import numpy as np import cv2 font= cv2.FONT_HERSHEY_SIMPLEX lower_red=np.array([0,127,128])#红色阈值下界 higher_red=np.array([10,255,255])#红色阈值上界 lower_green=np.array([35,110,106])#绿色阈值下界 higher_green=np.array([77,255,255])#绿色阈值上界 cap=cv2.VideoCapture(0)#打开电脑内置摄像头 if(cap.isOpened()): while(True): ret,frame=cap.read()#按帧读取,这是读取一帧 img_hsv=cv2.cvtColor(frame,cv2.COLOR_BGR2HSV) mask_red=cv2.inRange(img_hsv,lower_red,higher_red)#可以认为是过滤出红色部分,获得红色的掩膜 mask_green=cv2.inRange(img_hsv,lower_green,higher_green)#获得绿色部分掩膜 mask_green = cv2.medianBlur(mask_green, 7) # 中值滤波 mask_red = cv2.medianBlur(mask_red, 7) # 中值滤波 mask=cv2.bitwise_or(mask_green,mask_red)#三部分掩膜进行按位或运算 image1,cnts1,hierarchy1=cv2.findContours(mask_red,cv2.RETR_EXTERNAL,cv2.CHAIN_APPROX_NONE)#轮廓检测 image3,cnts3,hierarchy3=cv2.findContours(mask_green,cv2.RETR_EXTERNAL,cv2.CHAIN_APPROX_NONE) for cnt in cnts1: (x,y,w,h)=cv2.boundingRect(cnt)#该函数返回矩阵四个点 cv2.rectangle(frame, (x, y), (x + w, y + h), (0, 0, 255), 2)#将检测到的颜色框起来 cv2.putText(frame,'red',(x,y-5),font,0.7,(0,0,255),2) for cnt in cnts3: (x, y, w, h) = cv2.boundingRect(cnt) # 该函数返回矩阵四个点 cv2.rectangle(frame, (x, y), (x + w, y + h), (0, 255, 0), 2) # 将检测到的颜色框起来 cv2.putText(frame, 'green', (x, y - 5), font, 0.7, (0,255,0), 2) cv2.imshow('frame',frame) k=cv2.waitKey(20)&0xFF if k ==27: break cv2.waitKey(0) cv2.destroyAllWindows()
时间: 2023-10-10 08:09:36 浏览: 53
这段代码是用来通过电脑内置摄像头实时检测红色和绿色物体,并在画面上用矩形框标记出来。首先,代码导入了必要的库,然后定义了红色和绿色的阈值范围。接下来,打开摄像头并进行帧读取,将读取到的帧转换为HSV颜色空间。然后使用阈值范围过滤红色和绿色部分,并进行中值滤波处理。最后,使用轮廓检测找到物体的轮廓,并在画面上用矩形框标记出来。
请问有什么问题我可以帮助你解答吗?
相关问题
import numpy as np import cv2 font= cv2.FONT_HERSHEY_SIMPLEX lower_red=np.array([0,127,128])#红色阈值下界 higher_red=np.array([10,255,255])#红色阈值上界 lower_green=np.array([35,110,106])#绿色阈值下界 higher_green=np.array([77,255,255])#绿色阈值上界 cap=cv2.VideoCapture(0)#打开电脑内置摄像头 cv2.namedWindow("Display_Image", cv2.WINDOW_NORMAL) if(cap.isOpened()): while(True): ret,frame=cap.read()#按帧读取,这是读取一帧 img_hsv=cv2.cvtColor(frame,cv2.COLOR_BGR2HSV) mask_red=cv2.inRange(img_hsv,lower_red,higher_red)#可以认为是过滤出红色部分,获得红色的掩膜 mask_green=cv2.inRange(img_hsv,lower_green,higher_green)#获得绿色部分掩膜 mask_green = cv2.medianBlur(mask_green, 7) # 中值滤波 mask_red = cv2.medianBlur(mask_red, 7) # 中值滤波 mask=cv2.bitwise_or(mask_green,mask_red)#三部分掩膜进行按位或运算 cnts1, hierarchy1 = cv2.findContours(mask_red, cv2.RETR_EXTERNAL, cv2.CHAIN_APPROX_NONE) cnts3, hierarchy3 = cv2.findContours(mask_green, cv2.RETR_EXTERNAL, cv2.CHAIN_APPROX_NONE) for cnt in cnts1: (x,y,w,h)=cv2.boundingRect(cnt)#该函数返回矩阵四个点 cv2.rectangle(frame, (x, y), (x + w, y + h), (0, 0, 255), 2)#将检测到的颜色框起来 cv2.putText(frame,'red',(x,y-5),font,0.7,(0,0,255),2) for cnt in cnts3: (x, y, w, h) = cv2.boundingRect(cnt) # 该函数返回矩阵四个点 cv2.rectangle(frame, (x, y), (x + w, y + h), (0, 255, 0), 2) # 将检测到的颜色框起来 cv2.putText(frame, 'green', (x, y - 5), font, 0.7, (0,255,0), 2) cv2.imshow('frame',frame) k=cv2.waitKey(20)&0xFF if k ==27: break cv2.waitKey(0) cv2.destroyAllWindows()这段代码为什么打不电脑自带的摄像头
