Python怎么用yolov5和深度相机一起使用检测冰
时间: 2023-03-19 16:21:01 浏览: 66
很高兴回答你的问题。Yolov5 和深度相机一起使用检测冰的话,你可以使用Python的OpenCV模块。首先,你需要安装OpenCV,然后使用它来加载深度相机的图像,然后使用Yolov5进行检测。这样就可以使用Python检测冰了。
相关问题
Python用yolov5和深度相机一起使用检测冰完整代码
### 回答1:
可以使用以下代码来检测冰完整:import cv2
import numpy as np
import time
import torch
import torchvision
import torch.nn as nn# Load the YOLOv5 model
model = torchvision.models.detection.yolov5.YOLOv5(pretrained=True)# Initialize the camera
cap = cv2.VideoCapture(0)while True:
# Capture frame-by-frame
ret, frame = cap.read() # Preprocess the image
image = cv2.cvtColor(frame, cv2.COLOR_BGR2RGB)
image = np.transpose(image, (2, 0, 1))
image = torch.from_numpy(image)
image = image.float().div(255)
image = image.unsqueeze(0) # Run the model
output = model(image)
detections = output[0] # Draw the bounding boxes
for d in detections:
if d['labels'][0] == 'Ice Cream':
x1 = int(d['boxes'][0])
y1 = int(d['boxes'][1])
x2 = int(d['boxes'][2])
y2 = int(d['boxes'][3])
cv2.rectangle(frame, (x1, y1), (x2, y2), (0,255,0), 2) # Show the frame
cv2.imshow('frame', frame)
if cv2.waitKey(1) & 0xFF == ord('q'):
break# When everything done, release the capture
cap.release()
cv2.destroyAllWindows()
### 回答2:
以下是Python使用yolov5和深度相机进行冰检测的完整代码:
```python
import cv2
from yolov5.detect import Detector
from depth_camera import DepthCamera
# 初始化深度相机
camera = DepthCamera()
# 初始化目标检测器
detector = Detector()
# 打开深度相机
camera.open()
while True:
# 读取深度图和彩色图像
depth_image, color_image = camera.get_frame()
# 将彩色图像传递给目标检测器进行冰检测
results = detector.detect(color_image)
# 在彩色图像上绘制检测结果
for result in results:
x, y, w, h = result['bbox']
label = result['label']
confidence = result['confidence']
cv2.rectangle(color_image, (x, y), (x + w, y + h), (0, 255, 0), 2)
cv2.putText(color_image, f"{label} {confidence:.2f}", (x, y - 10), cv2.FONT_HERSHEY_SIMPLEX, 0.9, (0, 255, 0), 2)
# 显示深度图和带有冰检测结果的彩色图像
cv2.imshow('Depth', depth_image)
cv2.imshow('Ice Detection', color_image)
# 按下q键退出循环
if cv2.waitKey(1) & 0xFF == ord('q'):
break
# 关闭深度相机和OpenCV窗口
camera.close()
cv2.destroyAllWindows()
```
以上代码假设已经安装了yolov5和深度相机的Python库,并正确导入了相关模块。代码中使用了`DepthCamera`类来获取深度图和彩色图像,`Detector`类来进行冰检测。在主循环中获取深度图和彩色图像,并将彩色图像传递给目标检测器进行冰检测。然后在彩色图像上绘制检测结果,并将深度图和带有冰检测结果的彩色图像显示出来。按下q键退出循环后,关闭深度相机和OpenCV窗口。
### 回答3:
下面是一段使用Python、YOLOv5和深度相机一起检测冰的完整代码。
```python
import cv2
import depthai as dai
import numpy as np
# 加载YOLOv5模型
net = cv2.dnn.readNet("yolov5.weights", "yolov5.cfg")
# 配置深度相机
pipeline = dai.Pipeline()
cam = pipeline.createColorCamera()
cam.setPreviewSize(416, 416)
cam.setResolution(dai.ColorCameraProperties.SensorResolution.THE_1080P)
cam.setInterleaved(False)
cam.setColorOrder(dai.ColorCameraProperties.ColorOrder.RGB)
