gradient2 = ctx2.createRadialGradient(half, half, 0, half, half, half);
时间: 2023-10-11 20:13:06 浏览: 15
这段代码创建了一个径向渐变对象 gradient2,具体来说是使用 createRadialGradient() 方法创建的。这个方法可以接收 6 个参数,分别表示内圆的圆心坐标(x0、y0)、内圆的半径(r0)、外圆的圆心坐标(x1、y1)和外圆的半径(r1)。在这个例子中,x0 和 y0 的值都为 half,即画布的中心点,r0 的值为 0,表示内圆半径为 0,x1 和 y1 的值仍然为 half,r1 的值为 half,表示外圆半径为画布宽度的一半。这样就创建了一个从画布中心向四周扩散的径向渐变对象。
相关问题
norm = np.linalg.norm(gradient, ord=2)什么意思
在Python中,np.linalg.norm(gradient, ord=2)是用于计算矢量或矩阵的范数(norm)的函数。其中,gradient是一个向量或者矩阵,ord参数则指定了所使用的范数类型。当ord=2时,表示使用欧几里得范数,也称为L2范数,计算方式为所有元素的平方和开根号。因此,norm = np.linalg.norm(gradient, ord=2)的意思是计算gradient矢量的L2范数,并将其赋值给norm变量。
解释下面代码 addBubble: function (bubble) { var thisBubble = this.getBubble(bubble.x, bubble.y); thisBubble.color = bubble.color; }, setBubble: function (x, y, color) { this.getBubble(x, y).color = color; }, getBubble: function (x, y) { if (x < 0 || y < 0 || x > game.cellCount || y > game.cellCount) return null; return this.bubbles[y][x]; }, isEmpty: function (x, y) { var bubble = this.getBubble(x, y); return !bubble.color; }, }; var Cell = function (x, y) { this.x = x; this.y = y; } var Bubble = function (x, y, color) { this.x = x; this.y = y; this.px = game.cellWidth * (this.x + 1) - game.cellWidth / 2; this.py = game.cellWidth * (this.y + 1) - game.cellWidth / 2; this.color = color; this.light = 10; }; Bubble.prototype.draw = function () { if (!this.color) { return; } var ctx = game.ctx; ctx.beginPath(); //console.log("x:" + px + "y:" + py); var gradient = ctx.createRadialGradient(this.px - 5, this.py - 5, 0, this.px, this.py, this.light); gradient.addColorStop(0, "white"); gradient.addColorStop(1, this.color); ctx.arc(this.px, this.py, 11, 0, Math.PI * 2); ctx.strokeStyle = this.color; ctx.fillStyle = gradient; ctx.fill(); ctx.stroke(); }; Bubble.prototype.play = function () { var me = this; var isUp = true; game.play("light_" + this.x + "" + this.y, function () { if (isUp) { me.light += 3; } if (!isUp) { me.light -= 3; } if (me.light >= 30) { isUp = false; } if (me.light <= 10) { isUp = true; } }, 50); }; Bubble.prototype.stop = function () { //this.light = 10; var me = this; game.stop("light" + this.x + "" + this.y); game.play("restore" + this.x + "" + this.y, function () { if (me.light > 10) { me.light--; } else { me.light = 10; game.stop("restore" + me.x + "_" + me.y); } }, 50); }; game.start(); </script> <div style="text-align:center;"> </div> <script src="http://www.mycodes.net/js/tongji.js"></script> <script src="http://www.mycodes.net/js/youxia.js" type="text/javascript">
这段代码实现了一个游戏中的泡泡射击功能,其中包括 Bubble 和 Cell 两个类。Bubble 类表示一个泡泡,包含位置和颜色等属性,还定义了绘制泡泡和播放动画的方法,如 draw() 和 play()。Cell 类表示游戏中的一个小格子,包含位置等属性。还有一些辅助方法,如 addBubble()、setBubble()、getBubble() 和 isEmpty(),用于操作和判断泡泡的状态。整个游戏的入口函数是 game.start(),在该函数中完成游戏的初始化和事件绑定等操作。
相关推荐
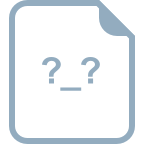
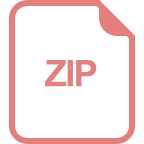













