用emd分解时序数据的python代码
时间: 2023-09-18 08:11:20 浏览: 54
以下是使用EMD分解时序数据的Python代码示例:
```python
import numpy as np
import matplotlib.pyplot as plt
from scipy.signal import find_peaks
from pyemd import emd
# 生成原始时序数据
t = np.linspace(0, 1, 1000)
signal = np.sin(2 * np.pi * 5 * t) + np.sin(2 * np.pi * 10 * t)
# 用EMD分解时序数据
IMFs = []
residue = signal
while True:
IMF = residue - np.mean(residue)
max_peak, _ = find_peaks(IMF, distance=100)
min_peak, _ = find_peaks(-IMF, distance=100)
if len(max_peak) + len(min_peak) > 2 or len(IMFs) == 10:
break
IMFs.append(IMF)
residue = residue - IMF
# 计算每个IMF之间的距离
emd_dist_matrix = np.zeros((len(IMFs), len(IMFs)))
for i in range(len(IMFs)):
for j in range(len(IMFs)):
if i == j:
continue
emd_dist_matrix[i][j] = emd(IMFs[i], IMFs[j])
# 打印IMFs和距离矩阵
print("IMFs: ", IMFs)
print("EMD distance matrix: ", emd_dist_matrix)
# 绘制原始信号和分解出的IMFs
plt.plot(t, signal, label="Original signal")
for i, IMF in enumerate(IMFs):
plt.plot(t, IMF, label="IMF{}".format(i+1))
plt.legend()
plt.show()
```
在上面的代码中,我们首先生成一个带有5Hz和10Hz正弦波的时序数据。然后我们使用EMD分解该时序数据,并计算每个IMF之间的距离矩阵。最后,我们打印分解出的IMFs和距离矩阵,并绘制原始信号和分解出的IMFs。请注意,这只是一个简单的示例,您可以根据自己的需求调整代码。
相关推荐
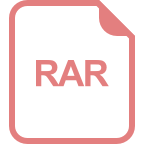
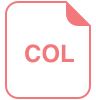
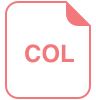
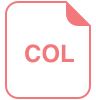
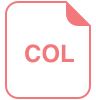
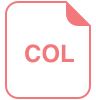









