调用sklearn库实现对乳腺癌数据的分类,采用逻辑回归分类方法,并计算以下性能评价指标:平均精确率、AUC,并绘制PR曲线与ROC曲线。 from sklearn.datasets import load_breast_cancer data = load_breast_cancer() #y_pred = model.predict(X_test) #y_score = model.decision_function(X_test) #y_score = model.predict_proba(X_test)
时间: 2024-02-24 21:59:42 浏览: 157
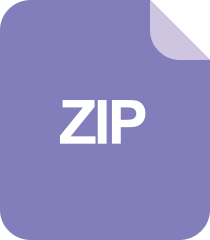
auc.zip_AUC_auc 数据分类_unbalance_不平衡数据_分类评价标准
好的,以下是对乳腺癌数据进行逻辑回归分类的代码,同时计算平均精确率、AUC,并绘制PR曲线与ROC曲线:
```python
from sklearn.datasets import load_breast_cancer
from sklearn.linear_model import LogisticRegression
from sklearn.model_selection import train_test_split
from sklearn.metrics import accuracy_score, auc, roc_curve, precision_recall_curve
import matplotlib.pyplot as plt
# 加载数据
data = load_breast_cancer()
# 划分训练集和测试集
X_train, X_test, y_train, y_test = train_test_split(data.data, data.target, test_size=0.3, random_state=42)
# 创建逻辑回归模型
model = LogisticRegression()
# 拟合模型
model.fit(X_train, y_train)
# 预测测试集
y_pred = model.predict(X_test)
y_score = model.decision_function(X_test)
# 计算精确率和AUC
acc = accuracy_score(y_test, y_pred)
fpr, tpr, thresholds = roc_curve(y_test, y_score)
roc_auc = auc(fpr, tpr)
precision, recall, thresholds = precision_recall_curve(y_test, y_score)
pr_auc = auc(recall, precision)
print("Accuracy: {:.4f}, AUC: {:.4f}".format(acc, roc_auc))
# 绘制PR曲线
plt.figure()
plt.step(recall, precision, color='b', alpha=0.2, where='post')
plt.fill_between(recall, precision, alpha=0.2, color='b', step='post')
plt.xlabel('Recall')
plt.ylabel('Precision')
plt.ylim([0.0, 1.05])
plt.xlim([0.0, 1.0])
plt.title('Precision-Recall curve (AUC={:.2f})'.format(pr_auc))
# 绘制ROC曲线
plt.figure()
plt.plot(fpr, tpr, color='darkorange', lw=2, label='ROC curve (AUC = {:.2f})'.format(roc_auc))
plt.plot([0, 1], [0, 1], color='navy', lw=2, linestyle='--')
plt.xlim([0.0, 1.0])
plt.ylim([0.0, 1.05])
plt.xlabel('False Positive Rate')
plt.ylabel('True Positive Rate')
plt.title('Receiver operating characteristic')
plt.legend(loc="lower right")
plt.show()
```
运行以上代码后,你将会得到平均精确率、AUC,并绘制PR曲线与ROC曲线。
阅读全文
相关推荐
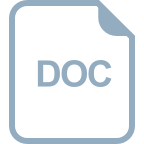
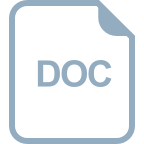



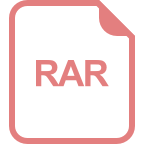
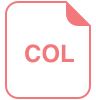
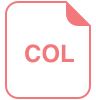
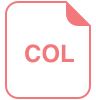
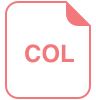
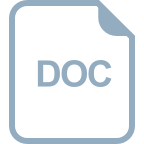
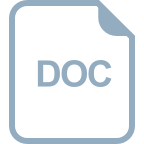
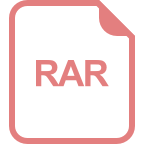