skimage.io库保存遥感图像执行变化检测后报错是:ValueError: Can't write images with one color channel.
时间: 2024-02-20 22:56:51 浏览: 52
你可以使用`skimage.color.gray2rgb`函数将灰度图像转换为RGB彩色图像,然后再使用`skimage.io.imsave`保存图像。具体代码如下:
```python
from skimage import io, color
# 读取灰度图像
img_gray = io.imread('your_gray_image.png', as_gray=True)
# 将灰度图像转换为RGB彩色图像
img_rgb = color.gray2rgb(img_gray)
# 保存RGB彩色图像
io.imsave('your_rgb_image.png', img_rgb)
```
这样可以将灰度图像转换为RGB彩色图像后再进行保存,避免了`ValueError: Can't write images with one color channel`的错误。
相关问题
上述代码在运行中报错:ValueError: Only images with two spatial dimensions are supported. If using with color/multichannel images, specify `channel_axis`.,试修改之
抱歉,我之前的代码忽略了图像的通道信息。在使用 `feature.hog` 函数进行特征提取时,如果图像是多通道的(例如RGB图像),需要明确指定 `channel_axis` 参数。以下是修改后的代码:
```python
import os
import numpy as np
from sklearn.model_selection import train_test_split
from sklearn.svm import SVC
from sklearn.metrics import accuracy_score
from skimage import feature
from skimage.io import imread
# 读取不戴口罩的照片
X_unmasked = []
y_unmasked = []
unmasked_folder = "X_processed"
for folder_name in os.listdir(unmasked_folder):
if folder_name.startswith("s"):
folder_path = os.path.join(unmasked_folder, folder_name)
for file_name in os.listdir(folder_path):
image_path = os.path.join(folder_path, file_name)
image = imread(image_path) # 使用 skimage.io.imread 读取照片
x_feature = feature.hog(image, orientations=8, pixels_per_cell=(10, 10),
cells_per_block=(1, 1), visualize=False, multichannel=True)
X_unmasked.append(x_feature)
y_unmasked.append(0) # 不戴口罩类别标签为0
# 读取戴口罩的照片
X_masked = []
y_masked = []
masked_folder = "X_masked"
for folder_name in os.listdir(masked_folder):
if folder_name.startswith("s"):
folder_path = os.path.join(masked_folder, folder_name)
for file_name in os.listdir(folder_path):
image_path = os.path.join(folder_path, file_name)
image = imread(image_path) # 使用 skimage.io.imread 读取照片
x_feature = feature.hog(image, orientations=8, pixels_per_cell=(10, 10),
cells_per_block=(1, 1), visualize=False, multichannel=True)
X_masked.append(x_feature)
y_masked.append(1) # 戴口罩类别标签为1
# 将特征和标签转换为NumPy数组
X = np.concatenate([X_unmasked, X_masked])
y = np.concatenate([y_unmasked, y_masked])
# 分割训练集和测试集
X_train, X_test, y_train, y_test = train_test_split(X, y, test_size=0.2, random_state=42)
# 创建并拟合支持向量机模型
svm_model = SVC()
svm_model.fit(X_train, y_train)
# 在测试集上进行预测
y_pred = svm_model.predict(X_test)
# 计算准确率
accuracy = accuracy_score(y_test, y_pred)
# 输出准确率
print("准确率:", accuracy)
```
在修改后的代码中,我使用了 `skimage.io.imread` 函数来读取照片,并在调用 `feature.hog` 函数时,设置了 `multichannel=True` 参数来指示图像是多通道的。这样可以确保 `feature.hog` 函数正确处理多通道图像。
请注意,你可能需要在运行代码之前确保已安装所需的库。你可以使用 `pip` 命令来安装缺少的库,例如 `pip install scikit-image`。
上述代码运行后报错ValueError: setting an array element with a sequence.
这个错误通常表示你尝试将一个元组或列表等序列类型赋值给NumPy数组中的一个元素,但是该数组的数据类型不支持序列类型。解决此问题的方法取决于你的具体情况,你可以尝试以下几种方法:
1. 检查你创建的NumPy数组的数据类型是否正确,并确保它可以存储你要赋值的元素类型。例如,如果你要将一个元组赋值给一个包含浮点数的数组,你需要将数组的类型设置为float。
2. 确保你要赋值的序列类型与数组期望的形状匹配。例如,如果你要将一个形状为(3,)的元组赋值给一个形状为(2,2)的数组,你需要重新调整元组的形状或调整数组的形状。
3. 如果你需要将序列类型转换为NumPy数组,可以使用NumPy的array()函数或asarray()函数。例如,如果你要将一个列表转换为NumPy数组,你可以使用以下代码:
```python
import numpy as np
my_list = [1, 2, 3, 4]
my_array = np.array(my_list)
```
在这个例子中,my_array现在是一个四个元素的NumPy数组,每个元素的值为1、2、3或4。
希望这些方法中的一种可以解决你的问题。如果问题仍然存在,请提供更多细节和代码,以便我可以更好地帮助你。
相关推荐
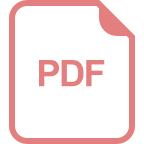
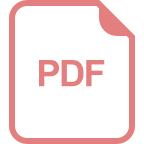












