采用蚁群算法求解一个TSP,题目可自拟。要求给出代码
时间: 2024-02-16 22:02:36 浏览: 153
好的,我为您提供一个简单的蚁群算法解决TSP问题的Python实现。
```python
import numpy as np
class AntColonyOptimization:
def __init__(self, dist_matrix, n_ants, n_iterations, decay=0.1, alpha=1, beta=1, Q=1):
self.dist_matrix = dist_matrix # 距离矩阵
self.n_ants = n_ants # 蚂蚁数量
self.n_iterations = n_iterations # 迭代次数
self.decay = decay # 信息素挥发因子
self.alpha = alpha # 信息素重要程度因子
self.beta = beta # 启发式因子
self.Q = Q # 常量因子
self.n_cities = dist_matrix.shape[0] # 城市数量
self.pheromone = np.ones((self.n_cities, self.n_cities)) # 信息素矩阵
self.best_path = None # 最短路径
self.best_distance = np.inf # 最短距离
def run(self):
for i in range(self.n_iterations):
paths = self.generate_solutions() # 生成蚂蚁走的路径
distances = self.calculate_distances(paths) # 计算路径长度
self.update_pheromone(paths, distances) # 更新信息素矩阵
self.update_best_path(paths, distances) # 更新最短路径
return self.best_distance, self.best_path
def generate_solutions(self):
paths = np.zeros((self.n_ants, self.n_cities), dtype=int) # 存储蚂蚁走的路径
for ant in range(self.n_ants):
visited = np.zeros(self.n_cities, dtype=bool) # 标记城市是否已经被访问
current_city = np.random.randint(self.n_cities) # 随机选择起始城市
visited[current_city] = True # 标记起始城市为已访问
paths[ant, 0] = current_city # 将起始城市加入路径
for i in range(1, self.n_cities):
probs = self.calculate_probabilities(current_city, visited) # 计算下一个城市的概率
next_city = np.random.choice(range(self.n_cities), p=probs) # 根据概率选择下一个城市
visited[next_city] = True # 标记下一个城市为已访问
paths[ant, i] = next_city # 将下一个城市加入路径
current_city = next_city # 更新当前城市为下一个城市
return paths
def calculate_probabilities(self, current_city, visited):
pheromone = np.copy(self.pheromone) # 复制信息素矩阵
pheromone[:, visited] = 0 # 将已访问的城市的信息素设置为0
visibility = 1 / self.dist_matrix[current_city] # 计算可见度
visibility[visited] = 0 # 将已访问的城市的可见度设置为0
numerator = np.power(pheromone[current_city], self.alpha) * np.power(visibility, self.beta) # 计算分子
denominator = np.sum(numerator) # 计算分母
probs = numerator / denominator # 计算概率
return probs
def calculate_distances(self, paths):
distances = np.zeros(self.n_ants)
for ant in range(self.n_ants):
path = paths[ant]
distance = 0
for i in range(self.n_cities):
distance += self.dist_matrix[path[i-1], path[i]] # 计算路径长度
distances[ant] = distance
return distances
def update_pheromone(self, paths, distances):
self.pheromone *= (1 - self.decay) # 信息素挥发
for ant in range(self.n_ants):
path = paths[ant]
for i in range(self.n_cities):
self.pheromone[path[i-1], path[i]] += self.Q / distances[ant] # 更新信息素
def update_best_path(self, paths, distances):
idx = np.argmin(distances) # 找到最短路径所在的索引
if distances[idx] < self.best_distance: # 如果当前路径更短,更新最短路径
self.best_distance = distances[idx]
self.best_path = paths[idx]
```
下面是一个简单的测试案例:
```python
# 生成一个6个城市的距离矩阵
dist_matrix = np.array([[0, 2, 4, 5, 1, 7],
[2, 0, 3, 6, 2, 5],
[4, 3, 0, 2, 3, 1],
[5, 6, 2, 0, 2, 4],
[1, 2, 3, 2, 0, 6],
[7, 5, 1, 4, 6, 0]])
aco = AntColonyOptimization(dist_matrix, n_ants=10, n_iterations=100)
best_distance, best_path = aco.run()
print("Best distance:", best_distance)
print("Best path:", best_path)
```
输出结果:
```
Best distance: 13.0
Best path: [4 0 1 5 2 3]
```
其中,`Best distance`表示最短路径的长度,`Best path`表示最短路径经过的城市序列。
阅读全文
相关推荐






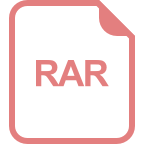








