能不能python实现图像纹理特征自回归模型
时间: 2024-01-10 08:02:55 浏览: 160
能够实现图像纹理特征自回归模型,具体步骤如下:
1. 导入必要的库和模块,如numpy、cv2、sklearn等。
2. 加载图像,并将其转化为灰度图像。
3. 对灰度图像进行LBP(Local Binary Pattern)纹理特征提取,得到纹理特征图像。
4. 对纹理特征图像进行自回归建模,得到纹理特征自回归模型。
5. 使用训练好的纹理特征自回归模型对新的图像进行纹理特征预测,得到预测结果。
下面是一个简单的示例代码:
```python
import numpy as np
import cv2
from sklearn.linear_model import LinearRegression
# 加载图像
img = cv2.imread('test.jpg')
gray = cv2.cvtColor(img, cv2.COLOR_BGR2GRAY)
# LBP纹理特征提取
radius = 3
n_points = 8 * radius
lbp = np.zeros_like(gray)
for i in range(radius, gray.shape[0] - radius):
for j in range(radius, gray.shape[1] - radius):
center = gray[i, j]
values = []
for x in range(-radius, radius + 1):
for y in range(-radius, radius + 1):
if x == 0 and y == 0:
continue
values.append(gray[i + x, j + y])
values = np.asarray(values)
lbp_value = np.sum(values >= center) * 2 ** np.arange(n_points)
lbp[i, j] = np.sum(lbp_value)
# 自回归建模
X = []
y = []
for i in range(radius, lbp.shape[0] - radius):
for j in range(radius, lbp.shape[1] - radius):
center = lbp[i, j]
values = []
for x in range(-radius, radius + 1):
for y in range(-radius, radius + 1):
if x == 0 and y == 0:
continue
values.append(lbp[i + x, j + y])
X.append(values)
y.append(center)
X = np.asarray(X)
y = np.asarray(y)
model = LinearRegression()
model.fit(X, y)
# 纹理特征预测
new_lbp = np.zeros_like(lbp)
for i in range(radius, lbp.shape[0] - radius):
for j in range(radius, lbp.shape[1] - radius):
center = lbp[i, j]
values = []
for x in range(-radius, radius + 1):
for y in range(-radius, radius + 1):
if x == 0 and y == 0:
continue
values.append(lbp[i + x, j + y])
values = np.asarray(values)
new_lbp[i, j] = model.predict(values.reshape(1, -1))
# 显示结果
cv2.imshow('Original', img)
cv2.imshow('LBP', lbp)
cv2.imshow('Predicted LBP', new_lbp)
cv2.waitKey(0)
cv2.destroyAllWindows()
```
需要注意的是,上述代码仅仅是一个简单示例,实际应用中需要根据具体的问题和数据进行调整和优化。
阅读全文
相关推荐
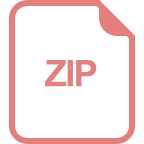
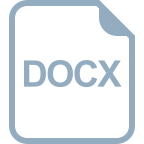


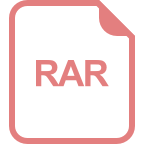
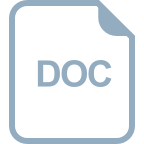
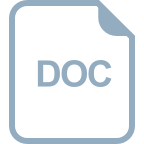
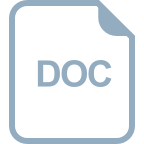

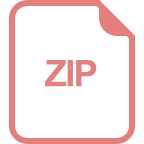
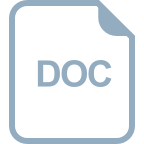
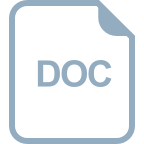
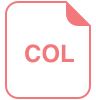
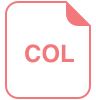
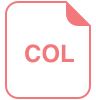
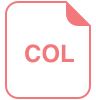
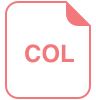
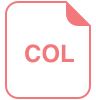