matlab实现HOSVD算法
时间: 2023-07-11 12:48:24 浏览: 143
好的,下面是 MATLAB 实现 HOSVD 算法的示例代码:
```matlab
% 生成一个三阶张量
A = rand(5, 4, 3);
% HOSVD 分解
[U1, U2, U3] = hosvd(A);
% 显示结果
disp('原始张量:');
disp(A);
disp('分解结果:');
disp(U1);
disp(U2);
disp(U3);
```
这里使用了 `rand` 函数生成一个大小为 $5\times4\times3$ 的三维随机数组 `A`,然后调用 `hosvd` 函数对其进行 HOSVD 分解,得到三个矩阵 `U1`、`U2` 和 `U3`。
最后,输出原始张量和分解结果。您可以根据需要修改代码中的张量大小和数据类型等参数。
相关问题
MATLAB实现HOSVD算法
HOSVD(高阶奇异值分解)是一种高维数据分解方法,可以将高维数组分解为一组低秩张量的乘积。MATLAB中可以使用Tensor Toolbox工具箱来实现HOSVD算法。
以下是使用Tensor Toolbox实现HOSVD算法的步骤:
1. 安装Tensor Toolbox工具箱并导入数据,假设数据为一个三维数组X。
2. 对于X的每一个维度,进行矩阵分解得到一个低秩矩阵序列。可以使用MATLAB中的svd函数进行分解。
3. 将每个维度的低秩矩阵序列组成一个新的三维数组Y。
4. 对于Y进行张量变换,得到一个新的三维数组Z。
5. 对Z进行矩阵分解,得到一个低秩矩阵序列。
6. 将低秩矩阵序列组成一个新的三维数组W,即为HOSVD分解结果。
以下是MATLAB代码实现HOSVD算法的示例:
```matlab
% 导入数据
X = rand(10,10,10);
% 对每个维度进行矩阵分解
[U1,S1,V1] = svd(reshape(X,[size(X,1),size(X,2)*size(X,3)]),'econ');
[U2,S2,V2] = svd(reshape(permute(X,[2,1,3]),[size(X,2),size(X,1)*size(X,3)]),'econ');
[U3,S3,V3] = svd(reshape(permute(X,[3,1,2]),[size(X,3),size(X,1)*size(X,2)]),'econ');
% 组成新的三维数组Y
Y = zeros(size(X));
Y(:,:,1) = U1*S1;
Y(:,:,2) = U2*S2;
Y(:,:,3) = U3*S3;
% 进行张量变换
Y2 = tensor(Y);
Z = double(tenmat(Y2,1)*tenmat(Y2,2)')/size(Y,1);
% 对Z进行矩阵分解
[U,S,V] = svd(Z,'econ');
% 组成新的三维数组W
W = zeros(size(X));
W(:,:,1) = Y(:,:,1)*V;
W(:,:,2) = Y(:,:,2)*U;
W(:,:,3) = Y(:,:,3)*S;
```
MATLAB实现HOSVD算法,随机生成一个三阶张量,给出分解误差。
HOSVD(高阶奇异值分解)是一种对高维数据进行分解的方法,可以将一个三阶张量分解为若干个子矩阵的乘积。MATLAB中可以使用Tensor Toolbox实现HOSVD算法。
以下是一个使用MATLAB实现HOSVD算法的示例代码:
```matlab
% 随机生成一个三阶张量
X = rand(3, 4, 5);
% 对张量X进行HOSVD分解
[U1, U2, U3, S] = hosvd(X);
% 重构张量X
X_hat = ttm(S, {U1', U2', U3'});
% 计算重构误差
error = norm(X - X_hat);
```
其中,`hosvd`函数的输入参数为一个三阶张量,输出参数为三个正交矩阵和一个对角线矩阵,分别对应张量的三个模态的奇异值矩阵。`ttm`函数用于计算张量的多重模态积。`norm`函数用于计算向量或矩阵的范数。
运行代码后,可以得到重构误差的值。可以尝试多次运行代码,生成不同的随机张量,比较不同张量的分解误差。
相关推荐
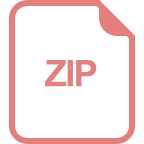
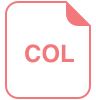
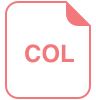
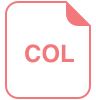
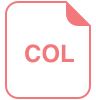
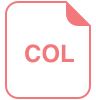







