多分类focalloss pytorch代码,详解
时间: 2024-04-30 14:24:06 浏览: 27
Focal Loss是一种用于处理类别不平衡问题的损失函数。在训练深度学习模型时,由于数据集中不同类别的样本数量往往存在较大的差异,因此训练出的模型容易出现对数量较大的类别表现良好,对数量较小的类别表现较差的情况。Focal Loss通过调整样本的权重,使得模型更加关注难以分类的样本,从而提高模型在数量较小的类别上的性能。
下面是使用PyTorch实现多分类Focal Loss的代码:
```
import torch
import torch.nn as nn
import torch.nn.functional as F
class FocalLoss(nn.Module):
def __init__(self, alpha=1, gamma=2, reduction='mean'):
super(FocalLoss, self).__init__()
self.alpha = alpha
self.gamma = gamma
self.reduction = reduction
def forward(self, inputs, targets):
ce_loss = F.cross_entropy(inputs, targets, reduction='none')
pt = torch.exp(-ce_loss)
focal_loss = self.alpha * (1 - pt) ** self.gamma * ce_loss
if self.reduction == 'mean':
return torch.mean(focal_loss)
elif self.reduction == 'sum':
return torch.sum(focal_loss)
else:
return focal_loss
```
在这里,我们定义了一个名为FocalLoss的自定义损失函数,并在其构造函数中定义了三个参数。alpha参数用于平衡每个类别的权重,gamma参数用于调整样本难度的权重,reduction参数用于指定损失函数的计算方式(mean或sum)。
在forward函数中,我们首先计算普通的交叉熵损失(ce_loss),然后计算每个样本的难度系数(pt),最后计算Focal Loss(focal_loss)。最后根据reduction参数的设定,返回损失函数的值。
在使用Focal Loss时,我们需要在训练过程中将损失函数替换为Focal Loss即可。例如,如果我们使用了PyTorch的nn.CrossEntropyLoss作为损失函数,我们可以将其替换为FocalLoss:
```
criterion = FocalLoss(alpha=1, gamma=2)
```
这样,在训练过程中就会使用Focal Loss作为损失函数,从而提高模型在数量较小的类别上的性能。
相关推荐
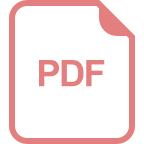
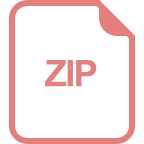














