bp神经网络Tensorflow的作用
时间: 2023-11-16 19:38:24 浏览: 73
BP神经网络是一种常见的神经网络模型,它常用于分类、回归和预测等任务。TensorFlow是一种流行的机器学习框架,它提供了构建和训练BP神经网络的工具和函数。
TensorFlow的BP神经网络模块提供了各种不同的层类型、优化器、损失函数和评估指标,可以用于构建各种不同的神经网络模型。使用TensorFlow的BP神经网络模块,可以轻松地构建、训练和评估BP神经网络,从而完成各种不同的机器学习任务。
相关问题
bp神经网络TensorFlow
BP神经网络是一种常见的人工神经网络,用于解决分类、回归等问题。TensorFlow是一个用于构建和训练机器学习模型的开源框架,其中包括了BP神经网络的实现。
在TensorFlow中,可以使用tf.keras.Sequential来创建一个简单的BP神经网络模型。例如,以下代码创建了一个包含两个隐藏层的BP神经网络:
```
import tensorflow as tf
from tensorflow import keras
model = keras.Sequential([
keras.layers.Dense(64, activation='relu', input_shape=(784,)),
keras.layers.Dense(64, activation='relu'),
keras.layers.Dense(10, activation='softmax')
])
```
其中,第一层和第二层都是全连接层,每个层包含64个神经元,并使用ReLU作为激活函数。第三层是输出层,包含10个神经元,使用softmax激活函数。
接下来,可以使用compile方法来配置模型的训练过程,并使用fit方法来训练模型:
```
model.compile(optimizer=tf.train.AdamOptimizer(0.001),
loss='categorical_crossentropy',
metrics=['accuracy'])
model.fit(train_images, train_labels, epochs=5, batch_size=32)
```
其中,使用Adam优化器和交叉熵损失函数来训练模型,并使用准确率作为评估指标。训练数据可以通过train_images和train_labels来提供,epochs参数指定训练轮数,batch_size参数指定每个批次的样本数量。
bp神经网络tensorflow代码
以下是一个基本的BP神经网络的TensorFlow代码示例:
```
import tensorflow as tf
# 定义输入和输出数据
x_data = [[0,0], [0,1], [1,0], [1,1]]
y_data = [[0], [1], [1], [0]]
# 定义输入和输出占位符
x = tf.placeholder(tf.float32, shape=[None, 2])
y = tf.placeholder(tf.float32, shape=[None, 1])
# 定义神经网络模型
W1 = tf.Variable(tf.random_normal([2, 2]), name='weight1')
b1 = tf.Variable(tf.random_normal([2]), name='bias1')
layer1 = tf.sigmoid(tf.matmul(x, W1) + b1)
W2 = tf.Variable(tf.random_normal([2, 1]), name='weight2')
b2 = tf.Variable(tf.random_normal([1]), name='bias2')
hypothesis = tf.sigmoid(tf.matmul(layer1, W2) + b2)
# 定义损失函数和优化器
cost = -tf.reduce_mean(y * tf.log(hypothesis) + (1 - y) * tf.log(1 - hypothesis))
train = tf.train.GradientDescentOptimizer(learning_rate=0.1).minimize(cost)
# 启动会话并运行优化器
with tf.Session() as sess:
sess.run(tf.global_variables_initializer())
for step in range(10001):
_, cost_val = sess.run([train, cost], feed_dict={x: x_data, y: y_data})
if step % 1000 == 0:
print(step, cost_val)
# 训练结束后预测结果
predicted = tf.cast(hypothesis > 0.5, dtype=tf.float32)
accuracy = tf.reduce_mean(tf.cast(tf.equal(predicted, y), dtype=tf.float32))
h, c, a = sess.run([hypothesis, predicted, accuracy], feed_dict={x: x_data, y: y_data})
print("\nHypothesis: ", h, "\nCorrect: ", c, "\nAccuracy: ", a)
```
在这个例子中,我们使用了一个具有两个隐藏层的BP神经网络,输入是两个数字,输出是一个数字。我们使用了sigmoid函数作为激活函数,使用交叉熵作为损失函数,使用梯度下降优化器进行优化。在进行预测时,我们将预测结果转换为0或1,并计算准确率。
阅读全文
相关推荐
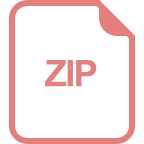