这段代码可能无法打开电脑自带的摄像头的原因有几种可能性:
1. 摄像头权限问题:请确保你的代码运行在有摄像头访问权限的用户下。
2. 摄像头驱动问题:有些摄像头需要特定的驱动程序才能正常工作。请检查你的摄像头是否需要安装相应的驱动程序。
3. 摄像头设备号错误:在`cap=cv2.VideoCapture(0)`这一行中,参数`0`表示默认使用设备号为0的摄像头。如果你的电脑有多个摄像头,可能需要更改该参数来指定正确的摄像头设备号。
如果上述解决方法都无效,可以尝试使用其他相机软件(如Zoom、Skype等)来确认摄像头是否正常工作,并检查是否需要更新摄像头驱动程序。
使用python,利用模板匹配的方法,找到摄像头中模板位置,进行标记,输出范围和坐标,并对模板颜色进行区分,判断其为红色、绿色或蓝色
好的,这是一个关于计算机视觉的问题,需要使用OpenCV库来完成。首先需要导入相关库:
```python
import cv2
import numpy as np
```
接下来,我们需要读取摄像头的实时视频流:
```python
cap = cv2.VideoCapture(0)
```
然后,我们需要读取模板图片并进行颜色分离:
```python
template = cv2.imread('template.jpg')
hsv_template = cv2.cvtColor(template, cv2.COLOR_BGR2HSV)
# 红色
lower_red = np.array([0, 50, 50])
upper_red = np.array([10, 255, 255])
mask_red1 = cv2.inRange(hsv_template, lower_red, upper_red)
lower_red = np.array([170, 50, 50])
upper_red = np.array([180, 255, 255])
mask_red2 = cv2.inRange(hsv_template, lower_red, upper_red)
mask_red = mask_red1 + mask_red2
# 绿色
lower_green = np.array([35, 43, 46])
upper_green = np.array([90, 255, 255])
mask_green = cv2.inRange(hsv_template, lower_green, upper_green)
# 蓝色
lower_blue = np.array([100, 43, 46])
upper_blue = np.array([124, 255, 255])
mask_blue = cv2.inRange(hsv_template, lower_blue, upper_blue)
```
然后,我们需要不断读取视频帧并进行模板匹配,找到匹配的位置:
```python
while True:
ret, frame = cap.read()
if not ret:
break
hsv_frame = cv2.cvtColor(frame, cv2.COLOR_BGR2HSV)
res_red = cv2.matchTemplate(hsv_frame, mask_red, cv2.TM_CCOEFF_NORMED)
res_green = cv2.matchTemplate(hsv_frame, mask_green, cv2.TM_CCOEFF_NORMED)
res_blue = cv2.matchTemplate(hsv_frame, mask_blue, cv2.TM_CCOEFF_NORMED)
threshold = 0.8
loc_red = np.where(res_red >= threshold)
loc_green = np.where(res_green >= threshold)
loc_blue = np.where(res_blue >= threshold)
for pt in zip(*loc_red[::-1]):
cv2.rectangle(frame, pt, (pt[0] + template.shape[1], pt[1] + template.shape[0]), (0, 0, 255), 2)
cv2.putText(frame, 'Red', pt, cv2.FONT_HERSHEY_SIMPLEX, 1, (0, 0, 255), 2)
for pt in zip(*loc_green[::-1]):
cv2.rectangle(frame, pt, (pt[0] + template.shape[1], pt[1] + template.shape[0]), (0, 255, 0), 2)
cv2.putText(frame, 'Green', pt, cv2.FONT_HERSHEY_SIMPLEX, 1, (0, 255, 0), 2)
for pt in zip(*loc_blue[::-1]):
cv2.rectangle(frame, pt, (pt[0] + template.shape[1], pt[1] + template.shape[0]), (255, 0, 0), 2)
cv2.putText(frame, 'Blue', pt, cv2.FONT_HERSHEY_SIMPLEX, 1, (255, 0, 0), 2)
cv2.imshow('frame', frame)
if cv2.waitKey(1) == ord('q'):
break
cap.release()
cv2.destroyAllWindows()
```
在这段代码中,我们使用了 `cv2.matchTemplate()` 函数来进行模板匹配,并使用 `cv2.rectangle()` 和 `cv2.putText()` 函数来在匹配到的位置上画出矩形框和标识文字。同时,我们还使用了颜色编码来区分不同的模板颜色。
注意,这里的阈值 `threshold` 需要根据实际情况进行调整,以确保正确匹配到模板。
相关推荐
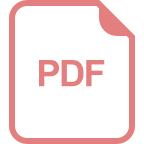
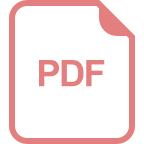











