def get_style_model_and_losses(cnn, style_img, content_img, style_weight=1000, content_weight=1, content_layers=content_layers_default, style_layers=style_layers_default): cnn = copy.deepcopy(cnn) # just in order to have an iterable access to or list of content/syle # losses content_losses = [] style_losses = [] model = nn.Sequential() # the new Sequential module network gram = GramMatrix() # we need a gram module in order to compute style targets # move these modules to the GPU if possible: if use_cuda: model = model.cuda() gram = gram.cuda()
时间: 2023-12-20 22:03:27 浏览: 34
这是一个 PyTorch 中的函数,用于获取风格迁移模型和计算内容和风格损失。具体来说,它首先复制一个传入的卷积神经网络(cnn),然后将其作为一个新的 Sequential 模块网络(model)。接下来,它定义了一个 GramMatrix 模块(用于计算风格目标),并将其移动到 GPU 上(如果可用)。最后,它返回内容和风格损失列表以及新的模型网络。其中,参数 style_weight 和 content_weight 控制了风格和内容损失的相对权重,而 content_layers 和 style_layers 则指定了计算内容和风格损失所用的网络层。
相关问题
def get_input_param_optimizer(input_img): # this line to show that input is a parameter that requires a gradient input_param = nn.Parameter(input_img.data) optimizer = optim.LBFGS([input_param]) return input_param, optimizer def run_style_transfer(cnn, content_img, style_img, input_img, num_steps=300, style_weight=1000, content_weight=1): """Run the style transfer.""" print('Building the style transfer model..') model, style_losses, content_losses = get_style_model_and_losses(cnn, style_img, content_img, style_weight, content_weight) input_param, optimizer = get_input_param_optimizer(input_img) print('Optimizing..') run = [0] while run[0] <= num_steps: def closure(): # correct the values of updated input image input_param.data.clamp_(0, 1) optimizer.zero_grad() model(input_param) style_score = 0 content_score = 0 for sl in style_losses: style_score += sl.backward() for cl in content_losses: content_score += cl.backward() run[0] += 1 if run[0] % 50 == 0: print("run {}:".format(run)) print('Style Loss : {:4f} Content Loss: {:4f}'.format( style_score.data[0], content_score.data[0])) print() return style_score + content_score optimizer.step(closure) # a last correction... input_param.data.clamp_(0, 1) return input_param.data
这是一个用于运行风格迁移的 Python 代码。它使用 PyTorch 框架来实现。具体来说,它定义了一个函数 `run_style_transfer`,该函数接受一些参数:`cnn`,`content_img`,`style_img`,`input_img`,`num_steps`,`style_weight` 和 `content_weight`。其中,`cnn` 是卷积神经网络,`content_img` 是内容图像,`style_img` 是风格图像,`input_img` 是输入图像(即风格迁移生成的图像),`num_steps` 是进行优化的迭代次数,`style_weight` 和 `content_weight` 是用于计算损失的权重参数。
在 `run_style_transfer` 函数中,首先调用了 `get_style_model_and_losses` 函数来获取风格迁移模型,并拆分出样式损失和内容损失。然后,调用 `get_input_param_optimizer` 函数来获取输入图像参数和优化器。接下来,使用 LBFGS 优化器来最小化样式损失和内容损失的加权和,以生成最终的输出图像。
在 `closure` 函数中,对输入图像进行修正,并计算样式损失和内容损失。最后,将两者相加,返回优化器的损失值。在每 50 个迭代步骤之后,输出当前的样式损失和内容损失。
最后,将生成的图像进行最后一次修正,将其像素值限制在 0 到 1 之间,并返回最终的输出图像。
var_list = model.trainable_variables这里的model怎么导
您可以通过定义一个继承自 `tf.keras.Model` 的模型类来创建一个模型实例,并在训练时使用这个模型实例。以下是一个简单的例子:
```
import tensorflow as tf
class MyModel(tf.keras.Model):
def __init__(self):
super(MyModel, self).__init__()
self.dense1 = tf.keras.layers.Dense(10, activation='relu')
self.dense2 = tf.keras.layers.Dense(1)
def call(self, inputs):
x = self.dense1(inputs)
return self.dense2(x)
model = MyModel()
```
在这个例子中,我们定义了一个继承自 `tf.keras.Model` 的模型类 `MyModel`,并在 `__init__` 方法中定义了两个全连接层。在 `call` 方法中,我们将输入数据传入第一个全连接层,然后将输出传入第二个全连接层。这个模型可以接受任意维度的输入数据,输出一个维度为 1 的标量。
现在,您就可以使用 `model.trainable_variables` 来获取这个模型中的可训练变量了。例如,您可以像下面这样定义一个损失函数和优化器,并使用 `model.trainable_variables` 来更新模型中的变量:
```
optimizer = tf.keras.optimizers.Adam()
loss_fn = tf.keras.losses.MeanSquaredError()
for x, y in dataset:
with tf.GradientTape() as tape:
y_pred = model(x)
loss = loss_fn(y, y_pred)
gradients = tape.gradient(loss, model.trainable_variables)
optimizer.apply_gradients(zip(gradients, model.trainable_variables))
```
在这个例子中,我们使用了 `tf.GradientTape` 来计算损失函数的梯度,并使用 `optimizer.apply_gradients` 方法来更新模型中的变量。注意,在计算梯度时,我们使用了 `model.trainable_variables` 来获取模型中的可训练变量。
相关推荐
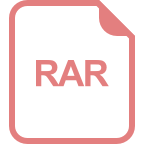
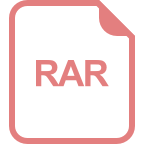
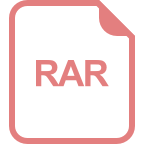












