DataFrame.to_dict() 方法代码实例、
时间: 2024-05-02 07:19:48 浏览: 14
可以通过以下代码将 DataFrame 转换为字典:
```
import pandas as pd
# 创建 DataFrame
df = pd.DataFrame({'col1': [1, 2, 3], 'col2': ['A', 'B', 'C']})
# 将 DataFrame 转换为字典
df_dict = df.to_dict()
print(df_dict)
```
输出结果如下:
```
{'col1': {0: 1, 1: 2, 2: 3}, 'col2': {0: 'A', 1: 'B', 2: 'C'}}
```
需要注意的是,to_dict() 方法默认返回的字典格式为列名为键,每列数据为值的嵌套字典。如果要将行名也包括在字典中,可以使用 orient='index' 参数:
```
df_dict = df.to_dict(orient='index')
print(df_dict)
```
输出结果如下:
```
{0: {'col1': 1, 'col2': 'A'}, 1: {'col1': 2, 'col2': 'B'}, 2: {'col1': 3, 'col2': 'C'}}
```
希望可以解决您的问题。
相关问题
def get_all_file_info(cls, folder_id, df, ft): print(folder_id) cls.params["folderId"] = folder_id res = requests.get(url=cls.url, params=cls.params) js_data = json.loads(res.text)["data"] js_data_FoldersInfo: list[dict] = js_data["FoldersInfo"] js_data_FilesInfo: list[dict] = js_data["FilesInfo"] df = pd.concat([pd.DataFrame(js_data_FilesInfo), df], axis=0) ft = pd.concat([pd.DataFrame(js_data_FoldersInfo), ft], axis=0) if not js_data_FoldersInfo: return df, ft for _folder_id in pd.DataFrame(js_data_FoldersInfo).FolderId: df, ft = cls.get_all_file_info(_folder_id, df, ft) return df, ft 优化这段代码
这段代码可能存在一些可优化的地方,以下是一些可能的建议:
1. 使用适当的命名规则和注释,以提高代码可读性。
2. 尽可能避免使用递归函数,因为递归消耗大量的内存和 CPU 资源,可能导致堆栈溢出等问题。可以尝试使用迭代方式处理。
3. 避免多次使用 pd.DataFrame(),可以将列表先转换为 DataFrame,然后再使用 pd.concat() 进行合并。
4. 尽可能使用列表推导式等 Pythonic 的方法进行数据处理,以提高代码效率和可读性。
下面是一种可能的优化方案:
```python
def get_all_file_info(cls, folder_id, df, ft):
"""
获取指定文件夹及其子文件夹中的所有文件和文件夹信息
:param cls: 类实例
:param folder_id: 文件夹 ID
:param df: 文件信息 DataFrame
:param ft: 文件夹信息 DataFrame
:return: 文件信息 DataFrame 和文件夹信息 DataFrame
"""
cls.params["folderId"] = folder_id
res = requests.get(url=cls.url, params=cls.params)
js_data = json.loads(res.text)["data"]
js_data_folders: list[dict] = js_data["FoldersInfo"]
js_data_files: list[dict] = js_data["FilesInfo"]
df = pd.concat([df, pd.DataFrame(js_data_files)], axis=0)
ft = pd.concat([ft, pd.DataFrame(js_data_folders)], axis=0)
folders_to_process = js_data_folders.copy()
while folders_to_process:
folder_id = folders_to_process.pop(0)["FolderId"]
cls.params["folderId"] = folder_id
res = requests.get(url=cls.url, params=cls.params)
js_data = json.loads(res.text)["data"]
js_data_folders: list[dict] = js_data["FoldersInfo"]
js_data_files: list[dict] = js_data["FilesInfo"]
df = pd.concat([df, pd.DataFrame(js_data_files)], axis=0)
ft = pd.concat([ft, pd.DataFrame(js_data_folders)], axis=0)
folders_to_process.extend(js_data_folders)
return df, ft
```
这个优化方案使用了一个 while 循环来遍历所有的文件夹,而不是使用递归函数。同时,使用了列表推导式和 copy() 方法来简化代码。
