lstm 文字情感分析
时间: 2023-07-31 22:04:09 浏览: 42
LSTM是一种循环神经网络模型,常用于处理序列数据,例如自然语言文本数据。在文字情感分析中,LSTM可以通过学习大量的文本数据,来识别出不同的情感类别,例如正面、负面、中性等。LSTM可以通过记忆单元和门控机制来捕捉长期的依赖关系,从而更好地理解文本数据中的语义信息。同时,LSTM也可以处理变长的序列数据,这使得它在处理自然语言文本数据时非常有用。
相关问题
lstm模型能干什么
LSTM(Long Short-Term Memory)是一种循环神经网络(RNN)的变体,它在处理序列数据时具有很强的能力。LSTM模型可以用于以下几个方面:
1. 序列建模:LSTM可以对序列数据进行建模,例如自然语言处理中的文本生成、机器翻译、语音识别等任务。通过学习序列中的上下文信息,LSTM可以预测下一个单词、生成连贯的句子或者将一种语言翻译成另一种语言。
2. 时间序列预测:LSTM可以用于时间序列数据的预测,例如股票价格预测、天气预测等。通过学习时间序列中的模式和趋势,LSTM可以预测未来的数值或者趋势变化。
3. 情感分析:LSTM可以用于情感分析任务,例如判断一段文本的情感倾向是积极还是消极。通过学习文本中的情感特征,LSTM可以对文本进行情感分类或者情感打分。
4. 语音识别:LSTM可以用于语音识别任务,例如将语音转换成文本。通过学习语音信号中的特征和模式,LSTM可以将语音信号转化为对应的文字。
除了tfidf算法,还有哪些常用的文字识别算法?
除了TF-IDF算法之外,常用的文本识别算法还有以下几种:
1. 朴素贝叶斯算法:一种基于贝叶斯定理和特征独立假设的分类算法,常用于文本分类和垃圾邮件过滤等领域。
2. 支持向量机(SVM)算法:一种基于结构风险最小化理论的二分类模型,常用于文本分类、情感分析等领域。
3. 深度学习算法:包括循环神经网络(RNN)、长短时记忆网络(LSTM)和卷积神经网络(CNN)等模型,常用于文本分类、情感分析、机器翻译等领域。
4. KNN算法:一种基于最近邻思想的分类算法,常用于文本分类、推荐系统等领域。
相关推荐
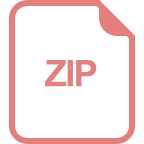
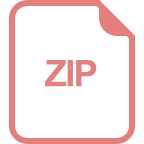







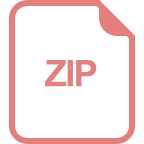
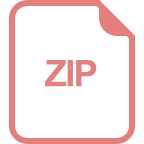
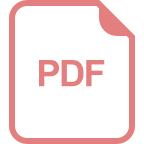
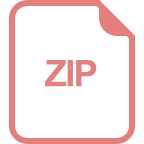
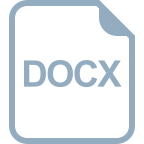
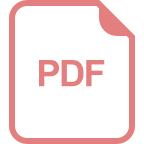