基于unet的pytorch细胞图像分割代码
时间: 2023-05-26 20:03:23 浏览: 131
以下是一个基于Unet的PyTorch细胞图像分割代码示例:
```python
import torch
import torch.nn as nn
import torch.nn.functional as F
class Unet(nn.Module):
def __init__(self):
super(Unet, self).__init__()
# encoder
self.conv1 = nn.Conv2d(3, 64, 3, padding=1)
self.bn1 = nn.BatchNorm2d(64)
self.conv2 = nn.Conv2d(64, 64, 3, padding=1)
self.bn2 = nn.BatchNorm2d(64)
self.pool1 = nn.MaxPool2d(2)
self.conv3 = nn.Conv2d(64, 128, 3, padding=1)
self.bn3 = nn.BatchNorm2d(128)
self.conv4 = nn.Conv2d(128, 128, 3, padding=1)
self.bn4 = nn.BatchNorm2d(128)
self.pool2 = nn.MaxPool2d(2)
self.conv5 = nn.Conv2d(128, 256, 3, padding=1)
self.bn5 = nn.BatchNorm2d(256)
self.conv6 = nn.Conv2d(256, 256, 3, padding=1)
self.bn6 = nn.BatchNorm2d(256)
self.pool3 = nn.MaxPool2d(2)
self.conv7 = nn.Conv2d(256, 512, 3, padding=1)
self.bn7 = nn.BatchNorm2d(512)
self.conv8 = nn.Conv2d(512, 512, 3, padding=1)
self.bn8 = nn.BatchNorm2d(512)
self.pool4 = nn.MaxPool2d(2)
# decoder
self.upconv1 = nn.ConvTranspose2d(512, 256, 2, stride=2)
self.conv9 = nn.Conv2d(512, 256, 3, padding=1)
self.bn9 = nn.BatchNorm2d(256)
self.conv10 = nn.Conv2d(256, 256, 3, padding=1)
self.bn10 = nn.BatchNorm2d(256)
self.upconv2 = nn.ConvTranspose2d(256, 128, 2, stride=2)
self.conv11 = nn.Conv2d(256, 128, 3, padding=1)
self.bn11 = nn.BatchNorm2d(128)
self.conv12 = nn.Conv2d(128, 128, 3, padding=1)
self.bn12 = nn.BatchNorm2d(128)
self.upconv3 = nn.ConvTranspose2d(128, 64, 2, stride=2)
self.conv13 = nn.Conv2d(128, 64, 3, padding=1)
self.bn13 = nn.BatchNorm2d(64)
self.conv14 = nn.Conv2d(64, 64, 3, padding=1)
self.bn14 = nn.BatchNorm2d(64)
# output
self.conv15 = nn.Conv2d(64, 1, 1)
def forward(self, x):
# encoder
x1 = F.relu(self.bn1(self.conv1(x)))
x1 = F.relu(self.bn2(self.conv2(x1)))
x2 = self.pool1(x1)
x2 = F.relu(self.bn3(self.conv3(x2)))
x2 = F.relu(self.bn4(self.conv4(x2)))
x3 = self.pool2(x2)
x3 = F.relu(self.bn5(self.conv5(x3)))
x3 = F.relu(self.bn6(self.conv6(x3)))
x4 = self.pool3(x3)
x4 = F.relu(self.bn7(self.conv7(x4)))
x4 = F.relu(self.bn8(self.conv8(x4)))
x5 = self.pool4(x4)
# decoder
x5 = self.upconv1(x5)
x5 = torch.cat([x3, x5], dim=1)
x5 = F.relu(self.bn9(self.conv9(x5)))
x5 = F.relu(self.bn10(self.conv10(x5)))
x4 = self.upconv2(x5)
x4 = torch.cat([x2, x4], dim=1)
x4 = F.relu(self.bn11(self.conv11(x4)))
x4 = F.relu(self.bn12(self.conv12(x4)))
x3 = self.upconv3(x4)
