def Draw_Classification_Map(label, name: str, scale: float = 4.0, dpi: int = 400): ''' get classification map , then save to given path :param label: classification label, 2D :param name: saving path and file's name :param scale: scale of image. If equals to 1, then saving-size is just the label-size :param dpi: default is OK :return: null ''' fig, ax = plt.subplots() numlabel = np.array(label) v = spy.imshow(classes=numlabel.astype(np.int16), fignum=fig.number) ax.set_axis_off() ax.xaxis.set_visible(False) ax.yaxis.set_visible(False) fig.set_size_inches(label.shape[1] * scale / dpi, label.shape[0] * scale / dpi) foo_fig = plt.gcf() # 'get current figure' plt.gca().xaxis.set_major_locator(plt.NullLocator()) plt.gca().yaxis.set_major_locator(plt.NullLocator()) plt.subplots_adjust(top=1, bottom=0, right=1, left=0, hspace=0, wspace=0) foo_fig.savefig(name + '.png', format='png', transparent=True, dpi=dpi, pad_inches=0) pass怎么才能把这段代码输出的超像素级图片转换为像素级图片
时间: 2024-01-06 20:05:55 浏览: 41
要将超像素级别的图片转换为像素级别的图片,需要进行图像分割的反过程。一种简单的方法是,对于每个超像素,使用超像素中心点的值来填充整个超像素区域。可以使用 OpenCV 中的函数 `watershed` 对图像进行分割,然后对于每个超像素,找到其对应的标签,并使用该标签中心点的像素值填充整个超像素区域。以下是可能实现该方法的代码示例:
```python
import cv2
import numpy as np
# 读取超像素级别的图像
superpixel_image = cv2.imread('superpixel_image.png')
# 读取超像素标签图像
superpixel_labels = cv2.imread('superpixel_labels.png', cv2.IMREAD_GRAYSCALE)
# 对超像素标签图像进行分水岭分割
markers = cv2.watershed(superpixel_image, superpixel_labels)
# 对于每个超像素,找到其对应的标签,并使用该标签中心点的像素值填充整个超像素区域
image = np.zeros_like(superpixel_image)
for label in np.unique(markers):
if label == -1:
continue
mask = np.zeros_like(superpixel_labels)
mask[markers == label] = 255
_, contours, _ = cv2.findContours(mask, cv2.RETR_EXTERNAL, cv2.CHAIN_APPROX_SIMPLE)
if len(contours) == 0:
continue
center = np.mean(contours[0], axis=0, dtype=np.int32)
pixel_value = superpixel_image[center[0], center[1]]
image[markers == label] = pixel_value
# 保存像素级别的图像
cv2.imwrite('pixel_image.png', image)
```
请注意,以上代码示例仅供参考,具体实现可能会因图像分割算法和超像素算法的不同而有所差异。
相关推荐
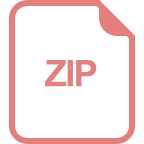
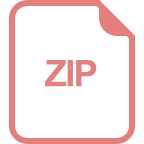
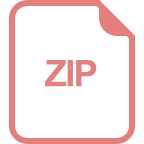















