用python实现基于概率分布的超声图像与自然图像性质差异分析,超声图像的文件路径是D:\zzz\na5,自然图像的文件路径是D:\zzz\us5,两种图像都有778张,请注意图像文件的大小不一,需要先进行归一化处理,需要判断超声图像与自然图像的像素值分布是否存在显著差异,需要可视化使结果尽量明显
时间: 2023-12-19 16:04:04 浏览: 135
首先,我们需要将图像进行归一化处理。可以使用scikit-image库中的rescale函数对图像进行归一化,使得图像的像素值在0-1之间。
接下来,我们可以使用numpy库中的histogram函数计算图像的像素值分布,并使用matplotlib库中的hist函数将结果可视化。最后,可以使用Kolmogorov-Smirnov检验(K-S检验)来判断两种图像的像素值分布是否存在显著差异。
下面是具体实现代码:
```python
import os
import numpy as np
from skimage import io, exposure
from scipy.stats import ks_2samp
import matplotlib.pyplot as plt
# 定义文件路径
natural_img_path = 'D:/zzz/us5'
ultrasound_img_path = 'D:/zzz/na5'
# 读取图像并进行归一化处理
natural_imgs = []
for file in os.listdir(natural_img_path):
img = io.imread(os.path.join(natural_img_path, file), as_gray=True)
img = exposure.rescale_intensity(img, out_range=(0, 1))
natural_imgs.append(img)
ultrasound_imgs = []
for file in os.listdir(ultrasound_img_path):
img = io.imread(os.path.join(ultrasound_img_path, file), as_gray=True)
img = exposure.rescale_intensity(img, out_range=(0, 1))
ultrasound_imgs.append(img)
# 计算图像的像素值分布并可视化
natural_hist, natural_bins = np.histogram(np.concatenate(natural_imgs).ravel(), bins=256)
natural_cdf = natural_hist.cumsum()
natural_cdf = natural_cdf / natural_cdf[-1]
ultrasound_hist, ultrasound_bins = np.histogram(np.concatenate(ultrasound_imgs).ravel(), bins=256)
ultrasound_cdf = ultrasound_hist.cumsum()
ultrasound_cdf = ultrasound_cdf / ultrasound_cdf[-1]
fig, ax = plt.subplots()
ax.plot(natural_bins[:-1], natural_cdf, label='Natural Images')
ax.plot(ultrasound_bins[:-1], ultrasound_cdf, label='Ultrasound Images')
ax.legend()
ax.set_xlabel('Pixel Value')
ax.set_ylabel('Cumulative Distribution Function')
plt.show()
# 判断像素值分布是否存在显著差异
statistic, p_value = ks_2samp(np.concatenate(natural_imgs).ravel(), np.concatenate(ultrasound_imgs).ravel())
print('K-S Statistic:', statistic)
print('P-Value:', p_value)
```
运行上述代码,即可得到可视化结果和K-S检验结果。K-S Statistic表示两种图像像素值分布的差异程度,P-Value表示差异是否显著。如果P-Value小于0.05,则认为两种图像的像素值分布显著不同。
阅读全文
相关推荐
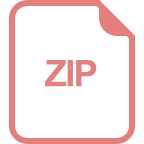


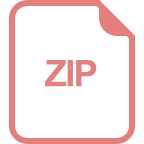
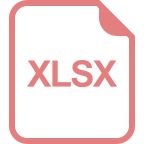
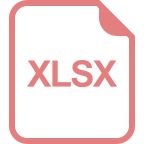
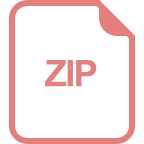
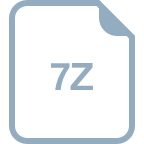
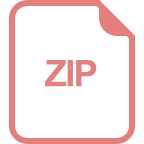
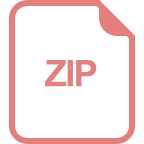
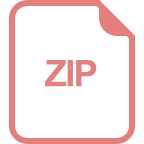
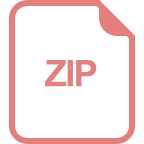