identity_matrix = [[0] * len_message for _ in range(len_message)]
时间: 2024-03-26 10:36:29 浏览: 21
这行代码是用来创建一个长度为len_message的零矩阵,矩阵的大小为len_message x len_message。具体来说,这行代码使用了Python中的列表推导式和嵌套列表,相当于执行了以下代码:
```python
identity_matrix = []
for i in range(len_message):
row = [0] * len_message
identity_matrix.append(row)
```
列表推导式部分 `[0] * len_message` 创建了一个长度为len_message的列表,每个元素都是0。然后,循环执行`range(len_message)`,即`[0, 1, 2, ..., len_message-1]`,对于每个循环变量i,都创建了一个长度为len_message的行,即`[0, 0, ..., 0]`,并将其添加到identity_matrix中。
其中,由于没有使用循环变量i,因此使用了下划线_来代替循环变量。这种方式可以在循环过程中忽略循环变量,因为这里只需要循环len_message次,而不需要使用循环变量i的值。
相关问题
注意力机制self.space_attention = nn.Sequential( nn.Conv2d(512, 512, kernel_size=3, padding=1, bias=True), nn.BatchNorm2d(512), nn.ReLU(inplace=True) ) self.channel_attention = nn.Sequential( nn.Conv2d(512, 512, kernel_size=3, padding=1, bias=True), nn.BatchNorm2d(512), nn.ReLU(inplace=True) ) out_c1 = nn.AdaptiveMaxPool2d((1, 1))(x4_0) out_c1 = self.sigmoid(out_c1) channel_feature = x4_0 * out_c1 channel_att = self.channel_attention(channel_feature) x = x4_0 + channel_att out_s1 = torch.max(x, 1)[0].unsqueeze(1) out_s1 = self.sigmoid(out_s1) space_feature = x * out_s1 space_att = self.space_attention(space_feature) x = x + space_att 想在网络中换成self.attention = BiLevelRoutingAttention(dim=512, n_win=7, num_heads=8, qk_dim=None, qk_scale=None, kv_per_win=4, kv_downsample_ratio=4, kv_downsample_kernel=None, kv_downsample_mode='identity', topk=4, param_attention="qkvo", param_routing=False, diff_routing=False, soft_routing=False, side_dwconv=3, auto_pad=True)之后代码怎么写啊
如果您想在网络中使用`BiLevelRoutingAttention`,可以将原来的`self.space_attention`和`self.channel_attention`替换为`self.attention`,然后在`__init__`函数中初始化`self.attention`,并将其输入和输出的维度设置为512(因为原来的代码中维度为512)。代码示例如下:
```
from attention import BiLevelRoutingAttention
class YourNetwork(nn.Module):
def __init__(self):
super(YourNetwork, self).__init__()
self.attention = BiLevelRoutingAttention(dim=512, n_win=7, num_heads=8, qk_dim=None, qk_scale=None,
kv_per_win=4, kv_downsample_ratio=4, kv_downsample_kernel=None,
kv_downsample_mode='identity', topk=4, param_attention="qkvo",
param_routing=False, diff_routing=False, soft_routing=False,
side_dwconv=3, auto_pad=True)
# 其他层的初始化
...
def forward(self, x):
# 其他层的前向传播
...
out_c1 = nn.AdaptiveMaxPool2d((1, 1))(x4_0)
out_c1 = self.sigmoid(out_c1)
channel_feature = x4_0 * out_c1
channel_att = self.attention(channel_feature) # 使用BiLevelRoutingAttention
x = x4_0 + channel_att
out_s1 = torch.max(x, 1)[0].unsqueeze(1)
out_s1 = self.sigmoid(out_s1)
space_feature = x * out_s1
space_att = self.attention(space_feature) # 使用BiLevelRoutingAttention
x = x + space_att
# 其他层的后续处理
...
return x
```
注意,`BiLevelRoutingAttention`的输入和输出需要满足一定的维度要求,具体可参考`attention.py`文件中的实现。因此,如果您的输入和输出维度不符合要求,可能需要对其进行调整。
self.fc1 = nn.Sequential( nn.Linear(in_features=12*7*7, out_features=196), nn.ReLU(), ) # 补充第二个全连接层... self.fc2 = nn.Sequential( nn.Linear(in_features=196, out_features=84), nn.ReLU(), ) # 补充第三个全连接层... self.fc2 = nn.Sequential( nn.Linear(in_features=84, out_features=n_classes), nn.ReLU(), )有什么错误吗
这段代码有一个错误,就是最后一个全连接层的定义重复了,应该将其改为`self.fc3`,而不是`self.fc2`。正确的代码如下:
```
self.fc1 = nn.Sequential(
nn.Linear(in_features=12*7*7, out_features=196),
nn.ReLU(),
)
# 补充第二个全连接层...
self.fc2 = nn.Sequential(
nn.Linear(in_features=196, out_features=84),
nn.ReLU(),
)
# 补充第三个全连接层...
self.fc3 = nn.Sequential(
nn.Linear(in_features=84, out_features=n_classes),
nn.ReLU(),
)
```
其中,`n_classes`表示分类的类别数。在最后一个全连接层中,一般不使用激活函数,因为输出结果需要经过softmax进行归一化处理。因此,可以将nn.ReLU()改为nn.Identity(),即不使用任何激活函数。
相关推荐
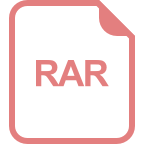
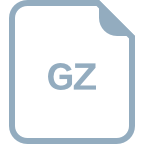












