根据梯度下降法解析解,编写线性回归算法,,数据集:http://archive.ics.uci.edu/ml/datasets/Abalone
时间: 2023-10-06 21:10:25 浏览: 39
由于数据集中的目标变量不是连续的数值,而是离散的类别,因此我们需要对目标变量进行预处理。在这里,我们将把目标变量“Rings”转换为一个连续的数值变量,并根据转换后的变量进行线性回归。
首先,我们需要加载数据,并将目标变量转换为连续的数值变量。
```python
import pandas as pd
# 加载数据
url = "http://archive.ics.uci.edu/ml/machine-learning-databases/abalone/abalone.data"
names = ["Sex", "Length", "Diameter", "Height", "Whole weight", "Shucked weight", "Viscera weight", "Shell weight", "Rings"]
data = pd.read_csv(url, header=None, names=names)
# 将目标变量转换为连续的数值变量
data["Rings"] = data["Rings"] - 1.5
```
然后,我们需要将特征变量和目标变量分离,并将它们转换为NumPy数组。
```python
import numpy as np
# 将特征变量和目标变量分离
X = data.iloc[:, :-1].values
y = data.iloc[:, -1].values
# 将目标变量转换为列向量
y = y.reshape(-1, 1)
```
接下来,我们需要对特征变量进行标准化处理,以便在梯度下降过程中更好地收敛。
```python
from sklearn.preprocessing import StandardScaler
# 对特征变量进行标准化处理
sc_X = StandardScaler()
X = sc_X.fit_transform(X)
```
现在,我们可以开始实现线性回归算法。在这里,我们将使用梯度下降法来计算回归系数。
```python
class LinearRegression:
def __init__(self, alpha=0.01, num_iterations=1000):
self.alpha = alpha
self.num_iterations = num_iterations
def fit(self, X, y):
# 添加截距项
X = np.hstack((np.ones((X.shape[0], 1)), X))
# 初始化回归系数
self.theta = np.zeros((X.shape[1], 1))
# 计算梯度下降
for i in range(self.num_iterations):
h = np.dot(X, self.theta)
error = h - y
gradient = np.dot(X.T, error) / y.size
self.theta -= self.alpha * gradient
def predict(self, X):
# 添加截距项
X = np.hstack((np.ones((X.shape[0], 1)), X))
# 预测目标变量
y_pred = np.dot(X, self.theta)
return y_pred
```
现在,我们可以使用我们的线性回归算法来拟合数据集,并计算预测误差。
```python
# 拟合数据集
lr = LinearRegression(alpha=0.01, num_iterations=1000)
lr.fit(X, y)
# 计算预测误差
y_pred = lr.predict(X)
mse = np.mean((y_pred - y) ** 2)
print("预测误差:", mse)
```
最后,我们可以使用matplotlib库将预测目标变量与实际目标变量进行可视化比较。
```python
import matplotlib.pyplot as plt
# 可视化比较
plt.figure(figsize=(10, 6))
plt.scatter(y, y_pred)
plt.plot([y.min(), y.max()], [y.min(), y.max()], "k--", lw=3)
plt.xlabel("实际目标变量")
plt.ylabel("预测目标变量")
plt.show()
```
相关推荐
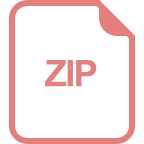
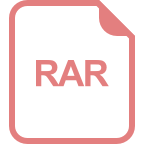














