讲讲 faster rcnn pytorch版本的代码
时间: 2023-03-19 07:23:59 浏览: 184
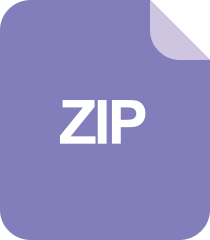
Faster RCNN pytroch代码
Faster R-CNN 是一种常用的目标检测算法,其 PyTorch 版本的实现可以参考以下代码:
1. 首先,需要导入所需的包和库:
```
import torch
import torch.nn as nn
import torch.nn.functional as F
from torchvision.models import vgg16
from torch.autograd import Variable
from torchvision.ops import RoIAlign
```
2. 定义 Faster R-CNN 模型,包括 RPN(区域生成网络)和 RoI pooling 层:
```
class FasterRCNN(nn.Module):
def __init__(self, n_class=21):
super(FasterRCNN, self).__init__()
# 加载预训练的 VGG16 模型
self.features = vgg16().features
self.rpn = RegionProposalNetwork(512, 512)
self.head = VGG16RoIHead(
n_class=n_class,
roi_size=7,
spatial_scale=(1. / 16),
classifier=self.classifier
)
def forward(self, x, scale=1.):
img_size = x.shape[2:]
h = self.features(x)
rpn_locs, rpn_scores, rois, roi_indices, anchor = self.rpn(h, img_size, scale)
roi_cls_locs, roi_scores = self.head(h, rois, roi_indices)
return roi_cls_locs, roi_scores, rois, roi_indices
```
3. 定义 RPN 层:
```
class RegionProposalNetwork(nn.Module):
def __init__(self, in_channels=512, mid_channels=512, ratios=[0.5, 1, 2], anchor_scales=[8, 16, 32]):
super(RegionProposalNetwork, self).__init__()
self.anchor_base = generate_anchor_base(anchor_scales=anchor_scales, ratios=ratios)
n_anchor = self.anchor_base.shape[0]
self.conv1 = nn.Conv2d(in_channels, mid_channels, 3, 1, 1)
self.score = nn.Conv2d(mid_channels, n_anchor * 2, 1, 1, 0)
self.loc = nn.Conv2d(mid_channels, n_anchor * 4, 1, 1, 0)
self.anchor = self.anchor_base.reshape((1, n_anchor, 4))
self.proposal_layer = ProposalCreator(self)
normal_init(self.conv1, 0, 0.01)
normal_init(self.score, 0, 0.01)
normal_init(self.loc, 0, 0.01)
def forward(self, x, img_size, scale=1.):
n, _, hh, ww = x.shape
anchor = _enumerate_shifted_anchor(
np.array(self.anchor_base),
self.feat_stride, hh, ww
)
anchor = torch.from_numpy(anchor).to(device=x.device, dtype=x.dtype)
n_anchor = anchor.shape[0] // (hh * ww)
h = F.relu(self.conv1(x))
rpn_locs = self.loc(h)
rpn_scores = self.score(h)
rpn_locs = rpn_locs.permute(0, 2, 3, 1).reshape(n, -1, 4)
rpn_scores = rpn_scores.permute(0, 2, 3, 1).reshape(n, -1, 2)
anchor = anchor.reshape(-1, 4)
阅读全文
相关推荐
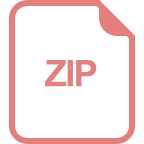





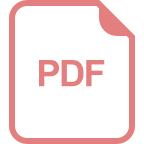
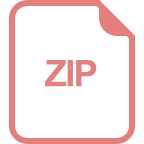
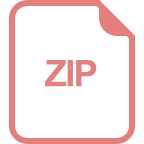
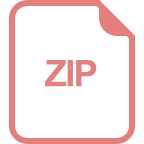
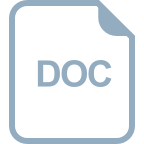



