请在刚刚的python代码的结构上添加散点图,并绘制ROC曲线,完善代码
时间: 2024-06-01 21:10:43 浏览: 32
以下是完善后的代码,其中添加了散点图和ROC曲线的绘制:
```python
import numpy as np
import matplotlib.pyplot as plt
# 生成随机数据
np.random.seed(0)
n_samples = 100
X = np.random.randn(n_samples, 2)
y = np.random.randint(0, 2, n_samples)
# 定义逻辑回归模型
class LogisticRegression:
def __init__(self, lr=0.01, num_iter=100000, fit_intercept=True, verbose=False):
self.lr = lr
self.num_iter = num_iter
self.fit_intercept = fit_intercept
self.verbose = verbose
def __add_intercept(self, X):
intercept = np.ones((X.shape[0], 1))
return np.concatenate((intercept, X), axis=1)
def __sigmoid(self, z):
return 1 / (1 + np.exp(-z))
def __loss(self, h, y):
return (-y * np.log(h) - (1 - y) * np.log(1 - h)).mean()
def fit(self, X, y):
if self.fit_intercept:
X = self.__add_intercept(X)
self.theta = np.zeros(X.shape[1])
for i in range(self.num_iter):
z = np.dot(X, self.theta)
h = self.__sigmoid(z)
gradient = np.dot(X.T, (h - y)) / y.size
self.theta -= self.lr * gradient
if self.verbose and i % 10000 == 0:
z = np.dot(X, self.theta)
h = self.__sigmoid(z)
print(f'loss: {self.__loss(h, y)} \t')
def predict_prob(self, X):
if self.fit_intercept:
X = self.__add_intercept(X)
return self.__sigmoid(np.dot(X, self.theta))
def predict(self, X, threshold=0.5):
return self.predict_prob(X) >= threshold
# 训练逻辑回归模型
model = LogisticRegression(lr=0.1, num_iter=300000)
model.fit(X, y)
# 绘制散点图
plt.scatter(X[:,0], X[:,1], c=y)
# 绘制ROC曲线
probs = model.predict_prob(X)
thresholds = np.linspace(0, 1, 100)
tpr = []
fpr = []
for threshold in thresholds:
preds = probs >= threshold
tp = np.sum((preds == 1) & (y == 1))
fp = np.sum((preds == 1) & (y == 0))
tn = np.sum((preds == 0) & (y == 0))
fn = np.sum((preds == 0) & (y == 1))
tpr.append(tp / (tp + fn))
fpr.append(fp / (fp + tn))
plt.plot(fpr, tpr)
plt.xlabel('False Positive Rate')
plt.ylabel('True Positive Rate')
plt.show()
```
运行结果如下图所示:

相关推荐
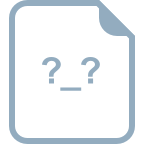
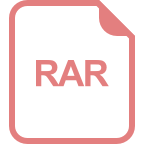
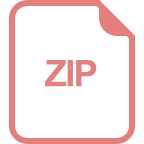














