用python写一个EOF分析
时间: 2023-07-20 07:41:42 浏览: 53
当处理文件时,EOF(End of File)是一个重要的概念。它表示输入流已经到达了文件的结尾。在Python中,我们可以使用文件对象的read()函数来读取文件中的所有内容,并通过检查是否返回一个空字符串来检测文件是否已经到达了结尾。
下面是一个简单的EOF分析的代码示例:
```python
with open('example.txt', 'r') as file:
content = file.read()
while content:
print(content, end='')
content = file.read()
```
在上面的代码中,我们创建了一个名为example.txt的文本文件,并使用open函数打开它。然后,我们使用while循环来读取文件中的内容,直到文件结束。在每次循环中,我们使用file.read()函数获取文件中的内容,如果返回一个空字符串,说明文件已经到达了结尾,退出循环。如果读取到了内容,我们使用print函数在屏幕上输出它。最后,我们使用with语句来自动关闭文件并释放资源。
相关问题
用python写一个语法分析器
语法分析器是计算机程序中的一部分,用于将输入的语言字符串解析成其语法结构。语法分析器可以通过递归下降法、LR分析等方式实现。
下面是一个简单的递归下降法语法分析器的示例,用于解析一个简单的算术表达式:
```
# 词法分析器,将字符串转换为tokens
class Lexer:
def __init__(self, text):
self.text = text
self.pos = 0
def error(self):
raise Exception("Invalid character")
def advance(self):
self.pos += 1
def get_token(self):
if self.pos >= len(self.text):
return Token(TokenType.EOF, None)
curr_char = self.text[self.pos]
if curr_char.isdigit():
value = 0
while self.pos < len(self.text) and self.text[self.pos].isdigit():
value = value * 10 + int(self.text[self.pos])
self.pos += 1
return Token(TokenType.INTEGER, value)
elif curr_char == '+':
self.advance()
return Token(TokenType.PLUS, '+')
elif curr_char == '-':
self.advance()
return Token(TokenType.MINUS, '-')
self.error()
# AST节点
class AST:
pass
class BinOp(AST):
def __init__(self, left, op, right):
self.left = left
self.token = self.op = op
self.right = right
class Num(AST):
def __init__(self, token):
self.token = token
self.value = token.value
# 解析器
class Parser:
def __init__(self, lexer):
self.lexer = lexer
self.current_token = self.lexer.get_token()
def error(self):
raise Exception("Invalid syntax")
def eat(self, token_type):
if self.current_token.type == token_type:
self.current_token = self.lexer.get_token()
else:
self.error()
def factor(self):
token = self.current_token
if token.type == TokenType.INTEGER:
self.eat(TokenType.INTEGER)
return Num(token)
elif token.type == TokenType.LPAREN:
self.eat(TokenType.LPAREN)
node = self.expr()
self.eat(TokenType.RPAREN)
return node
def term(self):
node = self.factor()
while self.current_token.type in (TokenType.MULTIPLY, TokenType.DIVIDE):
token = self.current_token
if token.type == TokenType.MULTIPLY:
self.eat(TokenType.MULTIPLY)
elif token.type == TokenType.DIVIDE:
self.eat(TokenType.DIVIDE)
node = BinOp(left=node, op=token, right=self.factor())
return node
def expr(self):
node = self.term()
while self.current_token.type in (TokenType.PLUS, TokenType.MINUS):
token = self.current_token
if token.type == TokenType.PLUS:
self.eat(TokenType.PLUS)
elif token.type == TokenType.MINUS:
self.eat(TokenType.MINUS)
node = BinOp(left=node, op=token, right=self.term())
return node
# Token类型
from enum import Enum
class TokenType(Enum):
INTEGER = 0
PLUS = 1
MINUS = 2
MULTIPLY = 3
DIVIDE = 4
LPAREN = 5
RPAREN = 6
EOF = 7
# Token对象
class Token:
def __init__(self, type, value):
self.type = type
self.value = value
def __str__(self):
return 'Token({type}, {value})'.format(
type=self.type,
value=repr(self.value)
)
def __repr__(self):
return self.__str__()
# 测试
def main():
while True:
try:
text = input('> ')
except EOFError:
break
if not text:
continue
lexer = Lexer(text)
parser = Parser(lexer)
result = parser.expr()
print(result)
if __name__ == '__main__':
main()
```
在上面的代码中,我们定义了一个Lexer类来将输入的字符串转换为tokens,定义了一个Parser类来将tokens解析成AST,最后通过递归下降法计算表达式的值。
测试代码:
```
> 2+3*4
BinOp(left=Num(Token(INTEGER, 2)), op=Token(PLUS, '+'), right=BinOp(left=Num(Token(INTEGER, 3)), op=Token(MULTIPLY, '*'), right=Num(Token(INTEGER, 4))))
> (2+3)*4
BinOp(left=BinOp(left=Num(Token(INTEGER, 2)), op=Token(PLUS, '+'), right=Num(Token(INTEGER, 3))), op=Token(MULTIPLY, '*'), right=Num(Token(INTEGER, 4)))
```
eof分解python
EOF分解(Empirical Orthogonal Function decomposition)是一种主成分分析方法,用于分解多维数据集中的空间和时间变化模态。在气象和海洋学中,EOF分解常用于分析海表面温度、气压等大气和海洋场的变化模态。
以下是使用Python进行EOF分解的示例代码:
```python
import numpy as np
from scipy.linalg import svd
# 假设有一个二维数据集,shape为 (n, m),其中 n 是时间步数,m 是空间点数
data = np.random.rand(n, m)
# 计算数据集的空间平均值
mean = np.mean(data, axis=0)
# 将数据集减去空间平均值,得到去平均后的数据集
data_anomaly = data - mean
# 计算数据集的协方差矩阵
covariance_matrix = np.cov(data_anomaly.T)
# 对协方差矩阵进行奇异值分解
U, s, V = svd(covariance_matrix)
# 提取前 k 个模态
k = 3
modes = U[:, :k]
# 计算每个时间步的时间系数
time_coefficients = np.dot(data_anomaly, modes)
# 合成前 k 个模态
reconstructed_data = np.dot(time_coefficients, modes.T) + mean
# 打印结果
print("EOF modes:")
print(modes)
print("Time coefficients:")
print(time_coefficients)
print("Reconstructed data:")
print(reconstructed_data)
```
这段代码首先对数据集进行了去平均处理,然后计算了数据集的协方差矩阵,并对其进行了奇异值分解。接着,根据指定的模态数量 k,提取了前 k 个模态,并计算了每个时间步的时间系数。最后,根据时间系数和模态,合成了重构数据集。
相关推荐
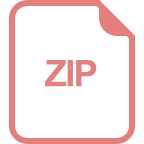
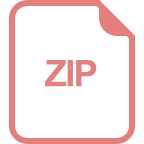












