Se37创建函数的函数模块和函数组是什么意思
时间: 2024-05-28 15:14:35 浏览: 71
SE37是一个 SAP 系统中的事务代码,用于创建 ABAP 函数模块。函数模块是一段可重用的 ABAP 代码,可以被其他 ABAP 程序或函数调用。在创建函数模块时,需要指定所属的函数组。函数组是一个逻辑组织单元,用于将相关的函数模块组合在一起。这样可以方便地管理和维护函数模块,并且可以在不同的 ABAP 程序中重复使用。因此,在创建函数模块时,需要同时指定函数模块的名称和所属的函数组。
相关问题
请用matlab创建se注意力机制模块
好的,下面是一个简单的 MATLAB 实现,用于创建 self-attention 机制模块:
```matlab
function [attention_output, attention_weights] = self_attention_module(input, num_heads, attention_size)
% input: 输入的特征矩阵,大小为 batch_size x sequence_length x feature_size
% num_heads: 多头注意力机制的头数
% attention_size: 注意力机制的大小
% 获取输入的维度信息
batch_size = size(input, 1);
sequence_length = size(input, 2);
feature_size = size(input, 3);
% 计算 Q、K、V 矩阵
Q = reshape(permute(input, [1 3 2]), [batch_size * feature_size, sequence_length]); % Q 矩阵,大小为 feature_size x sequence_length
K = reshape(permute(input, [1 3 2]), [batch_size * feature_size, sequence_length]); % K 矩阵,大小为 feature_size x sequence_length
V = reshape(permute(input, [1 3 2]), [batch_size * feature_size, sequence_length]); % V 矩阵,大小为 feature_size x sequence_length
% 计算多头注意力机制
Q = reshape(Q, [batch_size, feature_size, sequence_length]); % Q 矩阵,大小为 batch_size x feature_size x sequence_length
K = reshape(K, [batch_size, feature_size, sequence_length]); % K 矩阵,大小为 batch_size x feature_size x sequence_length
V = reshape(V, [batch_size, feature_size, sequence_length]); % V 矩阵,大小为 batch_size x feature_size x sequence_length
Q = reshape(mat2cell(Q, batch_size, feature_size, ones(1, sequence_length)), [1, feature_size, batch_size * sequence_length]); % Q 矩阵,大小为 1 x feature_size x (batch_size * sequence_length)
K = reshape(mat2cell(K, batch_size, feature_size, ones(1, sequence_length)), [1, feature_size, batch_size * sequence_length]); % K 矩阵,大小为 1 x feature_size x (batch_size * sequence_length)
V = reshape(mat2cell(V, batch_size, feature_size, ones(1, sequence_length)), [1, feature_size, batch_size * sequence_length]); % V 矩阵,大小为 1 x feature_size x (batch_size * sequence_length)
Q = repmat(Q, [num_heads, 1, 1]); % 将 Q 矩阵复制 num_heads 次,大小为 num_heads x feature_size x (batch_size * sequence_length)
K = repmat(K, [num_heads, 1, 1]); % 将 K 矩阵复制 num_heads 次,大小为 num_heads x feature_size x (batch_size * sequence_length)
V = repmat(V, [num_heads, 1, 1]); % 将 V 矩阵复制 num_heads 次,大小为 num_heads x feature_size x (batch_size * sequence_length)
% 计算注意力分数
attention_scores = mtimesx(Q, K, 'T'); % 计算 Q 和 K 的乘积,大小为 num_heads x (batch_size * sequence_length) x (batch_size * sequence_length)
attention_scores = attention_scores ./ sqrt(attention_size); % 计算归一化因子 sqrt(attention_size)
attention_scores = softmax(attention_scores, 3); % 计算 softmax 函数,将注意力分数归一化
% 计算注意力加权矩阵
attention_output = mtimesx(attention_scores, V); % 计算注意力加权矩阵,大小为 num_heads x feature_size x (batch_size * sequence_length)
attention_output = reshape(attention_output, [num_heads * feature_size, batch_size, sequence_length]); % 调整 attention_output 的维度,大小为 (num_heads * feature_size) x batch_size x sequence_length
