翻译一下To validate the effectiveness of modality-aware representation learning (MARL) and adaptive graph learning (AGL), we replace MARL with MLP and direct concatenation respectively, and replace AGL with the construction method adopted in popGCN [11] and kNN graph GkNN using RBF kernel. Table IV shows the ablation study results on applying different modules in our models. Specifically, the performance of the constructed graph GP opGCN of popGCN is the worst, especially on the ABIDE dataset, indicating that handconstructed graph is indeed not a desirable choice. Furthermore, both “MARL+GpopGCN ” and “MARL+GkNN ” worsen the performance of MARL, which means that an inappropriate metric can have negative impacts. Besides, AGL achieves a favorable performance despite the absence of MARL, which again validates the effectiveness of adaptive graph learning. More importantly, it can be observed that the combination of MARL and AGL achieves a performance that far exceeds the other combinations. In addition, we also compare the performance of AGL and transformer [35], which is a popular architecture, as shown in Table V. IPT is designed as an interpatient transformer into which the patients’ representations are fed to obtain the results. It can be seen that the performance of the two methods is very close to each other.
时间: 2023-06-10 10:04:45 浏览: 196
为了验证模态感知表示学习(MARL)和自适应图学习(AGL)的有效性,我们分别使用MLP和直接串联来代替MARL,使用popGCN的构建方法和使用RBF内核的kNN图GkNN来代替AGL,并在我们的模型中应用不同的模块,表IV显示了消融研究结果。具体来说,popGCN的构建图GP opGCN的性能最差,特别是在ABIDE数据集上,表明手工构建的图确实不是一个理想的选择。此外,“MARL GpopGCN”和“MARL GkNN”都会降低MARL的性能,这意味着不合适的度量标准会产生负面影响。此外,尽管MARL不在场,AGL仍然取得了一个良好的表现,这再次验证了自适应图学习的有效性。更重要的是,可以观察到MARL和AGL的组合实现了远远超过其他组合的性能。此外,我们还比较了AGL和Transformer[35]的性能,Transformer是一种流行的体系结构,如表V所示。IPT被设计为一种患者间的Transformer,将患者的表示馈入以获得结果。可以看出,这两种方法的性能非常接近。
阅读全文
相关推荐
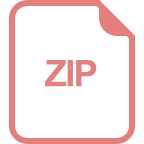
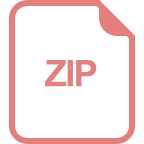
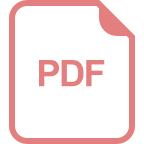
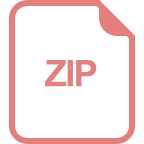
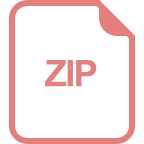
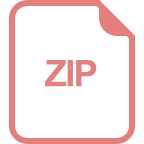
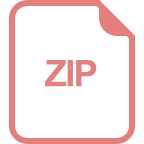
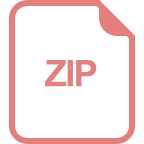
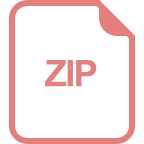
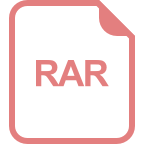
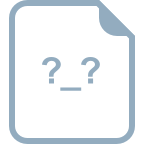
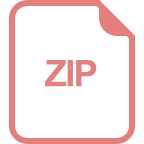
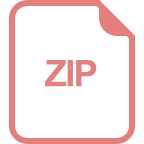
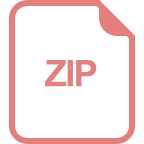
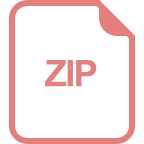
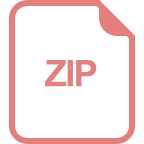
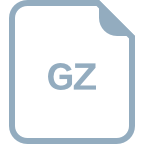
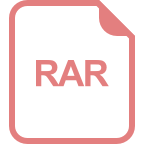