resnet20网络结构
时间: 2023-05-16 19:06:06 浏览: 620
ResNet20网络结构是一个深度残差网络,由20个卷积层和全连接层组成。每个卷积层都有一个残差块,其中包括两个卷积层和一个跳跃连接。该网络的主要目的是解决深度神经网络中的梯度消失和梯度爆炸问题,从而提高网络的训练效率和准确性。
相关问题
打印resnet20网络结构
以下是resnet20网络结构的代码:
```python
import torch.nn as nn
class BasicBlock(nn.Module):
expansion = 1
def __init__(self, in_planes, planes, stride=1):
super(BasicBlock, self).__init__()
self.conv1 = nn.Conv2d(in_planes, planes, kernel_size=3, stride=stride, padding=1, bias=False)
self.bn1 = nn.BatchNorm2d(planes)
self.conv2 = nn.Conv2d(planes, planes, kernel_size=3, stride=1, padding=1, bias=False)
self.bn2 = nn.BatchNorm2d(planes)
self.shortcut = nn.Sequential()
if stride != 1 or in_planes != self.expansion*planes:
self.shortcut = nn.Sequential(
nn.Conv2d(in_planes, self.expansion*planes, kernel_size=1, stride=stride, bias=False),
nn.BatchNorm2d(self.expansion*planes)
)
def forward(self, x):
out = nn.ReLU()(self.bn1(self.conv1(x)))
out = self.bn2(self.conv2(out))
out += self.shortcut(x)
out = nn.ReLU()(out)
return out
class ResNet(nn.Module):
def __init__(self, block, num_blocks, num_classes=10):
super(ResNet, self).__init__()
self.in_planes = 16
self.conv1 = nn.Conv2d(3, 16, kernel_size=3, stride=1, padding=1, bias=False)
self.bn1 = nn.BatchNorm2d(16)
self.layer1 = self._make_layer(block, 16, num_blocks[0], stride=1)
self.layer2 = self._make_layer(block, 32, num_blocks[1], stride=2)
self.layer3 = self._make_layer(block, 64, num_blocks[2], stride=2)
self.linear = nn.Linear(64*block.expansion, num_classes)
def _make_layer(self, block, planes, num_blocks, stride):
strides = [stride] + [1]*(num_blocks-1)
layers = []
for stride in strides:
layers.append(block(self.in_planes, planes, stride))
self.in_planes = planes * block.expansion
return nn.Sequential(*layers)
def forward(self, x):
out = nn.ReLU()(self.bn1(self.conv1(x)))
out = self.layer1(out)
out = self.layer2(out)
out = self.layer3(out)
out = nn.AvgPool2d(8)(out)
out = out.view(out.size(0), -1)
out = self.linear(out)
return out
def ResNet20():
return ResNet(BasicBlock, [3,3,3])
```
这是一个基于PyTorch实现的ResNet20网络结构。
使用Resnet 101 网络结构替换 Resnet 50 网络结构
可以使用Resnet 101网络结构替换Resnet 50网络结构,这样可以增加网络深度并且提升模型的性能。Resnet 101相比于Resnet 50,增加了更多的卷积层和残差块,可以更好地提取高级特征,提高模型的准确率。当然,使用更深的网络结构也会增加计算和存储开销,需要根据具体场景和硬件条件进行选择。
阅读全文
相关推荐
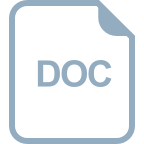
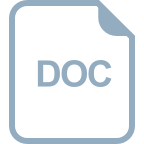
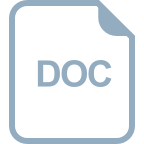













