深度学习代码讲解
时间: 2023-06-28 09:14:45 浏览: 50
深度学习代码通常使用Python编写,常用的深度学习框架包括TensorFlow、Keras、PyTorch等。下面以TensorFlow为例,简单讲解深度学习代码的基本结构。
1.导入库
首先需要导入TensorFlow库,以及其他需要使用的Python库,例如NumPy、Matplotlib等。
```python
import tensorflow as tf
import numpy as np
import matplotlib.pyplot as plt
```
2.准备数据
在深度学习中,需要准备训练数据和测试数据。通常使用NumPy数组来表示数据。
```python
x_train = np.array([...]) # 训练数据
y_train = np.array([...]) # 训练数据标签
x_test = np.array([...]) # 测试数据
y_test = np.array([...]) # 测试数据标签
```
3.构建模型
在TensorFlow中,可以使用Keras API来构建深度学习模型。下面是一个简单的全连接神经网络模型的代码示例。
```python
model = tf.keras.Sequential([
tf.keras.layers.Dense(128, activation='relu', input_shape=(784,)),
tf.keras.layers.Dense(10, activation='softmax')
])
```
4.编译模型
在构建完模型后,需要编译模型以进行训练。在编译模型时,需要选择损失函数、优化器和评价指标。
```python
model.compile(loss='categorical_crossentropy',
optimizer='adam',
metrics=['accuracy'])
```
5.训练模型
在编译完模型后,可以使用训练数据对模型进行训练。
```python
history = model.fit(x_train, y_train, epochs=10, validation_data=(x_test, y_test))
```
6.评估模型
在模型训练完成后,可以使用测试数据评估模型的性能。
```python
test_loss, test_acc = model.evaluate(x_test, y_test)
print('Test accuracy:', test_acc)
```
7.使用模型进行预测
在模型训练完成后,可以使用模型对新数据进行预测。
```python
predictions = model.predict(x_new)
```
以上是深度学习代码的基本结构,具体的实现会根据具体问题而有所不同。
相关推荐
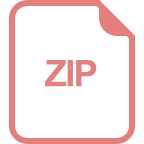
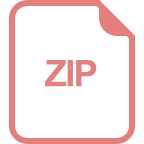
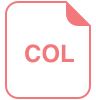
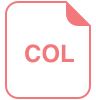
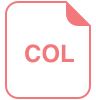
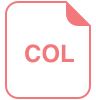
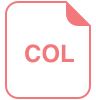









