Recent color transfer methods use local information to learn the transformation from a source to an exemplar image, and then transfer this appearance change to a target image. These solutions achieve very successful results for general mood changes, e.g., changing the appearance of an image from “sunny” to “overcast”. However, such methods have a hard time creating new image content, such as leaves on a bare tree. Texture transfer, on the other hand, can synthesize such content but tends to destroy image structure. We propose the first algorithm that unifies color and texture transfer, outperforming both by leveraging their respective strengths. A key novelty in our approach resides in teasing apart appearance changes that can be modeled simply as changes in color versus those that require new image content to be generated. Our method starts with an analysis phase which evaluates the success of color transfer by comparing the exemplar with the source. This analysis then drives a selective, iterative texture transfer algorithm that simultaneously predicts the success of color transfer on the target and synthesizes new content where needed. We demonstrate our unified algorithm by transferring large temporal changes between photographs, such as change of season – e.g., leaves on bare trees or piles of snow on a street – and flooding.翻译为中文
时间: 2024-02-14 11:22:24 浏览: 19
最近的颜色转换方法利用局部信息来学习从源图像到示例图像的转换,并将这种外观变化传递到目标图像。这些解决方案在一般情况下取得了非常成功的结果,例如将图像的外观从“晴朗”变为“阴天”。然而,这样的方法很难创建新的图像内容,例如裸树上的树叶。另一方面,纹理转换可以合成这样的内容,但往往会破坏图像结构。我们提出了第一个统一颜色和纹理转换的算法,通过利用它们各自的优势,优于两者。我们方法的一个关键创新在于将可以简单地建模为颜色变化的外观变化与需要生成新图像内容的变化区分开来。我们的方法始于一个分析阶段,通过比较示例和源来评估颜色转换的成功。然后,这个分析驱动一个有选择性的、迭代的纹理转换算法,同时预测颜色转换在目标上的成功,并在需要时合成新的内容。我们通过将大的时间变化,例如季节的变化(例如树上的叶子或街道上的积雪)和洪水转移,来展示我们的统一算法。
相关问题
how to parse a complex, densely nested object graph into a more straightforward model for use in another domain.
To parse a complex, densely nested object graph into a more straightforward model for use in another domain, you can use a technique called "object mapping" or "object transformation". This involves mapping the complex object graph to a simpler model that is more appropriate for the other domain.
Here are the general steps for object mapping:
1. Define the target model: Define the simpler model that you want to map to. This model should have only the properties that are relevant to the other domain.
2. Define the mapping rules: Define the rules for mapping the properties from the complex object graph to the properties in the target model. This may involve combining or filtering properties, renaming properties, or converting data types.
3. Implement the mapping: Implement the mapping rules using a mapping library or manually. One popular mapping library for Java is MapStruct, but there are many others available.
Here's an example of object mapping using MapStruct:
Suppose you have a complex object graph representing a library with many books, authors, and publishers. You want to map this to a simpler model that only includes the title, author name, and publisher name for each book.
1. Define the target model:
```
public class BookInfo {
private String title;
private String authorName;
private String publisherName;
// getters and setters
}
```
2. Define the mapping rules:
```
@Mapper
public interface LibraryMapper {
@Mapping(source = "book.title", target = "title")
@Mapping(source = "book.author.name", target = "authorName")
@Mapping(source = "book.publisher.name", target = "publisherName")
BookInfo mapBookToBookInfo(Book book);
}
```
3. Implement the mapping:
```
LibraryMapper mapper = Mappers.getMapper(LibraryMapper.class);
List<BookInfo> bookInfos = library.getBooks().stream()
.map(mapper::mapBookToBookInfo)
.collect(Collectors.toList());
```
This will map the complex object graph to a simpler model that is more appropriate for use in another domain.
kettle 报以下错误的原因:No cluster schemas are being used. As such it is not possible to split and cluster this transformation.
Kettle的集群模式需要在集群模式下进行配置和执行,而该错误通常是因为在转换中没有使用集群模式相关的配置,导致无法进行拆分和集群操作。可能出现这种情况的原因有:
1. 没有使用正确的集群模式连接配置。在Kettle中,需要使用Cluster schema连接来与集群模式进行通信,如果没有使用Cluster schema连接,就无法在集群模式下运行转换。
2. 没有正确配置转换步骤的并行度。在集群模式下,需要将转换中的步骤进行并行化配置,以便在集群中进行分布式执行。如果没有正确配置步骤的并行度,就无法进行集群分布式执行。
3. 转换中没有使用支持集群模式的步骤。Kettle中的一些步骤不支持集群模式,例如本地文件读写,如果在转换中使用了这些步骤,就无法进行集群模式的拆分和执行。
解决方法是检查转换中的配置是否正确,包括连接、步骤的并行度和使用的步骤是否支持集群模式。如果以上都正确,但仍出现该错误,则可能是Kettle版本或集群环境的问题,需要进行更深入的排查和调试。
相关推荐
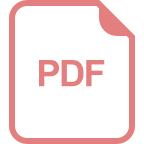
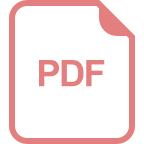












