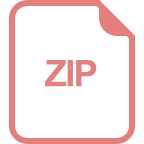
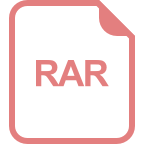