怎么在已经写好的yolov4代码,即可以识别apple_right和apple_down两类标签,怎么在这yolov4的predict程序里面加入当识别为apple_right时没变化,当识别apple_down时,加入轮廓提取的代码
时间: 2023-12-10 21:40:57 浏览: 118
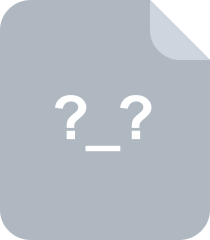
轮廓提取代码
要实现这个功能,你需要对yolov4的predict程序做出一些修改。具体步骤如下:
1. 首先,你需要修改yolov4模型的输出。yolov4默认的输出是一个列表,包含了每个检测到的物体的类别、置信度和坐标信息。你需要将其改为一个字典,其中键为类别名称,值为检测到该类别的物体的坐标信息。
2. 接着,你需要在代码中加入轮廓提取的功能。可以使用OpenCV库中的findContours函数来实现。首先,将检测到的物体的坐标信息转换成OpenCV中的矩形框表示法,然后使用findContours函数提取物体的轮廓。
3. 最后,你需要根据类别名称来判断是否需要进行轮廓提取。当检测到的物体属于apple_down类别时,才需要进行轮廓提取。否则,直接返回原始的检测结果。
下面是一个伪代码示例,帮助你更好地理解这个过程:
``` python
# 定义类别名称和类别编号
class_names = ["apple_right", "apple_down"]
class_ids = {"apple_right": 0, "apple_down": 1}
# 修改yolov4模型的输出
def yolov4_predict(model, image):
bbox_list = model.predict(image)
result_dict = {}
for i, bbox in enumerate(bbox_list):
class_id = np.argmax(bbox[5:])
class_name = class_names[class_id]
if class_name not in result_dict:
result_dict[class_name] = []
result_dict[class_name].append(bbox[:4])
return result_dict
# 加入轮廓提取的功能
def extract_contours(image, bbox):
x1, y1, x2, y2 = bbox
roi = image[y1:y2, x1:x2]
gray = cv2.cvtColor(roi, cv2.COLOR_BGR2GRAY)
ret, thresh = cv2.threshold(gray, 0, 255, cv2.THRESH_BINARY_INV+cv2.THRESH_OTSU)
contours, hierarchy = cv2.findContours(thresh, cv2.RETR_EXTERNAL, cv2.CHAIN_APPROX_SIMPLE)
return contours
# 根据类别名称进行判断
def process_bbox(image, bbox, class_name):
class_id = class_ids[class_name]
if class_id == 1: # 如果是apple_down类别,则进行轮廓提取
contours = extract_contours(image, bbox)
# 对轮廓进行处理...
# ...
# 返回处理后的结果
return processed_result
else: # 否则,直接返回原始结果
return bbox
# 在主程序中调用yolov4_predict函数,并根据类别名称进行处理
result_dict = yolov4_predict(model, image)
for class_name, bbox_list in result_dict.items():
for bbox in bbox_list:
processed_result = process_bbox(image, bbox, class_name)
# 处理processed_result...
# ...
```
注意,这只是一个伪代码示例,你需要根据具体的代码实现情况进行修改。另外,对于轮廓提取的过程,需要根据具体的需求进行处理,比如筛选轮廓、计算轮廓面积等。
阅读全文
相关推荐

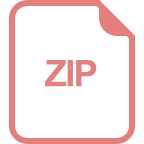
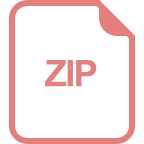
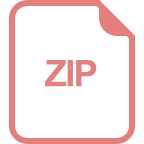
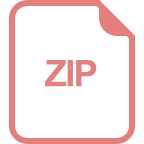
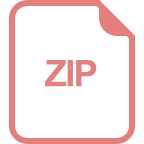
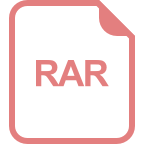
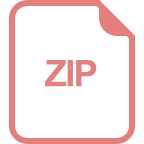
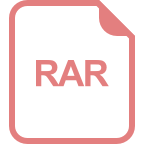
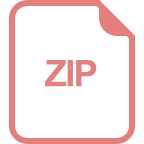
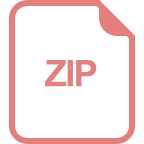