test_mean_max = np.max(test_mean) test_mean_min = np.min(test_mean) bins = 10 fig = plt.figure(figsize=(8, 6)) ax = plt.subplot(111) ax.hist(test_mean, bins=bins, range=(test_mean_min, test_mean_max), density=True, rwidth=0.9) mu, sigma = test_mean.mean(), test_mean.std() x = np.linspace(test_mean_min, test_mean_max, 100000) y = 1 / (sigma*np.sqrt(2*np.pi)) * np.exp(- (x-mu)**2 / (2*sigma**2)) ax.plot(x, y) plt.show()为什么已经归一化,y轴的值有大于1的值
时间: 2024-04-29 17:19:28 浏览: 136
在这段代码中,`density=True`参数会将直方图的值归一化为概率密度,即每个箱子内的计数除以箱子宽度和所有计数的总和。因此,直方图的y轴表示的是概率密度,不是计数。但是,代码中添加的曲线是正态分布的概率密度函数,它的最大值是1/sqrt(2*pi*sigma^2),也就是说,它的最大值并不是1。因此,即使直方图被归一化为概率密度,曲线的最大值仍然可以大于1。
相关问题
def aug(src): """图像亮度增强""" if get_lightness(src) > 130: print("图片亮度足够,不做增强") # 先计算分位点,去掉像素值中少数异常值,这个分位点可以自己配置。 # 比如1中直方图的红色在0到255上都有值,但是实际上像素值主要在0到20内。 max_percentile_pixel, min_percentile_pixel = compute(src, 1, 99) # 去掉分位值区间之外的值 src[src >= max_percentile_pixel] = max_percentile_pixel src[src <= min_percentile_pixel] = min_percentile_pixel # 将分位值区间拉伸到0到255,这里取了2550.1与2550.9是因为可能会出现像素值溢出的情况,所以最好不要设置为0到255。 out = np.zeros(src.shape, src.dtype) cv2.normalize(src, out, 255 * 0.1, 255 * 0.9, cv2.NORM_MINMAX) return out 这个代码如何修改为单个文件代码对图片进行图像增强
你可以将这段代码整合到一个单独的Python文件中,然后添加读取图片和保存图片的代码,以实现对单个图片的图像增强。修改后的代码如下:
```
import cv2
import numpy as np
def get_lightness(src):
"""计算图像亮度"""
hsv = cv2.cvtColor(src, cv2.COLOR_BGR2HSV)
h, s, v = cv2.split(hsv)
return np.mean(v)
def compute(src, low_percentile, high_percentile):
"""计算分位点"""
hist, bins = np.histogram(src.flatten(), 256, [0, 256])
cdf = hist.cumsum()
cdf_normalized = cdf / cdf.max()
low_limit = np.percentile(cdf_normalized, low_percentile)
high_limit = np.percentile(cdf_normalized, high_percentile)
min_gray = np.argwhere(cdf_normalized >= low_limit)[0]
max_gray = np.argwhere(cdf_normalized <= high_limit)[-1]
return max_gray, min_gray
def aug(src_path, dst_path):
"""图像亮度增强"""
src = cv2.imread(src_path)
if get_lightness(src) > 130:
print("图片亮度足够,不做增强")
return
# 先计算分位点,去掉像素值中少数异常值,这个分位点可以自己配置。
# 比如1中直方图的红色在0到255上都有值,但是实际上像素值主要在0到20内。
max_percentile_pixel, min_percentile_pixel = compute(src, 1, 99)
# 去掉分位值区间之外的值
src[src >= max_percentile_pixel] = max_percentile_pixel
src[src <= min_percentile_pixel] = min_percentile_pixel
# 将分位值区间拉伸到0到255,这里取了255*0.1与255*0.9是因为可能会出现像素值溢出的情况,所以最好不要设置为0到255。
out = np.zeros(src.shape, src.dtype)
cv2.normalize(src, out, 255 * 0.1, 255 * 0.9, cv2.NORM_MINMAX)
# 保存增强后的图片
cv2.imwrite(dst_path, out)
# 测试
img_path = 'test.jpg'
aug(img_path, 'test_aug.jpg')
```
在测试代码中,`img_path`是原始图片路径,'test_aug.jpg'是增强后的图片路径。注意,这里只对单张图片进行增强,如果你需要对整个文件夹中的图片进行增强,需要使用`os`模块来批量读取和保存图片。
Daily foreign exchange rates (spot rates) can be obtained from the Federal Reserve Bank in St Louis (FRED). The data are the noon buying rates in New York City certified by the Federal Reserve Bank of New York. Consider the exchange rates between the U.S. dollar and the Euro from January 4, 1999 to March 8, 2013. See the file d-exuseu.txt. (a) Compute the daily log return of the exchange rate. (b) Compute the sample mean, standard deviation, skewness, excess kurtosis, minimum, and maximum of the log returns of the exchange rate. (c) Obtain a density plot of the daily long returns of Dollar-Euro exchange rate. (d) Test H0 : µ = 0 versus Ha : µ ̸= 0, where µ denotes the mean of the daily log return of Dollar-Euro exchange rate.
