tensorflow2 模型 推理
时间: 2023-07-28 20:07:31 浏览: 42
TensorFlow 2.x 提供了多种方式进行模型推理,以下是其中的两种常用方式:
1. 使用 `model.predict()` 进行推理:
```python
import tensorflow as tf
# 加载模型
model = tf.keras.models.load_model('path/to/model.h5')
# 加载数据
x_test = ...
y_test = ...
# 进行推理
predictions = model.predict(x_test)
# 打印结果
print(predictions)
```
2. 使用 `tf.function` 进行推理:
```python
import tensorflow as tf
# 加载模型
model = tf.keras.models.load_model('path/to/model.h5')
# 定义推理函数
@tf.function
def inference(x):
return model(x)
# 加载数据
x_test = ...
y_test = ...
# 进行推理
predictions = inference(x_test)
# 打印结果
print(predictions)
```
以上两种方式都可以用于模型推理,具体选择哪种方式取决于你的需求和使用场景。
相关问题
tensorflow2模型保存导入
在 TensorFlow 2 中,保存和导入模型可以使用 `tf.keras` 模块的 API。以下是一个简单的示例:
1. 保存模型
```python
import tensorflow as tf
# 定义模型
model = tf.keras.Sequential([
tf.keras.layers.Dense(64, activation='relu'),
tf.keras.layers.Dense(10, activation='softmax')
])
# 编译模型
model.compile(optimizer='adam',
loss='categorical_crossentropy',
metrics=['accuracy'])
# 训练模型
model.fit(x_train, y_train, epochs=10)
# 保存模型
model.save('my_model')
```
2. 导入模型
```python
import tensorflow as tf
# 导入模型
loaded_model = tf.keras.models.load_model('my_model')
# 使用模型进行推理
result = loaded_model.predict(x_test)
```
在导入模型时,可以使用 `tf.keras.models.load_model` 函数加载已保存的模型。然后,您可以使用模型进行推理,就像在训练期间一样。
tensorflow调用模型代码
以下是一个简单的 TensorFlow 调用模型的代码示例:
1. 加载模型
```python
import tensorflow as tf
# 加载模型
model = tf.keras.models.load_model('path/to/model')
```
2. 预处理输入数据
```python
import numpy as np
# 预处理输入数据
input_data = np.array([[1, 2, 3, 4]])
```
3. 进行推理
```python
# 进行推理
output = model.predict(input_data)
```
4. 处理输出数据
```python
# 处理输出数据
result = np.argmax(output, axis=1)
# 输出结果
print(result)
```
这样就可以调用 TensorFlow 模型进行推理。其中,需要注意的是,模型的输入和输出都需要进行预处理和后处理,以适配模型的要求和输出格式。同时,需要根据具体的模型和场景,选择适合的预处理和后处理方法。
相关推荐
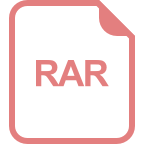
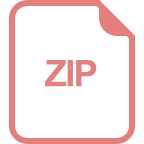
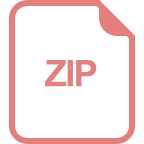
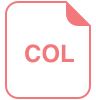
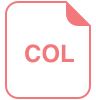
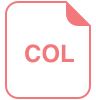
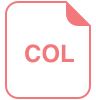
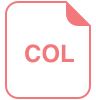







