基于sift的python-opencv多幅影像拼接
时间: 2023-09-12 12:10:20 浏览: 126
实现多幅影像拼接的步骤如下:
1. 读取所有待拼接的影像并转换为灰度图像;
2. 使用 SIFT 算法提取关键点和描述子;
3. 使用 FLANN 或 BFMatcher 算法匹配关键点;
4. 根据匹配得到的点对计算单应性矩阵;
5. 使用单应性矩阵将影像拼接起来。
下面是基于 Python-OpenCV 实现多幅影像拼接的示例代码:
```python
import cv2
import numpy as np
# 读取待拼接的影像
img1 = cv2.imread('image1.png')
img2 = cv2.imread('image2.png')
img3 = cv2.imread('image3.png')
# 转换为灰度图像
gray1 = cv2.cvtColor(img1, cv2.COLOR_BGR2GRAY)
gray2 = cv2.cvtColor(img2, cv2.COLOR_BGR2GRAY)
gray3 = cv2.cvtColor(img3, cv2.COLOR_BGR2GRAY)
# 创建 SIFT 对象
sift = cv2.xfeatures2d.SIFT_create()
# 提取关键点和描述子
kp1, des1 = sift.detectAndCompute(gray1, None)
kp2, des2 = sift.detectAndCompute(gray2, None)
kp3, des3 = sift.detectAndCompute(gray3, None)
# 创建 FLANN 匹配器
FLANN_INDEX_KDTREE = 0
index_params = dict(algorithm=FLANN_INDEX_KDTREE, trees=5)
search_params = dict(checks=50)
flann = cv2.FlannBasedMatcher(index_params, search_params)
# 匹配关键点
matches1_2 = flann.knnMatch(des1, des2, k=2)
matches2_3 = flann.knnMatch(des2, des3, k=2)
# 根据 Lowe's ratio test 筛选匹配点
good_matches1_2 = []
for m, n in matches1_2:
if m.distance < 0.7 * n.distance:
good_matches1_2.append(m)
good_matches2_3 = []
for m, n in matches2_3:
if m.distance < 0.7 * n.distance:
good_matches2_3.append(m)
# 提取匹配点对的坐标
src_pts1_2 = np.float32([kp1[m.queryIdx].pt for m in good_matches1_2]).reshape(-1, 1, 2)
dst_pts1_2 = np.float32([kp2[m.trainIdx].pt for m in good_matches1_2]).reshape(-1, 1, 2)
src_pts2_3 = np.float32([kp2[m.queryIdx].pt for m in good_matches2_3]).reshape(-1, 1, 2)
dst_pts2_3 = np.float32([kp3[m.trainIdx].pt for m in good_matches2_3]).reshape(-1, 1, 2)
# 计算单应性矩阵
H1_2, _ = cv2.findHomography(src_pts1_2, dst_pts1_2, cv2.RANSAC, 5.0)
H2_3, _ = cv2.findHomography(src_pts2_3, dst_pts2_3, cv2.RANSAC, 5.0)
# 拼接影像
h1, w1 = img1.shape[:2]
h2, w2 = img2.shape[:2]
h3, w3 = img3.shape[:2]
# 计算拼接后的影像大小
max_w = max(w1 + w2, w2 + w3)
max_h = max(h1, h2, h3)
# 创建空白影像
result = np.zeros((max_h, max_w, 3), dtype=np.uint8)
# 将第一幅影像拼接到空白影像上
result[:h1, :w1] = img1
# 将第二幅影像拼接到空白影像上
tmp_img = cv2.warpPerspective(img2, H1_2, (max_w, max_h))
result[:h2, w1:w1+w2] = tmp_img[:, w1:w1+w2]
# 将第三幅影像拼接到空白影像上
tmp_img = cv2.warpPerspective(img3, H1_2.dot(H2_3), (max_w, max_h))
result[:h3, w1+w2:max_w] = tmp_img[:, w2:w3]
# 显示拼接后的影像
cv2.imshow('result', result)
cv2.waitKey()
cv2.destroyAllWindows()
```
注意,这里使用了 Lowe's ratio test 进行匹配点筛选,并使用 RANSAC 算法计算单应性矩阵。实际应用中,根据不同的场景和数据,可能需要调整这些参数。
相关推荐
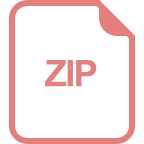
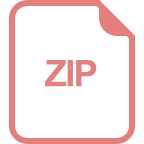
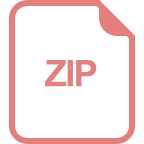














