hyperopt贝叶斯优化xgboost超参数
时间: 2023-06-22 09:32:44 浏览: 125
Hyperopt是一个Python库,用于使用贝叶斯优化算法来调整机器学习模型的超参数。下面是Hyperopt在调整XGBoost超参数时的步骤:
1. 定义参数空间:首先需要定义需要调整的超参数以及其取值范围。例如,可以使用Uniform分布来定义连续型参数的取值范围,使用qUniform分布来定义整数型参数的取值范围。
2. 定义评估函数:评估函数是用来计算模型的性能指标的,例如准确率、AUC等。在每次迭代中,Hyperopt会根据当前超参数的取值调用评估函数来计算模型的性能指标。
3. 定义搜索算法:Hyperopt支持多种搜索算法,例如随机搜索、贝叶斯优化等。在这里,我们选择使用贝叶斯优化算法。
4. 运行优化器:定义好参数空间、评估函数和搜索算法后,就可以运行Hyperopt的优化器来寻找最优超参数组合了。在每次迭代中,Hyperopt会根据当前的超参数取值计算模型的性能指标,并根据贝叶斯优化算法来更新超参数的取值,直到达到预设的最大迭代次数或收敛为止。
下面是一个使用Hyperopt优化XGBoost超参数的示例代码:
```python
from hyperopt import fmin, tpe, hp
from sklearn.datasets import load_boston
from sklearn.metrics import mean_squared_error
from sklearn.model_selection import train_test_split
import xgboost as xgb
# 加载数据集
data = load_boston()
X_train, X_test, y_train, y_test = train_test_split(data.data, data.target, test_size=0.2, random_state=42)
# 定义参数空间
space = {
'max_depth': hp.quniform('max_depth', 3, 10, 1),
'learning_rate': hp.loguniform('learning_rate', -5, 0),
'n_estimators': hp.quniform('n_estimators', 50, 200, 1),
'min_child_weight': hp.quniform('min_child_weight', 1, 10, 1),
'subsample': hp.uniform('subsample', 0.5, 1),
'gamma': hp.uniform('gamma', 0, 1),
'colsample_bytree': hp.uniform('colsample_bytree', 0.5, 1),
'reg_alpha': hp.uniform('reg_alpha', 0, 1),
'reg_lambda': hp.uniform('reg_lambda', 0, 1),
}
# 定义评估函数
def objective(params):
model = xgb.XGBRegressor(**params)
model.fit(X_train, y_train)
y_pred = model.predict(X_test)
mse = mean_squared_error(y_test, y_pred)
return mse
# 定义搜索算法
algo = tpe.suggest
# 运行优化器
best = fmin(fn=objective, space=space, algo=algo, max_evals=100)
print(best)
```
在这个示例中,我们使用Hyperopt库来优化XGBoost回归模型的超参数。我们首先加载了Boston房价数据集,并将其分成训练集和测试集。然后,我们定义了需要调整的超参数以及其取值范围,并定义了评估函数。最后,我们选择使用tpe.suggest算法来搜索最优超参数,并将最优超参数打印出来。
需要注意的是,由于贝叶斯优化算法是一种启发式算法,因此在每次运行时得到的最优超参数可能会有所不同。因此,为了确保得到的结果是稳定的,通常需要运行多次优化器并取平均值。
相关推荐
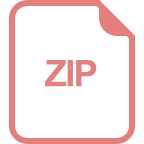