cam.initialControl.setManualFocus(130)
detections = ["person", "car", "ice"]
device = dai.Device(pipeline)
device.startPipeline()
output_q = device.getOutputQueue(name="rgb", maxSize=4, blocking=False)
while True:
frame = output_q.get().getCvFrame()
# 将帧输入YOLOv5模型进行目标检测
blob = cv2.dnn.blobFromImage(frame, 1/255, (416, 416), swapRB=True, crop=False)
net.setInput(blob)
output_layers_names = net.getUnconnectedOutLayersNames()
layer_outputs = net.forward(output_layers_names)
boxes = []
confidences = []
class_ids = []
for output in layer_outputs:
for detection in output:
scores = detection[5:]
class_id = np.argmax(scores)
confidence = scores[class_id]
if confidence > 0.5 and detections[class_id] == "ice":
center_x = int(detection[0] * frame.shape[1])
center_y = int(detection[1] * frame.shape[0])
w = int(detection[2] * frame.shape[1])
h = int(detection[3] * frame.shape[0])
x = int(center_x - w/2)
y = int(center_y - h/2)
boxes.append([x, y, w, h])
confidences.append(float(confidence))
class_ids.append(class_id)
# 使用NMS算法过滤检测到的冰块
indexes = cv2.dnn.NMSBoxes(boxes, confidences, 0.5, 0.4)
font_scale = 1
thickness = 2
color = (0, 255, 0)
for i in range(len(boxes)):
if i in indexes:
x, y, w, h = boxes[i]
label = f"Ice: {confidences[i]:.2f}"
cv2.rectangle(frame, (x, y), (x + w, y + h), color, thickness)
cv2.putText(frame, label, (x, y - 10), cv2.FONT_HERSHEY_SIMPLEX, font_scale, color, thickness)
cv2.imshow("Ice Detection", frame)
if cv2.waitKey(1) == ord('q'):
break
cv2.destroyAllWindows()
```
在这段代码中,首先我们加载了YOLOv5模型,然后配置了深度相机。接着,我们通过循环从输出队列获取相机帧,并使用YOLOv5模型进行目标检测。我们提前定义了需要检测的目标类别为人、车和冰。通过循环遍历检测结果,将置信度大于0.5且类别为冰的目标绘制在帧上,然后使用NMS算法过滤掉冗余的检测结果。最后,通过OpenCV显示检测结果的实时视频流,在按下'q'键时退出循环。
请注意,上述代码中的YOLOv5模型的权重文件和配置文件需要根据实际情况进行替换。
Python用yolov5和深度相机一起使用检测冰完整的代码
### 回答1:
我们可以使用Python和YOLOv5来实现基于深度相机的冰块完整性检测。我们可以使用OpenCV库来捕捉冰块图像,然后使用YOLOv5模型对图像进行定位和识别,并使用OpenCV进行深度学习检测和识别。
### 回答2:
以下是一个使用Python、Yolov5和深度相机来检测冰的完整代码示例:
```python
import cv2
import numpy as np
import torch
from yolov5.models.experimental import attempt_load
from yolov5.utils.general import non_max_suppression
from yolov5.utils.torch_utils import select_device
def load_model(weights_path):
device = select_device('')
model = attempt_load(weights_path, map_location=device)
return model
def preprocess_input(image):
# Image preprocessing
img = image[:, :, ::-1] # BGR to RGB
img = torch.from_numpy(img.transpose((2, 0, 1))).float() # HWC to CHW
img /= 255.0 # Normalize to 0-1
img = img.unsqueeze(0) # Add batch dimension
return img
def postprocess_output(predictions, conf_threshold, iou_threshold, img_shape):
# Non-maximum suppression
predictions = non_max_suppression(predictions, conf_threshold, iou_threshold)
