Attention()怎么用
时间: 2024-06-08 19:05:42 浏览: 16
Attention() 是一种用于机器学习模型中的一种注意力机制,通常用于处理输入序列中不同位置的信息。
下面是一个简单的示例,展示了如何使用 Attention() 函数:
```python
import tensorflow as tf
from tensorflow.keras.layers import Input, Dense, LSTM, Attention
from tensorflow.keras.models import Model
# 定义输入序列的长度和特征维度
sequence_length = 10
feature_dim = 32
# 定义模型的输入层
input_layer = Input(shape=(sequence_length, feature_dim))
# 定义 LSTM 层,作为编码器
encoder_output, state_h, state_c = LSTM(64, return_sequences=True, return_state=True)(input_layer)
# 定义 Attention 层,作为解码器
attention_output = Attention()([encoder_output, encoder_output])
# 定义输出层
output_layer = Dense(1, activation='sigmoid')(attention_output)
# 定义整个模型
model = Model(inputs=input_layer, outputs=output_layer)
# 编译模型
model.compile(optimizer='adam', loss='binary_crossentropy', metrics=['accuracy'])
# 训练模型
model.fit(x_train, y_train, batch_size=32, epochs=10, validation_data=(x_test, y_test))
```
在上面的示例中,我们首先定义了输入序列的长度和特征维度。然后,我们定义了一个 LSTM 层作为编码器,并将其输出作为 Attention 层的输入。最后,我们定义了一个输出层,使用 Attention 层的输出作为输入。
要使用 Attention() 函数,我们只需要将编码器的输出作为第一个参数传递给 Attention() 函数,然后再将 Attention 层的输出作为解码器的输入即可。在这个例子中,我们使用的是默认的 Attention() 函数,它执行 dot product attention,即对编码器的输出进行点乘操作,然后对结果进行 softmax 归一化,得到解码器的输入。
值得注意的是,Attention() 函数的参数不止一个,还可以设置其他的参数,例如 attention_type、use_scale 等,这些参数可以控制 Attention() 函数的行为。
相关推荐
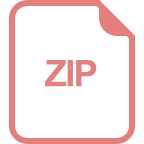
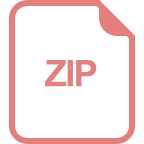
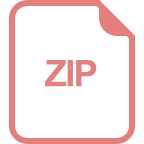
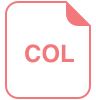
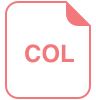
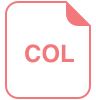
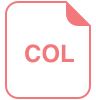
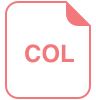









