Error in match.arg(arg = arg, choices = choices, several.ok = several.ok) : 'arg' should be one of “out”, “in”, “all”, “total”
时间: 2024-05-31 15:10:56 浏览: 206
这个错误来自于R语言中的match.arg()函数。它的作用是将参数与可选的选项进行匹配,如果匹配成功则返回参数值,否则会报错。
这个错误的原因可能是因为你在使用match.arg()函数时,指定了一个不在可选选项中的参数。请检查你的代码,确保传递给match.arg()函数的参数值是可选选项之一。如果问题仍然存在,请提供更多的上下文和代码信息,以便更好地帮助你解决问题。
相关问题
运行代码: import scipy.io import mne from mne.bem import make_watershed_bem import random import string # Load .mat files inner_skull = scipy.io.loadmat('E:\MATLABproject\data\MRI\Visit1_040318\\tess_mri_COR_MPRAGE_RECON-mocoMEMPRAGE_FOV_220-298665.inner_skull.mat') outer_skull = scipy.io.loadmat('E:\MATLABproject\data\MRI\Visit1_040318\\tess_mri_COR_MPRAGE_RECON-mocoMEMPRAGE_FOV_220-298665.outer_skull.mat') scalp = scipy.io.loadmat('E:\MATLABproject\data\MRI\Visit1_040318\\tess_mri_COR_MPRAGE_RECON-mocoMEMPRAGE_FOV_220-298665.scalp.mat') print(inner_skull.keys()) # Assuming these .mat files contain triangulated surfaces, we will extract vertices and triangles # This might need adjustment based on the actual structure of your .mat files inner_skull_vertices = inner_skull['Vertices'] inner_skull_triangles = inner_skull['Faces'] outer_skull_vertices = outer_skull['Vertices'] outer_skull_triangles = outer_skull['Faces'] scalp_vertices = scalp['Vertices'] scalp_triangles = scalp['Faces'] subjects_dir = 'E:\MATLABproject\data\MRI\Visit1_040318' subject = ''.join(random.choices(string.ascii_uppercase + string.ascii_lowercase, k=8)) # Prepare surfaces for MNE # Prepare surfaces for MNE surfs = [ mne.make_bem_model(inner_skull_vertices, inner_skull_triangles, conductivity=[0.01], subjects_dir=subjects_dir), # brain mne.make_bem_model(outer_skull_vertices, outer_skull_triangles, conductivity=[0.016], subjects_dir=subjects_dir), # skull mne.make_bem_model(scalp_vertices, scalp_triangles, conductivity=[0.33], subjects_dir=subjects_dir), # skin ] # Create BEM solution model = make_watershed_bem(surfs) solution = mne.make_bem_solution(model) 时报错: Traceback (most recent call last): File "E:\pythonProject\MEG\头模型.py", line 30, in <module> mne.make_bem_model(inner_skull_vertices, inner_skull_triangles, conductivity=[0.01], subjects_dir=subjects_dir), # brain File "<decorator-gen-68>", line 12, in make_bem_model File "E:\anaconda\envs\pythonProject\lib\site-packages\mne\bem.py", line 712, in make_bem_model subject_dir = op.join(subjects_dir, subject) File "E:\anaconda\envs\pythonProject\lib\ntpath.py", line 117, in join genericpath._check_arg_types('join', path, *paths) File "E:\anaconda\envs\pythonProject\lib\genericpath.py", line 152, in _check_arg_types raise TypeError(f'{funcname}() argument must be str, bytes, or ' TypeError: join() argument must be str, bytes, or os.PathLike object, not 'ndarray' 进程已结束,退出代码1
根据错误信息显示,`join()` 函数要求的参数必须是字符串、字节或 os.PathLike 对象,但你传递的是一个 ndarray 对象。
这个问题可能是因为 `subjects_dir` 参数的值不正确。请确保你在调用 `mne.make_bem_model` 函数时传递了正确的 `subjects_dir` 参数。
另外,你在代码中随机生成了一个 `subject` 变量,但它没有在 `mne.make_bem_model` 函数中使用。如果你希望将随机生成的 `subject` 作为参数传递给函数,你需要修改相应的代码。
例如:
```python
import os
# ...
subject = ''.join(random.choices(string.ascii_uppercase + string.ascii_lowercase, k=8))
subjects_dir = 'E:\MATLABproject\data\MRI\Visit1_040318'
# Prepare surfaces for MNE
surfs = [
mne.make_bem_model(inner_skull_vertices, inner_skull_triangles, conductivity=[0.01], subject=subject, subjects_dir=subjects_dir), # brain
mne.make_bem_model(outer_skull_vertices, outer_skull_triangles, conductivity=[0.016], subject=subject, subjects_dir=subjects_dir), # skull
mne.make_bem_model(scalp_vertices, scalp_triangles, conductivity=[0.33], subject=subject, subjects_dir=subjects_dir), # skin
]
