利用Python写零样本学习的图像处理代码
时间: 2024-03-06 09:20:53 浏览: 20
由于零样本学习的目标是从未见过的类别中进行分类,因此需要使用一些预训练的模型来提取图像的特征。在这里,我们将使用预训练的ResNet50模型来提取特征,并使用线性支持向量机(Linear SVM)进行分类。
首先,我们需要导入必要的库和模块:
```python
import numpy as np
import pandas as pd
import matplotlib.pyplot as plt
import seaborn as sns
import os
import cv2
import urllib
import random
from sklearn.model_selection import train_test_split
from sklearn.svm import LinearSVC
from sklearn.metrics import accuracy_score
from keras.applications.resnet50 import ResNet50, preprocess_input
from keras.preprocessing.image import load_img, img_to_array
```
接下来,我们需要下载并加载预训练的ResNet50模型:
```python
model = ResNet50(weights='imagenet', include_top=False, input_shape=(224, 224, 3))
```
然后,我们需要定义一个函数来提取图像的特征:
```python
def extract_features(img_path, model):
img = load_img(img_path, target_size=(224, 224))
img_array = img_to_array(img)
img_array = np.expand_dims(img_array, axis=0)
img_array = preprocess_input(img_array)
features = model.predict(img_array)
return features.flatten()
```
该函数接受图像的路径和预训练的模型作为输入,并返回一个展平的特征向量。
接下来,我们需要定义一个函数来加载数据集和标签,并将其分为训练和测试集:
```python
def load_data():
data_dir = 'data/'
classes = ['cat', 'dog', 'bird', 'fish', 'rabbit', 'squirrel']
images = []
labels = []
for class_id, class_name in enumerate(classes):
class_dir = os.path.join(data_dir, class_name)
for img_name in os.listdir(class_dir):
img_path = os.path.join(class_dir, img_name)
images.append(img_path)
labels.append(class_id)
x_train, x_test, y_train, y_test = train_test_split(images, labels, test_size=0.2, random_state=42)
return x_train, x_test, y_train, y_test
```
该函数遍历数据集目录中的所有图像,并将它们的路径和对应的标签加载到两个列表中。然后,它使用train_test_split函数将数据集分为训练和测试集。
接下来,我们需要定义一个函数来训练线性支持向量机模型:
```python
def train_model(x_train, y_train):
x_train_features = [extract_features(img_path, model) for img_path in x_train]
svm = LinearSVC(random_state=42, max_iter=10000)
svm.fit(x_train_features, y_train)
return svm
```
该函数使用extract_features函数提取训练集中所有图像的特征,并使用LinearSVC函数训练一个分类器。
最后,我们需要定义一个函数来测试模型的性能:
```python
def test_model(model, x_test, y_test):
x_test_features = [extract_features(img_path, model) for img_path in x_test]
y_pred = model.predict(x_test_features)
accuracy = accuracy_score(y_test, y_pred)
return accuracy
```
该函数使用extract_features函数提取测试集中所有图像的特征,并使用predict函数预测它们的标签。然后,它使用accuracy_score函数计算模型的准确性。
现在,我们可以调用这些函数来训练和测试模型:
```python
x_train, x_test, y_train, y_test = load_data()
svm_model = train_model(x_train, y_train)
accuracy = test_model(svm_model, x_test, y_test)
print("Accuracy:", accuracy)
```
这将加载数据集,训练模型,并输出测试集上的准确性。
相关推荐
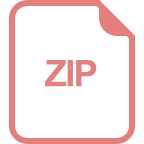
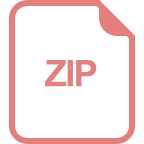
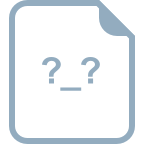
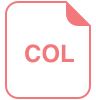
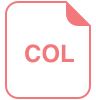
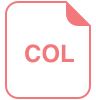