cls.params["folderId"] = folder_id res = requests.get(url=cls.url, params=cls.params) js_data = json.loads(res.text)["data"] js_data_folders: list[dict] = js_data["FoldersInfo"] js_data_files: list[dict] = js_data["FilesInfo"] df = pd.concat([df, pd.DataFrame(js_data_files)], axis=0) ft = pd.concat([ft, pd.DataFrame(js_data_folders)], axis=0) folders_to_process = js_data_folders.copy() while folders_to_process: folder_id = folders_to_process.pop(0)["FolderId"] cls.params["folderId"] = folder_id res = requests.get(url=cls.url, params=cls.params) js_data = json.loads(res.text)["data"] js_data_folders: list[dict] = js_data["FoldersInfo"] js_data_files: list[dict] = js_data["FilesInfo"] df = pd.concat([df, pd.DataFrame(js_data_files)], axis=0) ft = pd.concat([ft, pd.DataFrame(js_data_folders)], axis=0) folders_to_process.extend(js_data_folders) return df, ft 优化这段代码
这段代码已经进行了一些优化,但是还有一些可以改进的地方:
1. 需要确保请求返回的数据格式和结构是正确的,否则可能会导致代码出错。可以添加一些异常处理机制,例如使用 try-except 捕获异常并记录错误日志。
2. 对于返回的数据,可以使用 data.get("FoldersInfo", []) 和 data.get("FilesInfo", []) 来获取需要的信息,这样可以避免出现 Key Error 的问题。
3. 可以使用列表推导式来简化代码,例如使用 [x["FolderId"] for x in js_data_folders] 来获取所有文件夹的 ID。
下面是一个可能的优化方案:
```python
def get_all_file_info(cls, folder_id, df, ft):
"""
获取指定文件夹及其子文件夹中的所有文件和文件夹信息
:param cls: 类实例
:param folder_id: 文件夹 ID
:param df: 文件信息 DataFrame
:param ft: 文件夹信息 DataFrame
:return: 文件信息 DataFrame 和文件夹信息 DataFrame
"""
try:
cls.params["folderId"] = folder_id
res = requests.get(url=cls.url, params=cls.params)
res.raise_for_status()
data = json.loads(res.text)["data"]
except (requests.HTTPError, ValueError) as e:
logging.error(f"Failed to get file info for folder {folder_id}: {e}")
return df, ft
js_data_folders = data.get("FoldersInfo", [])
js_data_files = data.get("FilesInfo", [])
df = pd.concat([df, pd.DataFrame(js_data_files)], axis=0)
ft = pd.concat([ft, pd.DataFrame(js_data_folders)], axis=0)
folders_to_process = [x["FolderId"] for x in js_data_folders]
while folders_to_process:
folder_id = folders_to_process.pop(0)
try:
cls.params["folderId"] = folder_id
res = requests.get(url=cls.url, params=cls.params)
res.raise_for_status()
data = json.loads(res.text)["data"]
except (requests.HTTPError, ValueError) as e:
logging.error(f"Failed to get file info for folder {folder_id}: {e}")
continue
js_data_folders = data.get("FoldersInfo", [])
js_data_files = data.get("FilesInfo", [])
df = pd.concat([df, pd.DataFrame(js_data_files)], axis=0)
ft = pd.concat([ft, pd.DataFrame(js_data_folders)], axis=0)
folders_to_process.extend([x["FolderId"] for x in js_data_folders])
return df, ft
```
这个优化方案添加了异常处理机制,使用了 data.get() 方法来获取需要的信息,并使用了列表推导式来获取所有文件夹的 ID。如果请求出错,会记录错误日志并跳过当前文件夹的处理。
相关推荐
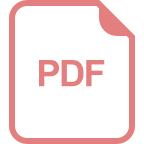
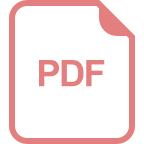
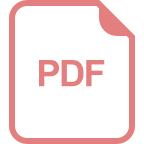