(a) The daily log return of the exchange rate can be calculated using the following formula:
log return = ln(price[t]) - ln(price[t-1])
where price[t] represents the exchange rate at time t and price[t-1] represents the exchange rate at time t-1.
Using the data in the file d-exuseu.txt, we can calculate the daily log returns as follows (assuming the data is stored in a variable called "exchange_rate"):
```python
import numpy as np
log_returns = np.log(exchange_rate[1:]) - np.log(exchange_rate[:-1])
```
The first element of "exchange_rate" is excluded from the calculation because there is no previous price to compare it to.
(b) The sample mean, standard deviation, skewness, excess kurtosis, minimum, and maximum of the log returns can be calculated using the following code:
```python
mean = np.mean(log_returns)
std_dev = np.std(log_returns)
skewness = stats.skew(log_returns)
kurtosis = stats.kurtosis(log_returns, fisher=False)
minimum = np.min(log_returns)
maximum = np.max(log_returns)
print("Sample mean:", mean)
print("Standard deviation:", std_dev)
print("Skewness:", skewness)
print("Excess kurtosis:", kurtosis - 3) # convert to excess kurtosis
print("Minimum:", minimum)
print("Maximum:", maximum)
```
This code requires the "scipy.stats" module to be imported at the beginning of the script. The output will show the sample mean, standard deviation, skewness, excess kurtosis, minimum, and maximum of the log returns.
(c) To obtain a density plot of the daily log returns, we can use the following code:
```python
import matplotlib.pyplot as plt
plt.hist(log_returns, bins=50, density=True)
plt.xlabel("Daily log return")
plt.ylabel("Density")
plt.show()
```
This code will create a histogram of the log returns with 50 bins and normalize it to create a density plot. The output will show the density plot of the log returns.
(d) To test the hypothesis H0 : µ = 0 versus Ha : µ ̸= 0, where µ denotes the mean of the daily log return of Dollar-Euro exchange rate, we can use a t-test. The null hypothesis states that the mean log return is equal to zero, while the alternative hypothesis states that the mean log return is not equal to zero.
```python
from scipy.stats import ttest_1samp
t_stat, p_value = ttest_1samp(log_returns, 0)
print("t-statistic:", t_stat)
print("p-value:", p_value)
```
This code uses the "ttest_1samp" function from the "scipy.stats" module to calculate the t-statistic and the p-value. The output will show the t-statistic and the p-value of the test. If the p-value is less than the significance level (e.g., 0.05), we can reject the null hypothesis and conclude that the mean log return is significantly different from zero.
阅读全文
相关推荐
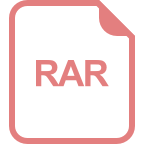
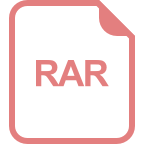
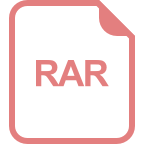
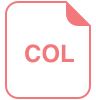
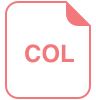
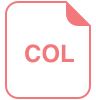
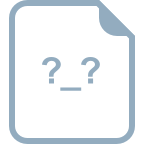
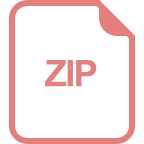
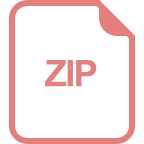
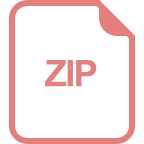
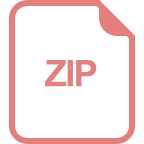
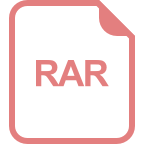
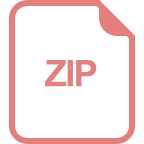
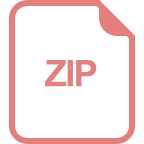