unet加残差网络代码
时间: 2023-10-15 19:02:06 浏览: 67
以下是使用 PyTorch 实现的 U-Net 加残差网络的代码:
``` python
import torch
import torch.nn as nn
import torch.nn.functional as F
class DoubleConv(nn.Module):
"""
双卷积层
"""
def __init__(self, in_ch, out_ch):
super(DoubleConv, self).__init__()
self.conv = nn.Sequential(
nn.Conv2d(in_ch, out_ch, 3, padding=1),
nn.BatchNorm2d(out_ch),
nn.ReLU(inplace=True),
nn.Conv2d(out_ch, out_ch, 3, padding=1),
nn.BatchNorm2d(out_ch),
nn.ReLU(inplace=True)
)
def forward(self, x):
x = self.conv(x)
return x
class ResidualBlock(nn.Module):
"""
残差块
"""
def __init__(self, in_ch, out_ch):
super(ResidualBlock, self).__init__()
self.conv = nn.Sequential(
nn.Conv2d(in_ch, out_ch, 3, padding=1),
nn.BatchNorm2d(out_ch),
nn.ReLU(inplace=True),
nn.Conv2d(out_ch, out_ch, 3, padding=1),
nn.BatchNorm2d(out_ch)
)
if in_ch != out_ch:
self.downsample = nn.Sequential(
nn.Conv2d(in_ch, out_ch, 1, stride=1),
nn.BatchNorm2d(out_ch)
)
else:
self.downsample = None
self.relu = nn.ReLU(inplace=True)
def forward(self, x):
identity = x
out = self.conv(x)
if self.downsample is not None:
identity = self.downsample(x)
out += identity
out = self.relu(out)
return out
class UNetResNet(nn.Module):
"""
U-Net 加残差网络
"""
def __init__(self, n_channels, n_classes):
super(UNetResNet, self).__init__()
self.n_channels = n_channels
self.n_classes = n_classes
# 下采样部分
self.conv1 = DoubleConv(n_channels, 64)
self.pool1 = nn.MaxPool2d(2)
self.conv2 = DoubleConv(64, 128)
self.pool2 = nn.MaxPool2d(2)
self.conv3 = DoubleConv(128, 256)
self.pool3 = nn.MaxPool2d(2)
self.conv4 = DoubleConv(256, 512)
self.pool4 = nn.MaxPool2d(2)
# 中间部分
self.conv5 = DoubleConv(512, 1024)
self.res1 = ResidualBlock(1024, 1024)
self.res2 = ResidualBlock(1024, 1024)
self.res3 = ResidualBlock(1024, 1024)
# 上采样部分
self.up6 = nn.ConvTranspose2d(1024, 512, 2, stride=2)
self.conv6 = DoubleConv(1024, 512)
self.up7 = nn.ConvTranspose2d(512, 256, 2, stride=2)
self.conv7 = DoubleConv(512, 256)
self.up8 = nn.ConvTranspose2d(256, 128, 2, stride=2)
self.conv8 = DoubleConv(256, 128)
self.up9 = nn.ConvTranspose2d(128, 64, 2, stride=2)
self.conv9 = DoubleConv(128, 64)
# 输出部分
self.outconv = nn.Conv2d(64, n_classes, 1)
def forward(self, x):
# 下采样
c1 = self.conv1(x)
p1 = self.pool1(c1)
c2 = self.conv2(p1)
p2 = self.pool2(c2)
c3 = self.conv3(p2)
p3 = self.pool3(c3)
c4 = self.conv4(p3)
p4 = self.pool4(c4)
# 中间部分
c5 = self.conv5(p4)
res1 = self.res1(c5)
res2 = self.res2(res1)
res3 = self.res3(res2)
# 上采样
up_6 = self.up6(res3)
merge6 = torch.cat([up_6, c4], dim=1)
c6 = self.conv6(merge6)
up_7 = self.up7(c6)
merge7 = torch.cat([up_7, c3], dim=1)
c7 = self.conv7(merge7)
up_8 = self.up8(c7)
merge8 = torch.cat([up_8, c2], dim=1)
c8 = self.conv8(merge8)
up_9 = self.up9(c8)
merge9 = torch.cat([up_9, c1], dim=1)
c9 = self.conv9(merge9)
# 输出部分
out = self.outconv(c9)
return out
```
这里实现的 U-Net 加残差网络与标准的 U-Net 不同之处在于中间部分采用了残差块,从而加强了网络的深度和学习能力。同时,为了保持特征图大小的一致性,在上采样过程中使用了转置卷积层。
相关推荐
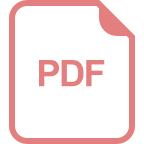
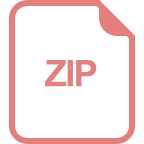
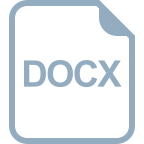














