pytorch中调用focal loss
时间: 2023-11-27 19:02:09 浏览: 30
在 PyTorch 中,可以通过使用 torch.nn.functional 中的 focal_loss 函数来实现 Focal Loss。以下是一个示例代码片段:
```python
import torch.nn.functional as F
def focal_loss(prediction, target, alpha=0.25, gamma=2):
pt = torch.exp(-F.binary_cross_entropy(prediction, target, reduction='none'))
loss = alpha * (1-pt)**gamma * F.binary_cross_entropy(prediction, target, reduction='mean')
return loss
```
在上面的代码中,prediction 是模型的输出,target 是标签,alpha 和 gamma 是 Focal Loss 中的两个超参数。函数中的代码实现了标准 Focal Loss 的计算流程。
相关问题
用pytorch写一下focalloss
当你使用PyTorch来实现Focal Loss时,你需要定义一个损失函数,它可以接受输入的预测值和目标值,并返回一个标量,表示预测和目标之间的损失。 损失函数的定义如下:Focal Loss = -α*(1-pt)^γ*log(pt),其中pt是预测值和目标之间的比率,α是权重系数,γ是焦点参数,用于控制预测值和目标之间的差异。
pytorch如何实现focal loss,实例说明
在PyTorch中,可以通过定义一个自定义的损失函数来实现Focal Loss。Focal Loss是一种针对类别不平衡问题的损失函数,可以帮助模型更好地处理少数类别的样本。
下面是一个实现Focal Loss的代码示例:
```python
import torch
import torch.nn as nn
class FocalLoss(nn.Module):
def __init__(self, alpha=1, gamma=2, reduction='mean'):
super(FocalLoss, self).__init__()
self.alpha = alpha
self.gamma = gamma
self.reduction = reduction
def forward(self, inputs, targets):
BCE_loss = nn.CrossEntropyLoss(reduction='none')(inputs, targets)
pt = torch.exp(-BCE_loss)
F_loss = self.alpha * (1-pt)**self.gamma * BCE_loss
if self.reduction == 'mean':
return torch.mean(F_loss)
elif self.reduction == 'sum':
return torch.sum(F_loss)
else:
return F_loss
```
在这个代码中,`alpha`是用来平衡不同类别的权重的超参数,`gamma`是控制难易样本权重的超参数,`reduction`是用来控制损失函数的计算方式,可以选择`mean`或`sum`。
使用示例代码:
```python
import torch
import torch.optim as optim
# 定义模型和优化器
model = ...
criterion = FocalLoss(alpha=1, gamma=2)
optimizer = optim.Adam(model.parameters())
# 训练模型
for epoch in range(num_epochs):
# 前向传播
outputs = model(inputs)
loss = criterion(outputs, targets)
# 反向传播和优化器更新
optimizer.zero_grad()
loss.backward()
optimizer.step()
```
这里的`inputs`是模型输入的数据,`targets`是对应数据的真实标签。在训练过程中,通过计算Focal Loss来更新模型参数。
相关推荐
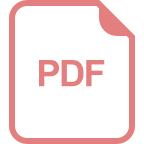