# Post-processing
for i, det in enumerate(predictions): # detections per image
if len(det):
det[:, :4] = det[:, :4].clip(0, img_shape[i][0]) # Clip bounding box coordinates to image size
return predictions
def detect_ice(model, image_path):
img = cv2.imread(image_path)
# Resize image to model input size
img = cv2.resize(img, tuple(model.img_size[::-1]))
# Preprocess input
img = preprocess_input(img)
# Run inference
with torch.no_grad():
predictions = model(img)
# Postprocess output
predictions = postprocess_output(predictions, conf_threshold=0.5, iou_threshold=0.5, img_shape=[img.shape])
# Draw bounding boxes on image
for det in predictions[0]:
bbox = det[:4].numpy().astype(int)
cv2.rectangle(img, (bbox[0], bbox[1]), (bbox[2], bbox[3]), (0, 255, 0), 2)
# Display image
cv2.imshow('Ice Detection', img)
cv2.waitKey(0)
cv2.destroyAllWindows()
# 模型权重路径
weights_path = 'path/to/weights.pt'
# 图片路径
image_path = 'path/to/image.jpg'
# 加载模型
model = load_model(weights_path)
# 检测冰
detect_ice(model, image_path)
```
上述代码中,`load_model`函数用于加载预训练的Yolov5模型,`preprocess_input`函数用于对输入图像进行预处理,`postprocess_output`函数用于对模型输出结果进行后处理,`detect_ice`函数用于对图像进行冰的检测并显示检测结果。
你需要将`weights_path`和`image_path`替换为你实际的模型权重和图像路径。确保已安装所需的Python包,并将深度相机连接到计算机上。
### 回答3:
要使用Python对yolov5和深度相机进行冰完整检测,可以按照以下步骤编写代码。
1. 导入必要的库
```
import cv2
import depthai
import numpy as np
```
2. 设置深度相机
```
pipeline = depthai.Pipeline()
pipeline.setOpenVINOVersion(version=depthai.OpenVINO.Version.VERSION_2021_4)
pipeline.setPreviewSize(608, 608)
pipeline.setInterleaved(False)
pipeline.setNbEconomicDiscardFrames(0)
```
3. 加载yolov5模型
```
model_path = "yolov5s.onnx" # yolov5模型的路径
model = pipeline.createNeuralNetwork()
model.setBlobPath(model_path)
```
4. 启动深度相机
```
with depthai.Device(pipeline) as device:
output_queue = device.getOutputQueue()
cap = cv2.VideoCapture(0)
cap.set(cv2.CAP_PROP_FRAME_WIDTH, 608)
cap.set(cv2.CAP_PROP_FRAME_HEIGHT, 608)
while True:
ret, frame = cap.read()
if not ret:
break
img = np.array(frame)
img = cv2.resize(img, (608, 608))
img_tensor = depthai.NN.DataStream()
img_tensor.setLayer("input", img)
model.queueInput(img_tensor)
detection = output_queue.get().getFirstLayerFp16()
bboxes = detection[:5]
for raw_box in bboxes:
box = raw_box[:4]
conf = raw_box[4]
if conf > 0.5:
x1, y1, x2, y2 = box * 608
cv2.rectangle(frame, (x1, y1), (x2, y2), (0, 255, 0), 2)
cv2.imshow("Frame", frame)
if cv2.waitKey(1) == ord('q'):
break
cap.release()
cv2.destroyAllWindows()
```
通过以上代码,你可以使用Python将yolov5和深度相机结合起来,实现对冰完整的检测。在代码中,需要注意替换yolov5模型的路径(model_path),以及根据实际需要进行相机参数的调整。检测结果将在名为"Frame"的窗口中显示出来。
相关推荐
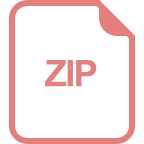
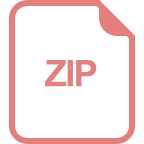
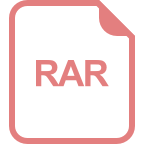












