LSTM模型的python代码
时间: 2023-11-13 19:09:59 浏览: 83
以下是一个基本的LSTM模型的Python代码实现,包括数据预处理,模型构建,训练和预测。
```python
import numpy as np
from keras.models import Sequential
from keras.layers import Dense, LSTM
# 定义超参数
timesteps = 1
data_dim = 3
num_classes = 2
batch_size = 32
epochs = 100
# 生成模拟数据
x_train = np.random.random((1000, timesteps, data_dim))
y_train = np.random.randint(num_classes, size=(1000, 1))
y_train = np.eye(num_classes)[y_train.reshape(-1)]
# 构建模型
model = Sequential()
model.add(LSTM(32, return_sequences=True, input_shape=(timesteps, data_dim)))
model.add(LSTM(32, return_sequences=True))
model.add(LSTM(32))
model.add(Dense(num_classes, activation='softmax'))
# 编译模型
model.compile(loss='categorical_crossentropy',
optimizer='adam',
metrics=['accuracy'])
# 训练模型
model.fit(x_train, y_train,
batch_size=batch_size,
epochs=epochs,
validation_split=0.2)
# 预测新数据
x_test = np.random.random((100, timesteps, data_dim))
y_test = np.random.randint(num_classes, size=(100, 1))
y_test = np.eye(num_classes)[y_test.reshape(-1)]
score = model.evaluate(x_test, y_test, batch_size=batch_size)
print('Test loss:', score[0])
print('Test accuracy:', score[1])
```
这个LSTM模型包含3个LSTM层和1个全连接层。它使用随机生成的数据来进行训练和测试。在训练完成后,它会输出测试集上的损失和准确率。你可以根据自己的数据来修改超参数和模型结构。
阅读全文
相关推荐
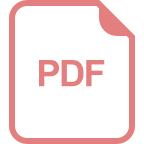
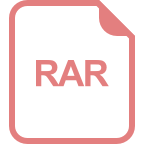








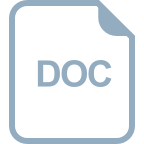






