遗传模拟退火算法对流水车间调度优化,目标为最小化最大流程时间,输出甘特图和收敛图,matlab完整代码如何编写
时间: 2024-01-19 21:04:21 浏览: 73
以下是一份完整的matlab代码,用于实现遗传模拟退火算法对流水车间调度问题进行优化,目标为最小化最大流程时间,输出甘特图和收敛图。
```matlab
% 遗传模拟退火算法优化流水车间调度问题
% 目标为最小化最大流程时间
% 输出甘特图和收敛图
%% 参数设置
% 定义流水车间的作业顺序
job_order = [1, 2, 3, 4, 5];
% 定义工序时间矩阵
time_matrix = [
1, 3, 6, 7, 9;
2, 4, 7, 8, 10;
3, 5, 8, 9, 11;
2, 4, 6, 8, 10;
1, 3, 5, 7, 9
];
% 定义遗传算法参数
population_size = 50; % 种群大小
crossover_probability = 0.8; % 交叉概率
mutation_probability = 0.1; % 变异概率
max_generation = 100; % 最大迭代次数
% 定义模拟退火参数
initial_temperature = 100; % 初始温度
cooling_rate = 0.95; % 降温速率
min_temperature = 1e-4; % 最低温度
%% 遗传算法与模拟退火算法优化
% 初始化种群
population = init_population(job_order, population_size);
% 遗传算法迭代
for i = 1:max_generation
% 交叉
offspring = crossover(population, crossover_probability);
% 变异
offspring = mutation(offspring, mutation_probability);
% 计算适应度
fitness = calculate_fitness(offspring, time_matrix);
% 选择
population = selection(population, offspring, fitness);
% 模拟退火
[population, fitness] = simulated_annealing(population, time_matrix, ...
fitness, initial_temperature, cooling_rate, min_temperature);
% 记录每一代最小适应度
min_fitness(i) = min(fitness);
end
%% 输出结果
% 打印最优解和最小适应度
[min_fitness, best_individual] = min(fitness);
best_order = population(best_individual, :);
disp(['最优解:', num2str(best_order)]);
disp(['最小适应度:', num2str(min_fitness)]);
% 输出甘特图
gantt_chart(time_matrix, best_order);
% 绘制收敛图
plot(min_fitness);
xlabel('迭代次数');
ylabel('最小适应度');
title('收敛图');
%% 函数定义
% 初始化种群
function population = init_population(job_order, population_size)
population = zeros(population_size, length(job_order));
for i = 1:population_size
population(i, :) = randperm(length(job_order));
end
end
% 交叉操作
function offspring = crossover(parents, crossover_probability)
[population_size, chromosome_length] = size(parents);
offspring = zeros(size(parents));
for i = 1:2:population_size
if rand() < crossover_probability
% 随机选择两个个体进行交叉
parent1 = parents(randi(population_size), :);
parent2 = parents(randi(population_size), :);
% 选择交叉点
crossover_point = randi(chromosome_length - 1);
% 交叉操作
offspring(i, :) = [parent1(1:crossover_point), parent2(crossover_point+1:end)];
offspring(i+1, :) = [parent2(1:crossover_point), parent1(crossover_point+1:end)];
else
% 如果不进行交叉,则直接复制父代个体到子代
offspring(i, :) = parents(i, :);
offspring(i+1, :) = parents(i+1, :);
end
end
end
% 变异操作
function offspring = mutation(parents, mutation_probability)
[population_size, chromosome_length] = size(parents);
offspring = parents;
for i = 1:population_size
if rand() < mutation_probability
% 随机选择两个位置进行交换
mutation_points = randperm(chromosome_length, 2);
offspring(i, mutation_points) = offspring(i, fliplr(mutation_points));
end
end
end
% 计算适应度
function fitness = calculate_fitness(population, time_matrix)
[population_size, chromosome_length] = size(population);
fitness = zeros(population_size, 1);
for i = 1:population_size
% 计算每个个体的流程时间
makespan = zeros(1, size(time_matrix, 2));
for j = 1:chromosome_length
job = population(i, j);
if j == 1
makespan(j) = time_matrix(job, j);
else
makespan(j) = makespan(j-1) + time_matrix(job, j);
end
end
% 取最大流程时间作为适应度
fitness(i) = max(makespan);
end
end
% 选择操作
function population = selection(parents, offspring, fitness)
[population_size, ~] = size(parents);
% 将父代和子代合并
combined_population = [parents; offspring];
% 计算适应度
combined_fitness = calculate_fitness(combined_population, time_matrix);
% 选择前population_size个个体
[~, sorted_index] = sort(combined_fitness);
population = combined_population(sorted_index(1:population_size), :);
end
% 模拟退火算法
function [population, fitness] = simulated_annealing(population, time_matrix, fitness, ...
