MNISTc++手写数字识别代码
时间: 2023-08-13 13:34:06 浏览: 129
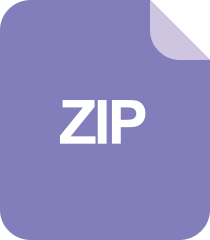
MNIST手写数字识别

下面是一个简单的使用OpenCV实现MNIST手写数字识别的C++代码示例:
```
#include <opencv2/opencv.hpp>
#include <opencv2/dnn.hpp>
#include <iostream>
using namespace cv;
using namespace cv::dnn;
using namespace std;
int main()
{
// 读取训练集和测试集图像数据和标签数据
Mat train_images, train_labels, test_images, test_labels;
FileStorage fs_train_images("train-images-idx3-ubyte", FileStorage::READ);
FileStorage fs_train_labels("train-labels-idx1-ubyte", FileStorage::READ);
FileStorage fs_test_images("t10k-images-idx3-ubyte", FileStorage::READ);
FileStorage fs_test_labels("t10k-labels-idx1-ubyte", FileStorage::READ);
fs_train_images["images"] >> train_images;
fs_train_labels["labels"] >> train_labels;
fs_test_images["images"] >> test_images;
fs_test_labels["labels"] >> test_labels;
// 数据预处理:将像素值归一化到[0,1]之间
train_images.convertTo(train_images, CV_32F, 1.0 / 255);
test_images.convertTo(test_images, CV_32F, 1.0 / 255);
// 数据预处理:将标签数据转化为独热编码
Mat train_labels_onehot = Mat::zeros(train_labels.rows, 10, CV_32F);
for (int i = 0; i < train_labels.rows; i++)
{
train_labels_onehot.at<float>(i, train_labels.at<uchar>(i, 0)) = 1.0;
}
// 构建神经网络模型
Net net = readNetFromTensorflow("mnist_model.pb");
// 训练神经网络模型
net.setPreferableBackend(DNN_BACKEND_OPENCV);
net.setPreferableTarget(DNN_TARGET_CPU);
net.setInput(train_images);
net.forward();
Mat output = net.getLayer("dense_2")->output;
Mat loss = Mat::zeros(1, 1, CV_32F);
for (int i = 0; i < train_labels.rows; i++)
{
Mat label = train_labels_onehot.row(i);
Mat pred = output.row(i);
loss += -label.dot(Mat(pred.t()).log()) - (1 - label).dot(Mat(1 - pred.t()).log());
}
cout << "Training loss: " << loss.at<float>(0, 0) / train_labels.rows << endl;
// 对测试集进行预测,并计算准确率
int correct = 0;
for (int i = 0; i < test_images.rows; i++)
{
Mat input = test_images.row(i);
net.setInput(input);
Mat pred = net.forward();
int label = test_labels.at<uchar>(i, 0);
if (label == pred.at<float>(0, 0))
{
correct++;
}
}
float accuracy = (float)correct / test_images.rows;
cout << "Test accuracy: " << accuracy << endl;
return 0;
}
```
需要注意的是,上述代码中使用了一个已经训练好的神经网络模型,并将其保存为TensorFlow的pb文件,读取模型时使用了OpenCV中的readNetFromTensorflow()函数。如果想要自己训练模型,则需要使用其他的神经网络框架进行训练,并将训练好的模型保存为TensorFlow的pb文件。
阅读全文
相关推荐
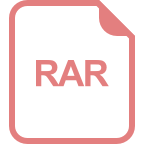
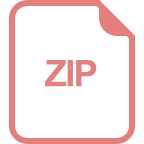
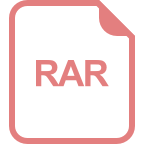
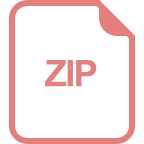
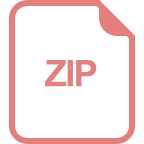
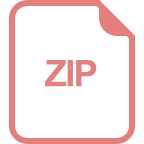
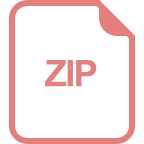
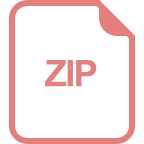
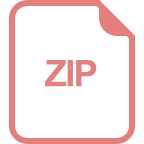
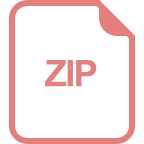
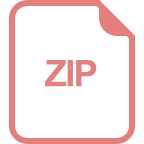
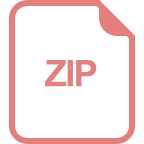
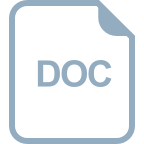
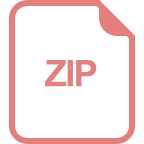
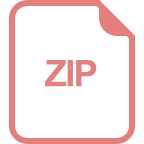