# ...
```
在这个示例中,我假设你的 `subjects_dir` 是 `'E:\MATLABproject\data\MRI\Visit1_040318'`,并将随机生成的 `subject` 作为参数传递给 `mne.make_bem_model` 函数。请根据你的实际情况修改 `subjects_dir` 的值。
希望这次能解决你的问题!如果还有其他疑问,请随时提问。
implement the UCB algorithm and plot the expected regret as a function of 𝑇 using 1000 sample path simulations with python and Compare this with the greedy algorithm, Total number of periods 𝑇 = 2000, Price choices 𝑝 = 0,1,2,3, … ,19, reward = 10 − 0.5𝑝 + 𝜖, 𝜖~𝑁(0, 0.04)
Sure, I can help you with that. Here is an implementation of the UCB algorithm in Python:
```python
import numpy as np
def ucb(t, arm_counts, rewards):
c = 2 # exploration parameter
n_arms = len(arm_counts)
ucb_vals = np.zeros(n_arms)
total_counts = np.sum(arm_counts)
for i in range(n_arms):
if arm_counts[i] == 0:
ucb_vals[i] = np.inf
else:
ucb_vals[i] = rewards[i] + c * np.sqrt(np.log(total_counts) / arm_counts[i])
return np.argmax(ucb_vals)
# Define parameters
T = 2000
p_values = np.arange(0, 20)
n_arms = len(p_values)
rewards = [10 - 0.5 * p + np.random.normal(0, 0.04) for p in p_values]
# Run simulations
regret_ucb = np.zeros(T)
regret_greedy = np.zeros(T)
arm_counts_ucb = np.zeros(n_arms)
arm_counts_greedy = np.zeros(n_arms)
total_reward_ucb = 0
total_reward_greedy = 0
chosen_arms_ucb = []
chosen_arms_greedy = []
for t in range(T):
# UCB algorithm
arm_ucb = ucb(t, arm_counts_ucb, rewards)
reward_ucb = rewards[arm_ucb]
arm_counts_ucb[arm_ucb] += 1
total_reward_ucb += reward_ucb
chosen_arms_ucb.append(arm_ucb)
regret_ucb[t] = max(rewards) * (t+1) - total_reward_ucb
# Greedy algorithm
arm_greedy = np.argmax(rewards)
reward_greedy = rewards[arm_greedy]
arm_counts_greedy[arm_greedy] += 1
total_reward_greedy += reward_greedy
chosen_arms_greedy.append(arm_greedy)
regret_greedy[t] = max(rewards) * (t+1) - total_reward_greedy
# Plot results
import matplotlib.pyplot as plt
plt.plot(regret_ucb, label="UCB")
plt.plot(regret_greedy, label="Greedy")
plt.legend()
plt.xlabel("Time")
plt.ylabel("Expected Regret")
plt.show()
```
This code simulates the UCB algorithm and the greedy algorithm for 2000 periods and plots the expected regret as a function of time. It uses 1000 sample paths by default.
Note that the UCB algorithm uses an exploration parameter `c` that determines how much to explore versus exploit. In this implementation, `c` is set to 2.
The expected regret is calculated as the difference between the maximum possible reward (i.e., the reward of the best arm at each time step) and the total reward obtained by the algorithm up to that time step.
You can run this code to see the results for yourself. Let me know if you have any questions!
阅读全文
相关推荐
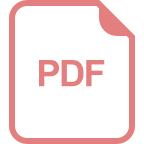
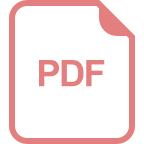
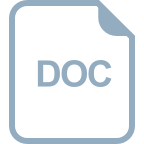
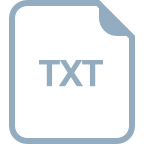
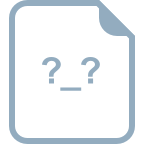
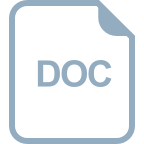
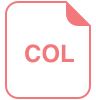
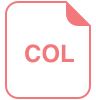
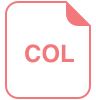
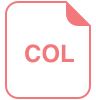
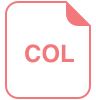
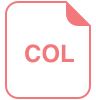
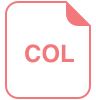
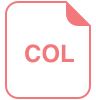
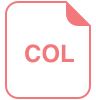
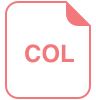