ai算法java代码示例
时间: 2023-07-28 10:07:59 浏览: 47
以下是一个简单的基于Java的决策树算法示例:
```
import java.util.ArrayList;
public class DecisionTree {
private TreeNode root;
public DecisionTree() {
root = null;
}
public void train(ArrayList<DataPoint> data) {
root = buildTree(data);
}
public String predict(DataPoint data) {
return predictHelper(root, data);
}
private TreeNode buildTree(ArrayList<DataPoint> data) {
if (data.isEmpty()) {
return new TreeNode("unknown");
}
// 选择最优的特征作为分裂点
Feature bestFeature = getBestFeature(data);
TreeNode node = new TreeNode(bestFeature.getName());
// 递归构建子树
ArrayList<String> featureValues = bestFeature.getValues();
for (String value : featureValues) {
ArrayList<DataPoint> subset = getSubset(data, bestFeature, value);
TreeNode child = buildTree(subset);
node.addChild(value, child);
}
return node;
}
private String predictHelper(TreeNode node, DataPoint data) {
if (node.isLeaf()) {
return node.getLabel();
}
String featureValue = data.getFeature(node.getName());
TreeNode child = node.getChild(featureValue);
return predictHelper(child, data);
}
private Feature getBestFeature(ArrayList<DataPoint> data) {
// 计算信息增益,选择信息增益最大的特征
double entropy = getEntropy(data);
Feature bestFeature = null;
double bestGain = 0;
for (Feature feature : data.get(0).getFeatures()) {
double gain = entropy - getConditionalEntropy(data, feature);
if (gain > bestGain) {
bestGain = gain;
bestFeature = feature;
}
}
return bestFeature;
}
private double getEntropy(ArrayList<DataPoint> data) {
// 计算数据集的熵
int size = data.size();
int[] counts = new int[2];
for (DataPoint point : data) {
counts[point.getLabel()]++;
}
double entropy = 0;
for (int count : counts) {
if (count > 0) {
double p = (double) count / size;
entropy -= p * Math.log(p) / Math.log(2);
}
}
return entropy;
}
private double getConditionalEntropy(ArrayList<DataPoint> data, Feature feature) {
// 计算特征条件下的数据集的熵
double conditionalEntropy = 0;
ArrayList<String> featureValues = feature.getValues();
for (String value : featureValues) {
ArrayList<DataPoint> subset = getSubset(data, feature, value);
double p = (double) subset.size() / data.size();
conditionalEntropy += p * getEntropy(subset);
}
return conditionalEntropy;
}
private ArrayList<DataPoint> getSubset(ArrayList<DataPoint> data, Feature feature, String value) {
// 获取某个特征取某个值的数据子集
ArrayList<DataPoint> subset = new ArrayList<DataPoint>();
for (DataPoint point : data) {
if (point.getFeature(feature.getName()).equals(value)) {
subset.add(point);
}
}
return subset;
}
}
class TreeNode {
private String name;
private String label;
private ArrayList<TreeNode> children;
private ArrayList<String> values;
public TreeNode(String name) {
this.name = name;
label = null;
children = new ArrayList<TreeNode>();
values = new ArrayList<String>();
}
public void addChild(String value, TreeNode child) {
children.add(child);
values.add(value);
}
public String getName() {
return name;
}
public boolean isLeaf() {
return label != null;
}
public void setLabel(String label) {
this.label = label;
}
public String getLabel() {
return label;
}
public TreeNode getChild(String value) {
int index = values.indexOf(value);
return children.get(index);
}
}
class DataPoint {
private ArrayList<Feature> features;
private int label;
public DataPoint(ArrayList<Feature> features, int label) {
this.features = features;
this.label = label;
}
public String getFeature(String name) {
for (Feature feature : features) {
if (feature.getName().equals(name)) {
return feature.getValue();
}
}
return null;
}
public ArrayList<Feature> getFeatures() {
return features;
}
public int getLabel() {
return label;
}
}
class Feature {
private String name;
private String value;
private ArrayList<String> values;
public Feature(String name, String value) {
this.name = name;
this.value = value;
values = new ArrayList<String>();
values.add(value);
}
public Feature(String name, ArrayList<String> values) {
this.name = name;
this.values = values;
value = null;
}
public String getName() {
return name;
}
public String getValue() {
return value;
}
public ArrayList<String> getValues() {
return values;
}
}
```
这段代码实现了一个简单的决策树算法,包括训练和预测功能。它使用了三个类:`DecisionTree`表示决策树,`TreeNode`表示树节点,`DataPoint`表示数据点。其中,`DecisionTree`类中的`train`方法用于训练决策树,`predict`方法用于预测新的数据点。这段代码只是一个简单的示例,实际应用中可能需要进行更多的优化和改进。
相关推荐
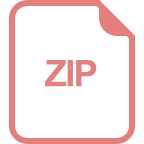














