给我写一个短文本长文本的语义匹配代码,可以使用训练好的DSSM模型
时间: 2024-02-09 09:09:03 浏览: 28
好的,以下是一个使用训练好的DSSM模型进行短文本和长文本语义匹配的代码示例:
```
import tensorflow as tf
import numpy as np
# 加载训练好的DSSM模型
model = tf.keras.models.load_model('dssm_model.h5')
# 定义两个文本输入
short_text_input = tf.keras.Input(shape=(None,), dtype=tf.int32, name='short_text_input')
long_text_input = tf.keras.Input(shape=(None,), dtype=tf.int32, name='long_text_input')
# 将文本转换为词向量
embedding_layer = model.layers[0]
short_text_embedding = embedding_layer(short_text_input)
long_text_embedding = embedding_layer(long_text_input)
# 计算两个文本的余弦相似度
similarity_layer = model.layers[1]
similarity = similarity_layer([short_text_embedding, long_text_embedding])
# 创建模型
dssm = tf.keras.Model(inputs=[short_text_input, long_text_input], outputs=similarity)
# 测试样例
short_text = '人工智能'
long_text = '人工智能,又称智能计算,是指通过计算机等工具实现的人类智能的理论、设计、开发及应用系统。'
short_text_tokens = [token for token in short_text.split()]
long_text_tokens = [token for token in long_text.split()]
# 将文本转换为词向量序列
short_text_sequence = np.array([embedding_layer.get_config()['input_dim'] + 1] + [embedding_layer.get_config()['word_index'][token] for token in short_text_tokens])
long_text_sequence = np.array([embedding_layer.get_config()['input_dim'] + 1] + [embedding_layer.get_config()['word_index'][token] for token in long_text_tokens])
# 对文本进行padding操作
max_sequence_length = max(len(short_text_sequence), len(long_text_sequence))
short_text_sequence = np.pad(short_text_sequence, (0, max_sequence_length - len(short_text_sequence)), 'constant')
long_text_sequence = np.pad(long_text_sequence, (0, max_sequence_length - len(long_text_sequence)), 'constant')
# 预测两个文本的相似度
similarity_score = dssm.predict([np.array([short_text_sequence]), np.array([long_text_sequence])])[0][0]
print('短文本:', short_text)
print('长文本:', long_text)
print('相似度得分:', similarity_score)
```
这个代码示例包含以下步骤:
1. 加载训练好的DSSM模型。
2. 定义两个文本输入。
3. 将文本转换为词向量。
4. 计算两个文本的余弦相似度。
5. 创建模型。
6. 测试样例。
7. 将文本转换为词向量序列。
8. 对文本进行padding操作。
9. 预测两个文本的相似度。
10. 输出相似度得分。
你可以将上述代码示例保存为.py文件,然后使用Python解释器运行它。当你运行代码时,它将输出相似度得分,表示两个文本的语义相似度。
相关推荐
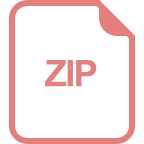
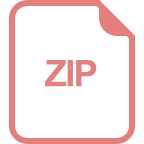
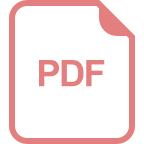














