> missing_idx <- which(!complete.cases(data)) > missing_idx [1] 69 > data <- na.omit(data) > c1<-with(data,kruskal(Fquantity,deal1:deal2:deal3,group=TRUE, main="a")) Error in deal1:deal2 : NA/NaN argument In addition: Warning messages: 1: In deal1:deal2 : numerical expression has 84 elements: only the first used 2: In deal1:deal2 : numerical expression has 84 elements: only the first used 3: In data.frame(y, trt) : NAs introduced by coercion 4: In data.frame(y, trt) : NAs introduced by coercion
时间: 2024-03-12 18:43:37 浏览: 132
根据你提供的代码和错误信息,出现了两个问题:
1. `missing_idx [1] 69`:`which(!complete.cases(data))` 返回的是包含缺失值的行的索引号,而这里的结果是69,说明第69行包含缺失值。你需要查看这个行的数据,确定哪些变量包含了缺失值。
2. `deal1:deal2` 参数报错:这个错误和之前的问题类似,可能是因为 `deal1` 和 `deal2` 的数据类型不正确或存在缺失值导致的。你需要检查一下 `deal1` 和 `deal2` 的数据类型,并使用之前提到的方法处理缺失值。
以下是一个可能的解决方案:
```R
# 查找包含缺失值的行的索引号
missing_idx <- which(!complete.cases(data))
# 查看包含缺失值的行的数据
data[missing_idx, ]
# 删除包含缺失值的行
data <- na.omit(data)
# 将 deal1 和 deal2 转换为因子型变量
data$deal1 <- as.factor(data$deal1)
data$deal2 <- as.factor(data$deal2)
# 进行方差分析
result <- with(data, kruskal.test(Fquantity ~ deal1:deal2:deal3))
```
这样处理之后,你应该能够避免这些错误并成功进行方差分析。
相关问题
> sum(!complete.cases(data)) [1] 1
这个结果说明在数据集中包含有一个包含缺失值的观测行。可以使用 `which` 函数查找包含缺失值的行的索引号,然后使用 `na.omit` 函数将这些行删除或使用其他方法进行处理。以下是一个示例代码:
```R
# 查找包含缺失值的行的索引号
missing_idx <- which(!complete.cases(data))
# 删除包含缺失值的行
data <- na.omit(data)
# 或者填充缺失值
data$Fquantity[missing_idx] <- mean(data$Fquantity, na.rm = TRUE)
```
这样处理之后,再次运行 `sum(!complete.cases(data))` 应该返回0,表示数据集中不再包含缺失值。
将下面代码写成matlab形式 int runBm3d( const Mat image_noisy, Mat& image_basic, Mat& image_denoised ) { int Height = image_noisy.rows; int Width = image_noisy.cols; int Channels = image_noisy.channels(); vector<Mat> block_noisy;//store the patch vector<int>row_idx;//patch idx along the row direction vector<int>col_idx; GetAllBlock(image_noisy, Width, Height, Channels, kHard, pHard, block_noisy, row_idx, col_idx); int bn_r = row_idx.size(); int bn_c = col_idx.size(); tran2d(block_noisy, kHard); vector<int> sim_num;//index number for the selected similar patch in the block vector vector<int> sim_idx_row;//index number for the selected similar patch in the original Mat vector<int> sim_idx_col; vector<Mat>data;//store the data during transforming and shrinking Mat kaiser = gen_kaiser(beta, kHard);//2-D kaiser window float weight_hd = 1.0;//weights used for current relevent patch Mat denominator_hd(image_noisy.size(), CV_32FC1, Scalar::all(0)); Mat numerator_hd(image_noisy.size(), CV_32FC1, Scalar::all(0)); for (int i = 0; i < bn_r; i++) { for (int j = 0; j < bn_c; j++) { //for each pack in the block sim_num.clear(); sim_idx_row.clear(); sim_idx_col.clear(); data.clear(); getSimilarPatch(block_noisy, data, sim_num, i, j, bn_r, bn_c, int((nHard - kHard) / pHard) + 1, NHard, tao_hard);//block matching for (int k = 0; k < sim_num.size(); k++)//calculate idx in the left-top corner { sim_idx_row.push_back(row_idx[sim_num[k] / bn_c]); sim_idx_col.push_back(col_idx[sim_num[k] % bn_c]); } tran1d(data, kHard);//3-D transforming DetectZero(data, lambda3d * sigma);//shrink the cofficient weight_hd = calculate_weight_hd(data, sigma); Inver3Dtrans(data,kHard);//3-D inverse transforming aggregation(numerator_hd, denominator_hd, sim_idx_row, sim_idx_col, data, weight_hd, kHard, kaiser);//aggregation using weigths } } image_basic = numerator_hd / denominator_hd;
function [image_basic,image_denoised] = runBm3d(image_noisy)
Height = size(image_noisy,1);
Width = size(image_noisy,2);
Channels = size(image_noisy,3);
block_noisy = {}; %store the patch
row_idx = []; %patch idx along the row direction
col_idx = [];
[block_noisy,row_idx,col_idx] = GetAllBlock(image_noisy, Width, Height, Channels, kHard, pHard);
bn_r = length(row_idx);
bn_c = length(col_idx);
block_noisy = tran2d(block_noisy, kHard);
sim_num = {}; %index number for the selected similar patch in the block vector
sim_idx_row = []; %index number for the selected similar patch in the original Mat
sim_idx_col = [];
data = {}; %store the data during transforming and shrinking
kaiser = gen_kaiser(beta, kHard); %2-D kaiser window
weight_hd = 1.0; %weights used for current relevent patch
denominator_hd = zeros(size(image_noisy),'single');
numerator_hd = zeros(size(image_noisy),'single');
for i = 1:bn_r
for j = 1:bn_c
%for each pack in the block
sim_num = {};
sim_idx_row = [];
sim_idx_col = [];
data = {};
[data,sim_num] = getSimilarPatch(block_noisy, i, j, bn_r, bn_c, int32((nHard - kHard) / pHard) + 1, NHard, tao_hard); %block matching
for k = 1:length(sim_num) %calculate idx in the left-top corner
sim_idx_row(k) = row_idx(sim_num(k) / bn_c);
sim_idx_col(k) = col_idx(mod(sim_num(k), bn_c));
end
data = tran1d(data, kHard); %3-D transforming
data = DetectZero(data, lambda3d * sigma); %shrink the cofficient
weight_hd = calculate_weight_hd(data, sigma);
data = Inver3Dtrans(data,kHard); %3-D inverse transforming
[numerator_hd, denominator_hd] = aggregation(numerator_hd, denominator_hd, sim_idx_row, sim_idx_col, data, weight_hd, kHard, kaiser); %aggregation using weigths
end
end
image_basic = numerator_hd ./ denominator_hd;
end
阅读全文
相关推荐


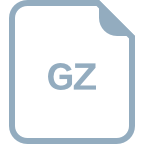









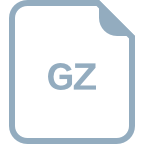


