编写一个程序,采用 scikit-learn 中的 LogisticRegression 逻辑回归模型对 iris 数据集进行多分类,并将结果可视化
时间: 2024-02-20 10:57:41 浏览: 89
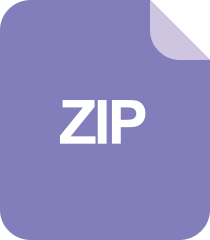
python实现决策树莺尾花(代码+数据集) Jupyter Notebook打开.ipynb文件
好的,下面是使用 scikit-learn 中的 LogisticRegression 模型对 iris 数据集进行多分类,并将结果可视化的代码:
```python
import numpy as np
import matplotlib.pyplot as plt
from sklearn.datasets import load_iris
from sklearn.linear_model import LogisticRegression
from sklearn.model_selection import train_test_split
from sklearn.metrics import classification_report
# 加载 iris 数据集
iris = load_iris()
X = iris.data
y = iris.target
# 划分训练集和测试集
X_train, X_test, y_train, y_test = train_test_split(X, y, test_size=0.3, random_state=0)
# 建立逻辑回归模型
clf = LogisticRegression(multi_class='multinomial', solver='lbfgs', max_iter=1000)
clf.fit(X_train, y_train)
# 预测测试集的类别
y_pred = clf.predict(X_test)
# 输出分类报告
print(classification_report(y_test, y_pred))
# 可视化结果
x_min, x_max = X[:, 0].min() - 0.5, X[:, 0].max() + 0.5
y_min, y_max = X[:, 1].min() - 0.5, X[:, 1].max() + 0.5
xx, yy = np.meshgrid(np.arange(x_min, x_max, 0.02), np.arange(y_min, y_max, 0.02))
Z = clf.predict(np.c_[xx.ravel(), yy.ravel()])
# Put the result into a color plot
Z = Z.reshape(xx.shape)
plt.figure(1, figsize=(8, 6))
plt.pcolormesh(xx, yy, Z, cmap=plt.cm.Paired)
# Plot also the training points
plt.scatter(X[:, 0], X[:, 1], c=y, edgecolors='k', cmap=plt.cm.Paired)
plt.xlabel('Sepal length')
plt.ylabel('Sepal width')
plt.xlim(xx.min(), xx.max())
plt.ylim(yy.min(), yy.max())
plt.xticks(())
plt.yticks(())
plt.show()
```
这段代码会输出分类报告,并将结果可视化。可视化结果以 Sepal length 和 Sepal width 为坐标轴,用不同颜色的点表示不同类别的数据,用背景色表示每个点的预测类别。您可以根据需要进行修改和调整。
阅读全文
相关推荐
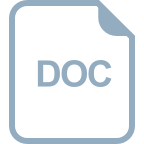
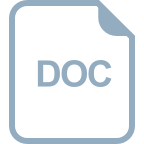











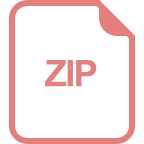
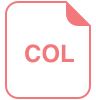
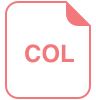

