pytorch二分类loss
时间: 2023-06-30 20:09:16 浏览: 182
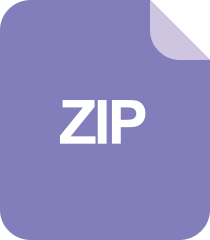
基于pytorch使用神经网络完成 鸟花 二分类问题(包含模型训练、样本数据等全套内容).zip
在 PyTorch 中,可以使用二分类交叉熵损失函数(Binary Cross Entropy Loss)来进行二分类任务的损失计算。假设模型输出为 $y_i \in [0,1]$,标签为 $t_i \in \{0,1\}$,则二分类交叉熵损失函数的公式为:
$$
\text{BCELoss}(y, t) = -\frac{1}{N}\sum_{i=1}^N [t_i\log(y_i)+(1-t_i)\log(1-y_i)]
$$
其中,$N$ 为样本数。在实际使用时,可以使用 PyTorch 中的 `BCELoss` 类来实现二分类交叉熵损失的计算。
示例代码如下:
```python
import torch.nn as nn
import torch
# 定义模型
class MyModel(nn.Module):
def __init__(self):
super(MyModel, self).__init__()
self.fc = nn.Linear(10, 1)
self.sig = nn.Sigmoid()
def forward(self, x):
x = self.fc(x)
x = self.sig(x)
return x
# 定义损失函数
criterion = nn.BCELoss()
# 定义输入和标签
x = torch.randn(2, 10)
t = torch.Tensor([1, 0]).view(2, 1)
# 计算输出
model = MyModel()
y = model(x)
# 计算损失
loss = criterion(y, t)
print(loss)
```
在上面的代码中,我们定义了一个简单的全连接神经网络模型 `MyModel`,并使用 `BCELoss` 类计算模型输出 `y` 和标签 `t` 的损失。需要注意的是,在计算损失时,`y` 的维度需要与 `t` 的维度相同。
阅读全文
相关推荐
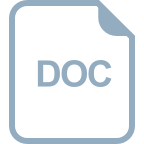
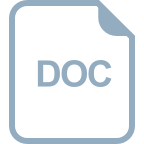
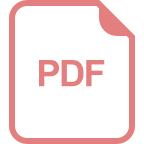
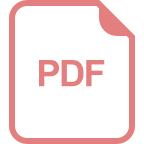
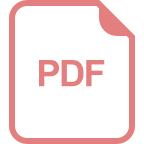












