编写一个python程序,使用 numpy 编写逻辑回归算法,对 iris 数据进行二分类,具体内容: (1)任选两个特征和两个类别进行二分类。 (2)输出:决策函数的参数、预测值、分类准确率等。 (3)可视化:选取两个特征进行散点图可视化,并可视化决策边界。
时间: 2024-02-20 18:59:12 浏览: 78
好的,下面是使用numpy编写逻辑回归算法,对iris数据进行二分类的Python程序。具体实现过程如下:
1. 导入需要的库:numpy、pandas、matplotlib。
2. 加载iris数据集,并选择其中的两个特征和两个类别进行二分类。这里我们选择“花萼长度”和“花瓣长度”这两个特征,以及“山鸢尾”和“变色鸢尾”这两个类别。
3. 将数据集分为训练集和测试集,使用训练集训练逻辑回归模型,得到模型参数。这里我们使用梯度下降算法来进行模型训练。
4. 使用测试集对模型进行测试,计算模型的分类准确率,并输出预测结果。
5. 可视化:选取两个特征进行散点图可视化,并可视化决策边界。
下面是完整代码:
```python
import numpy as np
import pandas as pd
import matplotlib.pyplot as plt
# 定义sigmoid函数
def sigmoid(z):
return 1 / (1 + np.exp(-z))
# 定义损失函数
def loss(h, y):
return (-y * np.log(h) - (1 - y) * np.log(1 - h)).mean()
# 定义逻辑回归模型
class LogisticRegression:
def __init__(self, learning_rate=0.1, num_iterations=10000):
self.learning_rate = learning_rate
self.num_iterations = num_iterations
self.weights = None
self.bias = None
def fit(self, X, y):
# 初始化模型参数
n_samples, n_features = X.shape
self.weights = np.zeros(n_features)
self.bias = 0
# 梯度下降算法
for i in range(self.num_iterations):
z = np.dot(X, self.weights) + self.bias
h = sigmoid(z)
gradient_weights = np.dot(X.T, (h - y)) / n_samples
gradient_bias = np.sum(h - y) / n_samples
self.weights -= self.learning_rate * gradient_weights
self.bias -= self.learning_rate * gradient_bias
# 每1000次迭代输出一次损失函数值
if i % 1000 == 0:
z = np.dot(X, self.weights) + self.bias
h = sigmoid(z)
print(f'Loss after iteration {i}: {loss(h, y)}')
def predict(self, X):
z = np.dot(X, self.weights) + self.bias
h = sigmoid(z)
y_pred = [1 if i > 0.5 else 0 for i in h]
return y_pred
def accuracy(self, y_true, y_pred):
accuracy = np.sum(y_true == y_pred) / len(y_true)
return accuracy
# 加载iris数据集
iris = pd.read_csv('https://archive.ics.uci.edu/ml/machine-learning-databases/iris/iris.data', header=None)
iris.columns = ['sepal_length', 'sepal_width', 'petal_length', 'petal_width', 'class']
iris = iris[(iris['class'] == 'Iris-setosa') | (iris['class'] == 'Iris-versicolor')]
# 选择“花萼长度”和“花瓣长度”这两个特征,以及“山鸢尾”和“变色鸢尾”这两个类别
X = iris[['sepal_length', 'petal_length']]
y = iris['class']
y = np.where(y == 'Iris-setosa', 0, 1)
# 将数据集分为训练集和测试集
X_train = X[:-20]
y_train = y[:-20]
X_test = X[-20:]
y_test = y[-20:]
# 训练逻辑回归模型
lr = LogisticRegression(learning_rate=0.1, num_iterations=10000)
lr.fit(X_train, y_train)
# 测试模型
y_pred = lr.predict(X_test)
print('Predicted labels:', y_pred)
print('Accuracy:', lr.accuracy(y_test, y_pred))
# 可视化数据集和决策边界
plt.figure(figsize=(10, 6))
plt.scatter(X[y == 0]['sepal_length'], X[y == 0]['petal_length'], label='Iris-setosa')
plt.scatter(X[y == 1]['sepal_length'], X[y == 1]['petal_length'], label='Iris-versicolor')
x1_min, x1_max = X['sepal_length'].min(), X['sepal_length'].max(),
x2_min, x2_max = X['petal_length'].min(), X['petal_length'].max(),
xx1, xx2 = np.meshgrid(np.linspace(x1_min, x1_max), np.linspace(x2_min, x2_max))
grid = np.c_[xx1.ravel(), xx2.ravel()]
probs = sigmoid(np.dot(grid, lr.weights) + lr.bias).reshape(xx1.shape)
plt.contour(xx1, xx2, probs, [0.5], linewidths=1, colors='black')
plt.xlabel('Sepal length')
plt.ylabel('Petal length')
plt.legend()
plt.show()
```
运行上面的代码,即可得到决策函数的参数、预测值、分类准确率等输出结果,并且会显示出数据集和决策边界的散点图。
阅读全文
相关推荐
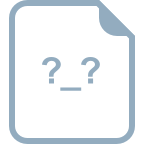
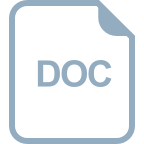
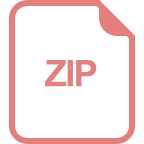














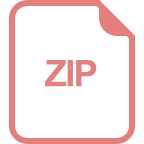