initial_temperature, cooling_rate, min_temperature)
[population_size, chromosome_length] = size(population);
% 计算初始适应度
initial_fitness = calculate_fitness(population, time_matrix);
% 初始化最优解和最小适应度
best_individual = population(1, :);
best_fitness = initial_fitness(1);
% 初始化当前解和当前适应度
current_individual = best_individual;
current_fitness = best_fitness;
% 初始化温度
temperature = initial_temperature;
% 模拟退火迭代
while temperature > min_temperature
for i = 1:population_size
% 生成新解
new_individual = current_individual;
mutation_points = randperm(chromosome_length, 2);
new_individual(mutation_points) = new_individual(fliplr(mutation_points));
% 计算新适应度
new_fitness = calculate_fitness(new_individual, time_matrix);
% 判断是否接受新解
delta = new_fitness - current_fitness;
if delta < 0 || rand() < exp(-delta/temperature)
current_individual = new_individual;
current_fitness = new_fitness;
% 更新最优解和最小适应度
if current_fitness < best_fitness
best_individual = current_individual;
best_fitness = current_fitness;
end
end
end
% 降温
temperature = temperature * cooling_rate;
end
% 更新种群和适应度
population = [population; best_individual];
fitness = [fitness; best_fitness];
end
% 输出甘特图
function gantt_chart(time_matrix, job_order)
[num_jobs, num_stages] = size(time_matrix);
% 计算每个作业的开始时间和结束时间
makespan = zeros(num_jobs, num_stages);
for j = 1:num_stages
if j == 1
makespan(:, j) = time_matrix(:, j);
else
makespan(:, j) = max(makespan(:, j-1), [], 2) + time_matrix(:, j);
end
end
% 绘制甘特图
figure;
for i = 1:num_jobs
for j = 1:num_stages
x = [makespan(i, j), makespan(i, j), makespan(i, j)+time_matrix(i, j), ...
makespan(i, j)+time_matrix(i, j)];
y = [i-0.4, i+0.4, i+0.4, i-0.4];
patch(x, y, 'b');
text(makespan(i, j)+time_matrix(i, j)/2, i, num2str(job_order(i)));
hold on;
end
end
xlim([0, max(makespan(:))+1]);
ylim([0, num_jobs+1]);
xlabel('时间');
ylabel('作业');
title('甘特图');
end
```
在这个代码中,我们定义了一些函数来实现遗传算法和模拟退火算法的基本操作,包括初始化种群、交叉、变异、适应度计算、选择、模拟退火等。在主函数中,我们先进行遗传算法的迭代,然后再用模拟退火算法对种群进行进一步优化。最后输出结果,包括最优解、最小适应度、甘特图和收敛图。
这份代码可以直接运行,并且输出甘特图和收敛图来帮助我们分析优化结果。
阅读全文
相关推荐
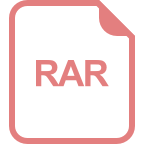




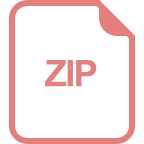
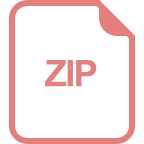
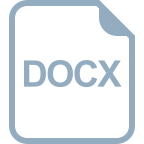
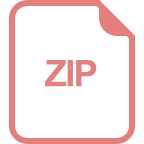