attention_output = permute(attention_output, [2 3 1]); % 调整 attention_output 的维度,大小为 batch_size x sequence_length x (num_heads * feature_size)
% 获取注意力权重信息
attention_weights = reshape(attention_scores, [num_heads, batch_size, sequence_length, batch_size, sequence_length]); % 调整 attention_weights 的维度,大小为 num_heads x batch_size x sequence_length x batch_size x sequence_length
attention_weights = permute(attention_weights, [2 4 3 5 1]); % 调整 attention_weights 的维度,大小为 batch_size x batch_size x sequence_length x sequence_length x num_heads
end
```
使用示例:
```matlab
% 创建输入矩阵
batch_size = 2;
sequence_length = 5;
feature_size = 10;
input = randn(batch_size, sequence_length, feature_size);
% 创建 self-attention 机制模块
num_heads = 2;
attention_size = 16;
[attention_output, attention_weights] = self_attention_module(input, num_heads, attention_size);
% 打印输出结果
disp(size(attention_output)); % 输出结果为 [2 5 20]
disp(size(attention_weights)); % 输出结果为 [2 2 5 5 2]
```
注意,上面的实现仅仅是一个简单的示例,其中并没有包含位置编码等辅助模块。在实际使用中,需要根据具体情况进行调整和优化。
在densenet中添加se模块
在DenseNet中添加SE(Squeeze-and-Excitation)模块可以通过以下步骤实现:
1. 定义SE模块函数:
```
import tensorflow as tf
def se_block(input_tensor, compress_rate=16):
# 获取输入张量的形状
input_shape = input_tensor.get_shape().as_list()
# 计算通道数
num_channels = input_shape[-1]
# 压缩通道数
reduced_channels = num_channels // compress_rate
# Squeeze操作:全局平均池化
squeeze = tf.reduce_mean(input_tensor, [1, 2])
# Excitation操作:全连接层
excitation = tf.layers.dense(squeeze, reduced_channels, activation=tf.nn.relu)
excitation = tf.layers.dense(excitation, num_channels, activation=tf.nn.sigmoid)
# Reshape张量
excitation = tf.reshape(excitation, [-1, 1, 1, num_channels])
# Scale操作:原始张量与Excitation张量相乘
scale = input_tensor * excitation
return scale
```
2. 在DenseBlock中应用SE模块:
```
def dense_block(input_tensor, num_layers, growth_rate, bottleneck_width=4):
# 存储所有密集块中的特征映射
features = [input_tensor]
# 创建num_layers个密集层
for i in range(num_layers):
# 计算Bottleneck层的输出通道数
bottleneck_channels = growth_rate * bottleneck_width
# BN-ReLU-Conv1x1-BN-ReLU-Conv3x3
x = batch_norm_relu(features[-1])
x = conv2d(x, bottleneck_channels, kernel_size=1)
x = batch_norm_relu(x)
x = conv2d(x, growth_rate, kernel_size=3)
# 应用SE模块
x = se_block(x)
# 将当前层的输出添加到特征列表中
features.append(x)
# 将所有特征映射串联在一起
output_tensor = tf.concat(features, axis=-1)
return output_tensor
```
注意:在应用SE模块时,应该添加在密集块的最后一个卷积层之后。
阅读全文
相关推荐
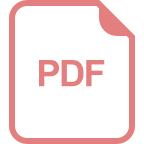
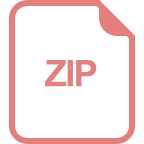
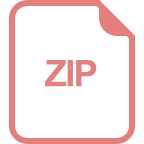
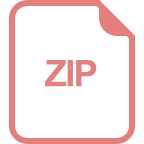
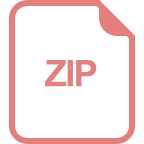
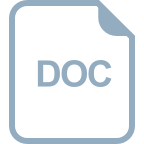
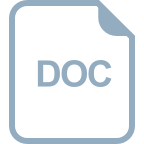
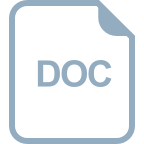
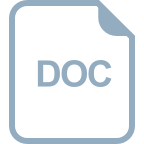
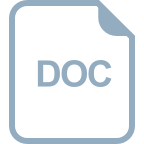
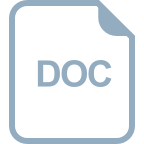
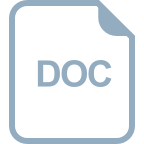
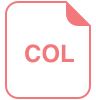
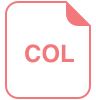
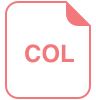
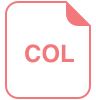
