使用pcl识别点云平面
时间: 2023-09-22 22:07:02 浏览: 108
点云平面识别是PCL库中的一个常见应用,可以通过以下步骤实现:
1. 读入点云数据,可以使用PCL的PointCloud类来处理点云数据。
2. 估计点云的法向量,可以使用PCL中的NormalEstimation类来估计点云的法向量。
3. 对点云进行分割,将点云分割成不同的平面,可以使用PCL中的SACSegmentation类来进行分割。
4. 对每个平面进行聚类,将点云分成不同的聚类,可以使用PCL中的EuclideanClusterExtraction类进行聚类。
5. 可以将每个聚类绘制出来,以便可视化结果。
下面是一个简单的示例代码,展示如何使用PCL库识别点云平面:
```
#include <pcl/point_types.h>
#include <pcl/features/normal_3d.h>
#include <pcl/segmentation/sac_segmentation.h>
#include <pcl/filters/extract_indices.h>
#include <pcl/kdtree/kdtree.h>
#include <pcl/segmentation/extract_clusters.h>
int main ()
{
// 加载点云数据
pcl::PointCloud<pcl::PointXYZ>::Ptr cloud (new pcl::PointCloud<pcl::PointXYZ>);
pcl::PointCloud<pcl::PointXYZ>::Ptr cloud_filtered (new pcl::PointCloud<pcl::PointXYZ>);
pcl::io::loadPCDFile<pcl::PointXYZ> ("table_scene_lms400.pcd", *cloud);
// 估计法向量
pcl::NormalEstimation<pcl::PointXYZ, pcl::Normal> ne;
pcl::PointCloud<pcl::Normal>::Ptr normals (new pcl::PointCloud<pcl::Normal>);
pcl::search::KdTree<pcl::PointXYZ>::Ptr tree (new pcl::search::KdTree<pcl::PointXYZ>);
tree->setInputCloud (cloud);
ne.setInputCloud (cloud);
ne.setSearchMethod (tree);
ne.setRadiusSearch (0.03);
ne.compute (*normals);
// 分割平面
pcl::SACSegmentation<pcl::PointXYZ> seg;
pcl::ModelCoefficients::Ptr coefficients (new pcl::ModelCoefficients);
pcl::PointIndices::Ptr inliers (new pcl::PointIndices);
seg.setOptimizeCoefficients (true);
seg.setModelType (pcl::SACMODEL_PLANE);
seg.setMethodType (pcl::SAC_RANSAC);
seg.setDistanceThreshold (0.01);
seg.setInputCloud (cloud);
seg.setInputNormals (normals);
seg.segment (*inliers, *coefficients);
// 提取平面点云
pcl::ExtractIndices<pcl::PointXYZ> extract;
extract.setInputCloud (cloud);
extract.setIndices (inliers);
extract.filter (*cloud_filtered);
// 聚类
pcl::search::KdTree<pcl::PointXYZ>::Ptr ec_tree (new pcl::search::KdTree<pcl::PointXYZ>);
ec_tree->setInputCloud (cloud_filtered);
std::vector<pcl::PointIndices> cluster_indices;
pcl::EuclideanClusterExtraction<pcl::PointXYZ> ec;
ec.setClusterTolerance (0.02);
ec.setMinClusterSize (100);
ec.setMaxClusterSize (25000);
ec.setSearchMethod (ec_tree);
ec.setInputCloud (cloud_filtered);
ec.extract (cluster_indices);
// 输出结果
std::cout << "Number of clusters: " << cluster_indices.size () << std::endl;
for (std::vector<pcl::PointIndices>::const_iterator it = cluster_indices.begin (); it != cluster_indices.end (); ++it)
{
pcl::PointCloud<pcl::PointXYZ>::Ptr cloud_cluster (new pcl::PointCloud<pcl::PointXYZ>);
for (std::vector<int>::const_iterator pit = it->indices.begin (); pit != it->indices.end (); ++pit)
cloud_cluster->push_back ((*cloud_filtered)[*pit]);
std::cout << "Cluster size: " << cloud_cluster->size () << std::endl;
}
return (0);
}
```
这个示例代码首先加载点云数据,然后使用NormalEstimation类估计点云的法向量。接着,使用SACSegmentation类分割点云,提取平面点云。最后,使用EuclideanClusterExtraction类对平面点云进行聚类,并输出聚类结果。
阅读全文
相关推荐
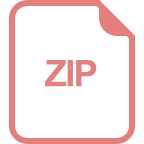
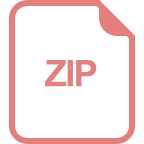
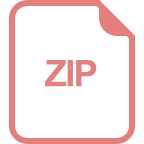















