pytorch声音分类
时间: 2023-06-30 13:13:01 浏览: 56
Pyorch可以用于声音分类任务,通常使用卷积神经网络(CNN)或循环神经网络(RNN)来处理音频信号。以下是一个简单的声音分类模型示例:
```python
import torch
import torch.nn as nn
class SoundClassifier(nn.Module):
def __init__(self):
super(SoundClassifier, self).__init__()
self.conv1 = nn.Conv1d(in_channels=1, out_channels=16, kernel_size=3)
self.pool = nn.MaxPool1d(kernel_size=2)
self.conv2 = nn.Conv1d(in_channels=16, out_channels=32, kernel_size=3)
self.fc1 = nn.Linear(in_features=32 * 39, out_features=128)
self.fc2 = nn.Linear(in_features=128, out_features=10)
def forward(self, x):
x = self.pool(torch.relu(self.conv1(x)))
x = self.pool(torch.relu(self.conv2(x)))
x = x.view(-1, 32 * 39)
x = torch.relu(self.fc1(x))
x = self.fc2(x)
return x
```
在这个模型中,输入是一维的音频信号,经过两层卷积和池化层,然后通过两个全连接层输出类别概率。可以使用交叉熵损失函数来训练这个模型,例如:
```python
criterion = nn.CrossEntropyLoss()
optimizer = torch.optim.SGD(model.parameters(), lr=0.01)
for epoch in range(num_epochs):
for i, (inputs, labels) in enumerate(train_loader):
optimizer.zero_grad()
outputs = model(inputs)
loss = criterion(outputs, labels)
loss.backward()
optimizer.step()
```
其中`train_loader`是一个PyTorch数据加载器,用于加载训练数据。在训练完成后,可以使用模型对新的音频信号进行分类:
```python
model.eval()
with torch.no_grad():
inputs = torch.Tensor(new_sound_signal).unsqueeze(0)
outputs = model(inputs)
predicted_class = torch.argmax(outputs).item()
```
相关推荐
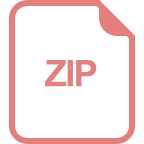