x3 = torch.cat([x1, x3], dim=1)
x3 = F.relu(self.bn13(self.conv13(x3)))
x3 = F.relu(self.bn14(self.conv14(x3)))
# output
out = torch.sigmoid(self.conv15(x3))
return out
```
在这个Unet模型中,输入图像的大小为256x256x3,输出是一个二进制图像,用于分割目标。
我们可以使用以下代码训练模型:
```python
import torch.optim as optim
from torch.utils.data import DataLoader
# 设置训练参数
lr = 0.001
num_epochs = 10
batch_size = 32
# 加载数据集
train_data = MyDataset(training_data)
train_loader = DataLoader(train_data, batch_size=batch_size, shuffle=True)
# 创建模型和优化器
model = Unet()
optimizer = optim.Adam(model.parameters(), lr=lr)
# 训练网络
for epoch in range(num_epochs):
for batch_idx, (data, target) in enumerate(train_loader):
optimizer.zero_grad()
output = model(data)
loss = nn.BCELoss()(output, target)
loss.backward()
optimizer.step()
if batch_idx % 10 == 0:
print('Epoch: {} [{}/{} ({:.0f}%)]\tLoss: {:.6f}'.format(
epoch+1, batch_idx * len(data), len(train_loader.dataset),
100. * batch_idx / len(train_loader), loss.item()))
```
这里使用了BCELoss作为损失函数,Adam优化器作为优化器。
可以使用以下代码测试模型:
```python
# 加载测试数据集
test_data = MyDataset(test_data)
test_loader = DataLoader(test_data, batch_size=batch_size, shuffle=True)
# 测试模型
model.eval()
test_loss = 0
correct = 0
with torch.no_grad():
for data, target in test_loader:
output = model(data)
# 计算损失
test_loss += nn.BCELoss()(output, target).item()
# 计算精度
pred = output.round()
correct += pred.eq(target.view_as(pred)).sum().item()
test_loss /= len(test_loader.dataset)
accuracy = 100. * correct / len(test_loader.dataset)
print('Test set: Average loss: {:.4f}, Accuracy: {}/{} ({:.0f}%)'.format(
test_loss, correct, len(test_loader.dataset),
accuracy))
```
阅读全文
相关推荐
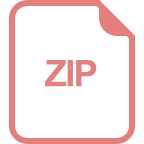
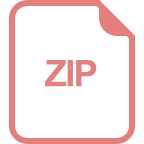
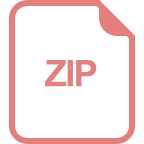
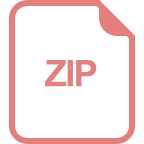
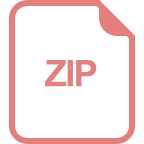
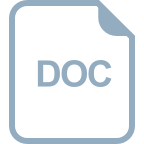
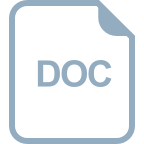
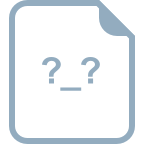
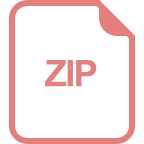
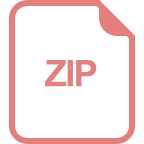
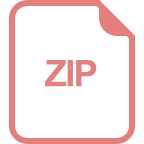
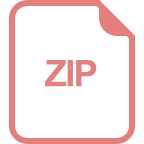
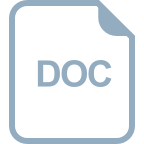
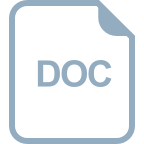
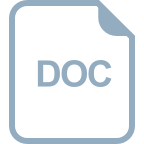
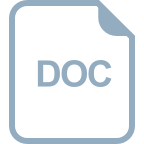
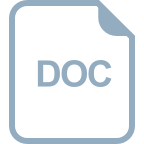
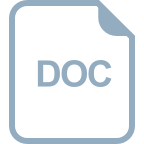